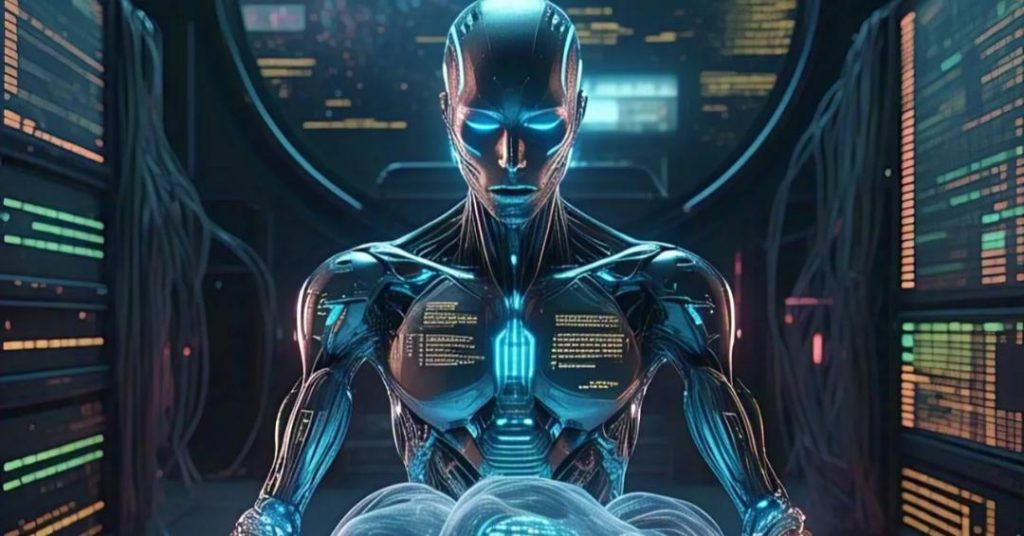
How Poor Data Management Can Put AI Projects at Risk?
Artificial intelligence (AI) has revolutionized the way businesses operate, making it easier to automate tasks, gain insights, and make data-driven decisions. However, for AI projects to succeed, organisations need AI-ready data, which is often lacking. According to a Gartner survey, 63% of organisations lack the necessary data, putting their AI projects at risk. This blog post explores the impact of poor data management on AI adoption and what organisations can do to overcome these challenges.
The AI Data Dilemma
The Gartner survey, which polled 1,203 data management leaders in 2024, found that organisations failing to distinguish between AI-ready requirements and traditional data management practices are endangering the success of their AI efforts. AI-ready data requires specific characteristics, such as high-quality, curated, and contextualised data, which are often lacking in traditional data management practices.
The survey highlights the growing concern among organisations about the lack of AI-ready data, which is hindering the adoption of AI technology. Poor data management practices, including inadequate metadata management and outdated data processing techniques, are contributing to this problem.
Metadata Management: The Unsung Hero of AI Projects
Metadata management is a critical aspect of AI projects, as it involves collecting, processing, and managing data to extract meaningful insights. However, many organisations neglect metadata management, leading to data inconsistencies, inaccuracies, and gaps. This can result in AI models that are biased, inaccurate, or fail to provide the expected results.
AI models rely on high-quality metadata to function correctly. Metadata includes information about the data, such as its origin, format, and quality. Poor metadata management can lead to:
- Data inconsistencies: Inaccurate or incomplete metadata can result in data inconsistencies, making it difficult to train AI models.
- Data inaccuracies: Inaccurate metadata can lead to incorrect data processing, resulting in biased or inaccurate AI models.
- Data gaps: Inadequate metadata management can result in data gaps, making it challenging to train AI models that can learn from the data.
Outdated Data Processing Techniques
Outdated data processing techniques are another significant obstacle to AI adoption. Traditional data processing techniques, such as batch processing and linear data processing, are not designed to handle the complexity and volume of data required for AI projects.
AI projects require real-time data processing, data streaming, and parallel processing to handle large volumes of data. Outdated data processing techniques can lead to:
- Slow data processing: Traditional data processing techniques can lead to slow data processing, making it difficult to train AI models in real-time.
- Inefficient data processing: Outdated data processing techniques can lead to inefficient data processing, resulting in wasted resources and increased costs.
- Data loss: Inadequate data processing techniques can result in data loss, making it challenging to train AI models that can learn from the data.
The Consequences of Poor Data Management
The consequences of poor data management are severe, and organisations that fail to address these challenges risk project failure, delayed timelines, and increased costs. The consequences of poor data management include:
- Project failure: Poor data management can lead to project failure, as AI models may not be able to learn from the data or provide accurate results.
- Delayed timelines: Inadequate data management can result in delayed timelines, as organisations may need to revisit and reprocess data to ensure it is AI-ready.
- Increased costs: Poor data management can result in increased costs, as organisations may need to invest in additional resources to manage and process data.
Best Practices for AI-Ready Data Management
To overcome the challenges of poor data management and ensure AI projects succeed, organisations can follow best practices for AI-ready data management, including:
- Metadata management: Implement a metadata management strategy to collect, process, and manage data with high-quality metadata.
- Data curation: Implement data curation practices to ensure data is accurate, complete, and consistent.
- Data processing: Implement real-time data processing, data streaming, and parallel processing techniques to handle large volumes of data.
- Data governance: Establish data governance practices to ensure data is properly managed, processed, and secured.
- AI-ready data engineering: Implement AI-ready data engineering practices to design and build data pipelines that are specifically designed for AI projects.
Conclusion
Poor data management is a significant obstacle to AI adoption, putting AI projects at risk. The lack of AI-ready data, inadequate metadata management, and outdated data processing techniques can result in project failure, delayed timelines, and increased costs. By implementing best practices for AI-ready data management, organisations can overcome these challenges and ensure the success of their AI projects. As the Gartner survey highlights, organisations that fail to distinguish between AI-ready requirements and traditional data management practices are putting their AI projects at risk. It is essential for organisations to prioritise AI-ready data management to reap the benefits of AI technology.
News Source: