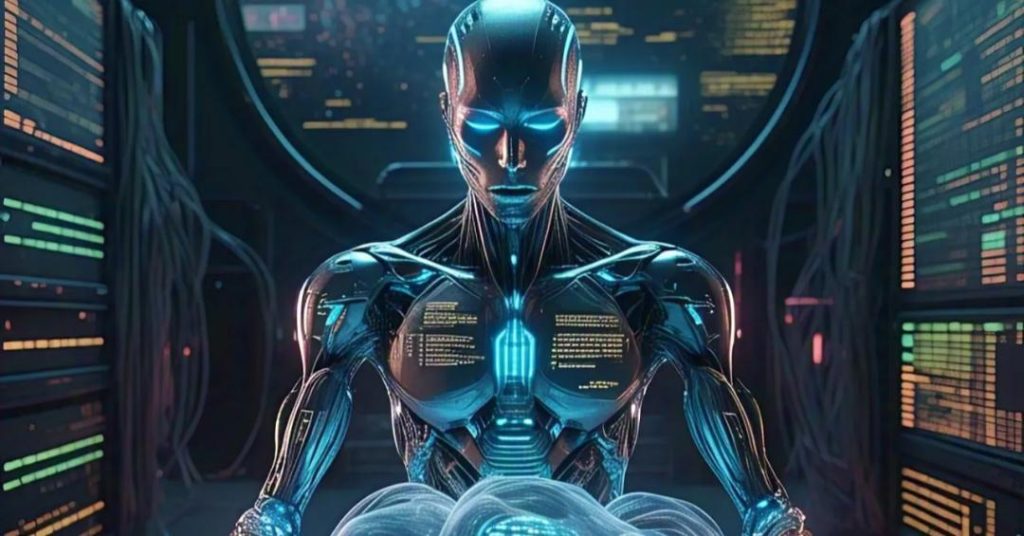
How Poor Data Management Can Put AI Projects at Risk
The increasing adoption of Artificial Intelligence (AI) in various industries has led to a surge in the need for quality data to fuel these projects. However, a recent survey by Gartner revealed a startling statistic – 63% of organizations lack AI-ready data, putting their AI projects at risk of failure. This alarming figure highlights the importance of effective data management in AI adoption.
In a survey of 1,203 data management leaders in 2024, Gartner found that many organizations are yet to understand the fundamental differences between AI-ready data requirements and traditional data management practices. This lack of understanding is a significant obstacle to the success of AI projects.
So, what exactly is AI-ready data, and why is poor data management putting AI projects at risk? In this blog post, we’ll delve into the world of AI data management and explore the consequences of poor data management practices.
What is AI-Ready Data?
AI-ready data refers to high-quality data that is structured, well-organized, and easily accessible. This type of data is essential for AI model training, testing, and deployment. AI-ready data ensures that AI models are trained on accurate and relevant information, which in turn enables them to make informed decisions and provide accurate predictions.
However, many organizations struggle to provide AI-ready data due to various reasons such as:
- Data quality issues: Poor data quality can lead to inaccurate predictions, biased models, and reduced model performance. Inaccurate data can also lead to unnecessary processing power, storage, and computational resources.
- Data scarcity: Many organizations lack the necessary data to train AI models. This scarcity can hinder the development of AI applications and limit their potential.
- Data silos: Data silos refer to the isolation of data across different departments or systems. This isolation can lead to data duplication, inconsistencies, and reduced data quality.
Consequences of Poor Data Management
Poor data management practices can have severe consequences on AI projects. Some of the consequences include:
- Delayed project timelines: Poor data management can lead to delays in AI project timelines. Inaccurate or incomplete data can cause AI models to fail, requiring significant rework and delaying project completion.
- Increased costs: Poor data management can lead to increased costs due to the need for additional data cleaning, processing, and rework. This can also lead to wasted resources and unnecessary expenses.
- Reduced model accuracy: Poor data management can reduce the accuracy of AI models. Inaccurate data can lead to biased models, reduced performance, and decreased confidence in AI applications.
- Compliance and regulatory issues: Poor data management can lead to compliance and regulatory issues. Inaccurate or incomplete data can lead to non-compliance with data regulations and increased risk of fines and penalties.
Metadata Management – The Unsung Hero of AI Data Management
Metadata management is often overlooked in discussions about AI data management. However, metadata is essential for AI-ready data. Metadata refers to the data that describes other data, such as data quality, data lineage, and data provenance. Effective metadata management ensures that AI models are trained on high-quality data and reduces the risk of data errors and inconsistencies.
Some of the benefits of metadata management include:
- Data discovery: Metadata management enables data discovery, making it easier to find and access relevant data for AI model training.
- Data quality: Metadata management ensures that data is accurate, complete, and consistent, reducing the risk of data errors and inconsistencies.
- Data governance: Metadata management enables data governance, ensuring that data is managed in accordance with organizational policies and regulations.
Best Practices for AI Data Management
To avoid the pitfalls of poor data management and ensure the success of AI projects, organizations should adopt the following best practices:
- Develop a data strategy: Develop a data strategy that outlines the organization’s data management goals, objectives, and policies.
- Invest in metadata management: Invest in metadata management tools and technology to ensure that data is accurately described and easily accessible.
- Implement data quality controls: Implement data quality controls to ensure that data is accurate, complete, and consistent.
- Train data management leaders: Train data management leaders to understand the differences between AI-ready data requirements and traditional data management practices.
- Monitor and evaluate data quality: Monitor and evaluate data quality to ensure that AI models are trained on high-quality data.
Conclusion
Poor data management can put AI projects at risk of failure. The consequences of poor data management can be severe, including delayed project timelines, increased costs, reduced model accuracy, and compliance and regulatory issues. To avoid these pitfalls, organizations must adopt effective data management practices, including metadata management, data quality controls, and data governance.
By understanding the importance of AI-ready data and adopting best practices for AI data management, organizations can ensure the success of their AI projects and unlock the full potential of AI technology.
News Source: