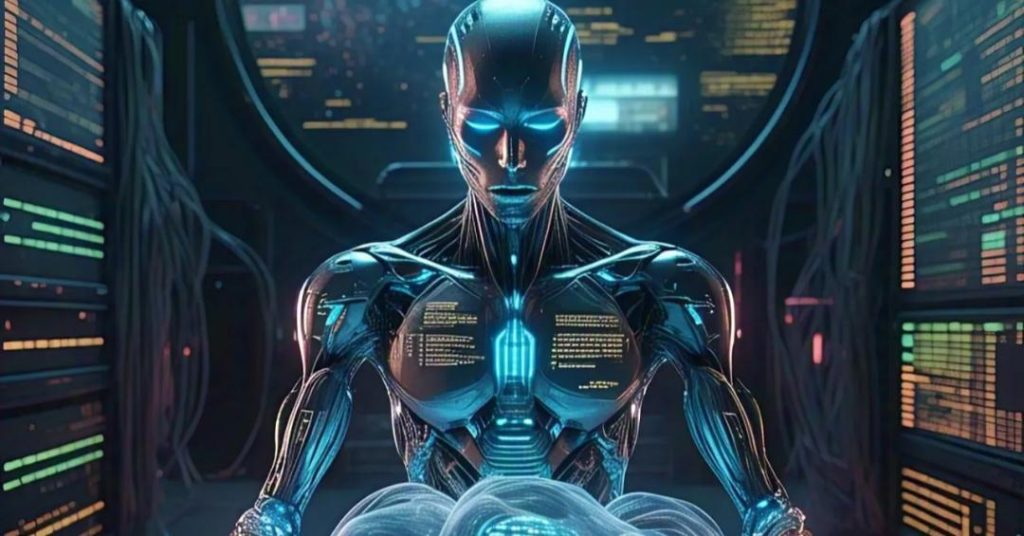
How Poor Data Management Can Put AI Projects at Risk?
Artificial Intelligence (AI) has revolutionized the way organizations operate, making it possible to automate tasks, gain insights, and drive business growth. However, AI projects often rely on high-quality data to produce accurate results. Unfortunately, many organizations are at risk of failing to deliver successful AI projects due to poor data management practices.
A recent survey conducted by Gartner found that 63% of organizations lack AI-ready data, which is a significant concern for companies investing heavily in AI projects. The survey, which polled 1,203 data management leaders in 2024, revealed that poor metadata management and outdated practices are major hindrances to AI adoption.
In this post, we’ll explore the data management challenges that can put AI projects at risk and offer insights on how to overcome these obstacles.
The Importance of AI-Ready Data
AI-ready data refers to high-quality, relevant, and accessible data that can be used to train AI models. This type of data is essential for AI applications such as machine learning, natural language processing, and predictive analytics. However, many organizations struggle to provide AI-ready data due to various reasons.
According to the Gartner survey, the lack of AI-ready data is attributed to several factors, including:
- Inadequate data governance: Poor data governance practices can lead to inconsistent and inaccurate data, which can negatively impact AI model performance.
- Incomplete data: Incomplete data sets can lead to biased AI models, which can have serious consequences in industries such as healthcare and finance.
- Outdated data: Outdated data can lead to inaccurate predictions and poor decision-making.
- Data quality issues: Data quality issues such as data duplication, inconsistencies, and errors can hinder AI model performance.
The Consequences of Poor Data Management
The consequences of poor data management can be severe, leading to the failure of AI projects. Some of the consequences include:
- Inaccurate predictions: Poor data quality can lead to inaccurate predictions, which can have serious consequences in industries such as finance and healthcare.
- Biased AI models: Biased AI models can perpetuate discrimination and inequality, leading to legal and reputational risks.
- Delayed project timelines: Poor data management can lead to delays in project timelines, causing significant financial losses.
- Increased costs: Poor data management can lead to increased costs, including data cleaning, processing, and storage costs.
Metadata Management and AI Adoption
Metadata management is critical for AI adoption. Metadata refers to the data that describes other data, such as data type, format, and location. Poor metadata management can lead to data inconsistencies and inaccuracies, which can negatively impact AI model performance.
According to the Gartner survey, 63% of organizations lack AI-ready data, which is a significant concern for companies investing heavily in AI projects. The survey found that poor metadata management and outdated practices are major hindrances to AI adoption.
How to Overcome Data Management Challenges
To overcome data management challenges and ensure the success of AI projects, organizations must adopt best practices in data management. Some of the best practices include:
- Implement data governance: Establish data governance policies and procedures to ensure data quality and consistency.
- Invest in data quality tools: Invest in data quality tools to detect and correct data errors and inconsistencies.
- Use metadata management tools: Use metadata management tools to manage data metadata and ensure data consistency and accuracy.
- Implement data analytics: Implement data analytics to gain insights into data quality and identify areas for improvement.
- Invest in AI-ready data: Invest in AI-ready data to ensure high-quality data for AI applications.
Conclusion
Poor data management can put AI projects at risk, leading to inaccurate predictions, biased AI models, delayed project timelines, and increased costs. To overcome data management challenges, organizations must adopt best practices in data management, including data governance, data quality tools, metadata management tools, data analytics, and AI-ready data.
By following these best practices, organizations can ensure the success of AI projects and reap the benefits of AI adoption. As the Gartner survey suggests, poor metadata management and outdated practices are major hindrances to AI adoption, and organizations must prioritize data management to ensure the success of their AI projects.
Source: