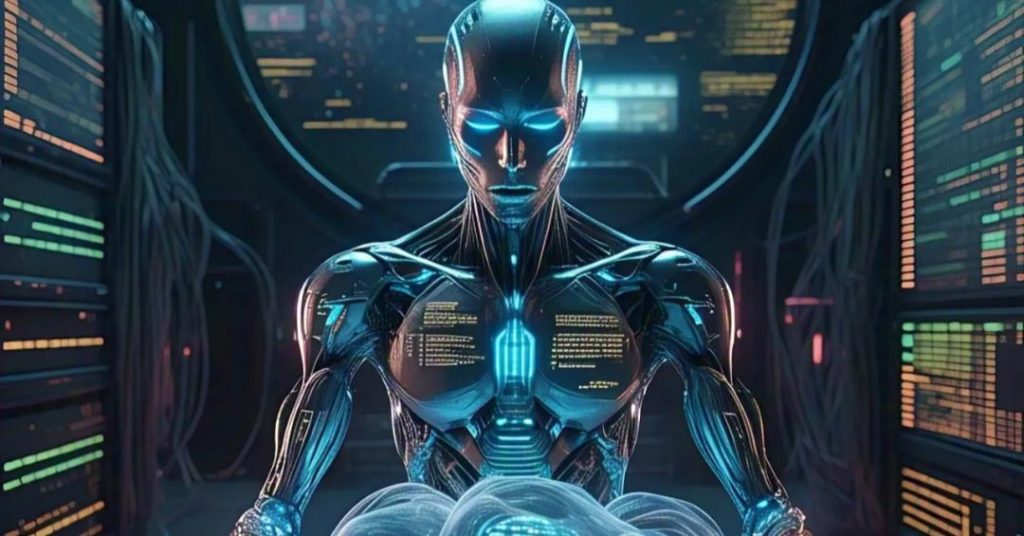
How Poor Data Management Can Put AI Projects at Risk?
The increasing adoption of Artificial Intelligence (AI) technology has brought about numerous benefits to businesses, including improved efficiency, enhanced decision-making, and competitive advantage. However, the success of AI projects depends heavily on the quality and availability of data. A recent survey by Gartner revealed that 63% of organisations lack AI-ready data, putting their AI projects at risk of failure. This alarming statistic highlights the importance of proper data management in ensuring the success of AI initiatives.
A survey of 1,203 data management leaders in 2024 found that organisations failing to realise the differences between AI-ready requirements and traditional data management endanger the success of their efforts. The survey also revealed that poor metadata management and outdated practices hinder AI adoption. These findings underscore the need for organisations to reassess their data management strategies to ensure they are equipped to support AI projects.
So, what exactly is AI-ready data, and why is it so crucial for AI projects? AI-ready data refers to data that is clean, accurate, complete, and readily available for use in AI models. This type of data is essential for AI algorithms to learn from, make predictions, and make decisions. AI models require massive amounts of data to train, validate, and test, and poor-quality data can lead to inaccurate results, biased decision-making, and poor performance.
The consequences of poor data management can be severe, leading to delays, cost overruns, and ultimately, project failure. In the worst-case scenario, poor data management can result in the loss of trust and reputation, as well as financial losses. Furthermore, poor data management can also lead to compliance issues, as AI models may produce biased or inaccurate results, which can have serious consequences in industries such as finance, healthcare, and law enforcement.
So, what are the common pitfalls of poor data management that can put AI projects at risk? Here are some of the most common issues:
- Data Silos: Data silos refer to the practice of storing data in isolated systems, making it difficult to access, integrate, and share data across the organisation. This can lead to data duplication, data quality issues, and poor data governance.
- Data Quality Issues: Poor data quality can result in inaccurate, incomplete, or inconsistent data, which can lead to biased AI models and poor performance.
- Lack of Data Governance: The lack of data governance can lead to data chaos, making it difficult to manage and maintain data quality, security, and compliance.
- Inadequate Metadata Management: Metadata refers to the data that describes other data, such as data origin, format, and quality. Inadequate metadata management can lead to data inconsistencies, data quality issues, and poor data integration.
- Outdated Practices: Outdated practices, such as manual data processing and data entry, can lead to errors, inaccuracies, and inefficiencies.
To avoid these pitfalls and ensure the success of AI projects, organisations must adopt a robust data management strategy that includes the following best practices:
- Data Integration: Integrate data from various sources to create a single, unified view of the data.
- Data Quality Management: Implement data quality management practices to ensure data accuracy, completeness, and consistency.
- Metadata Management: Implement metadata management practices to ensure data consistency, accuracy, and completeness.
- Data Governance: Establish data governance practices to ensure data quality, security, and compliance.
- Cloud-Based Data Management: Adopt cloud-based data management solutions to ensure scalability, flexibility, and cost-effectiveness.
In conclusion, poor data management can put AI projects at risk, leading to delays, cost overruns, and project failure. To ensure the success of AI initiatives, organisations must adopt a robust data management strategy that includes data integration, data quality management, metadata management, data governance, and cloud-based data management. By doing so, organisations can ensure they have the high-quality data needed to support AI projects and achieve their business goals.