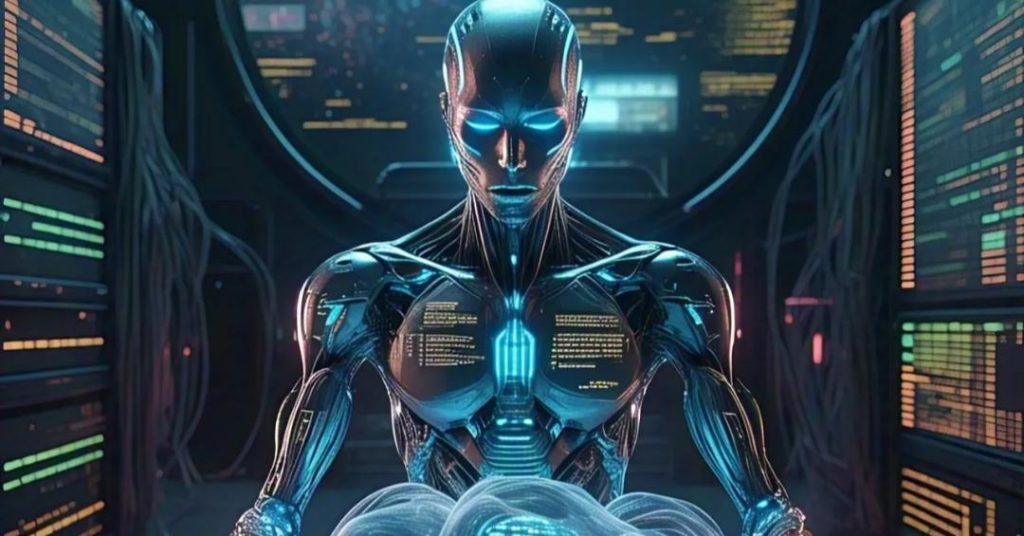
How Poor Data Management Can Put AI Projects at Risk
Artificial intelligence (AI) has been touted as the future of business, promising to revolutionize industries and boost productivity. However, a recent survey by Gartner has revealed a shocking truth: 63% of organizations lack AI-ready data, which puts their AI projects at risk of failure. This alarming statistic highlights the importance of proper data management in AI adoption. In this blog post, we will explore the consequences of poor data management and why it’s essential to bridge the gap between AI-ready requirements and traditional data management practices.
The Gartner survey, which polled 1,203 data management leaders in 2024, found that organizations failing to recognize the differences between AI-ready requirements and traditional data management practices are endangering the success of their AI efforts. The survey’s findings suggest that poor data management is a significant obstacle to AI adoption, and it’s crucial for organizations to address this issue to reap the benefits of AI.
So, what exactly is AI-ready data, and why is it so critical for AI projects? AI-ready data refers to high-quality, organized, and accessible data that is specifically designed to be used by AI systems. This type of data is essential for training AI models, which rely on large amounts of data to learn and improve over time. However, traditional data management practices often fall short of meeting the requirements of AI-ready data.
One of the main challenges in achieving AI-ready data is metadata management. Metadata refers to the data that describes other data, such as its origin, format, and quality. In traditional data management practices, metadata is often overlooked or undermanaged, which can lead to data inconsistencies, inaccuracies, and lack of transparency. AI systems, on the other hand, require high-quality metadata to understand the context and meaning of the data, making it a critical component of AI-ready data.
Another challenge in achieving AI-ready data is the lack of data standardization. AI systems require data to be standardized and formatted in a specific way to ensure consistency and accuracy. However, traditional data management practices often result in data being stored in various formats and structures, making it difficult to integrate and analyze. AI-ready data, on the other hand, requires data to be standardized and formatted in a way that is easily accessible and usable by AI systems.
The consequences of poor data management in AI projects are far-reaching and can lead to a range of negative outcomes, including:
- Delayed project timelines: Poor data management can lead to delays in AI project timelines, as data quality and integrity issues need to be addressed before AI models can be trained and deployed.
- Inaccurate results: AI systems rely on high-quality data to produce accurate results. Poor data management can lead to inaccurate results, which can have serious consequences in industries such as healthcare and finance.
- Inefficient use of resources: Poor data management can lead to inefficient use of resources, as data quality and integrity issues need to be addressed manually, which can be time-consuming and costly.
- Lack of trust: Poor data management can lead to a lack of trust in AI systems, as users may question the accuracy and reliability of the results produced by the systems.
To avoid these consequences, organizations need to prioritize data management and ensure that their AI projects are based on high-quality, AI-ready data. This requires a shift in mindset, from traditional data management practices to a more proactive and strategic approach to data management.
So, what can organizations do to ensure that their AI projects are based on high-quality, AI-ready data? Here are some best practices:
- Prioritize metadata management: Organizations should prioritize metadata management and ensure that metadata is accurate, complete, and up-to-date.
- Standardize data: Organizations should standardize data and ensure that it is formatted in a consistent way to ensure consistency and accuracy.
- Use data catalogs: Organizations should use data catalogs to manage and govern data, ensuring that data is accessible and usable by AI systems.
- Invest in data quality tools: Organizations should invest in data quality tools to ensure that data is accurate, complete, and consistent.
- Develop a data strategy: Organizations should develop a data strategy that aligns with their AI goals and objectives, and ensures that data is managed and governed in a way that supports AI adoption.
In conclusion, poor data management is a significant obstacle to AI adoption, and it’s essential for organizations to prioritize data management and ensure that their AI projects are based on high-quality, AI-ready data. By prioritizing metadata management, standardizing data, using data catalogs, investing in data quality tools, and developing a data strategy, organizations can avoid the consequences of poor data management and achieve the benefits of AI.