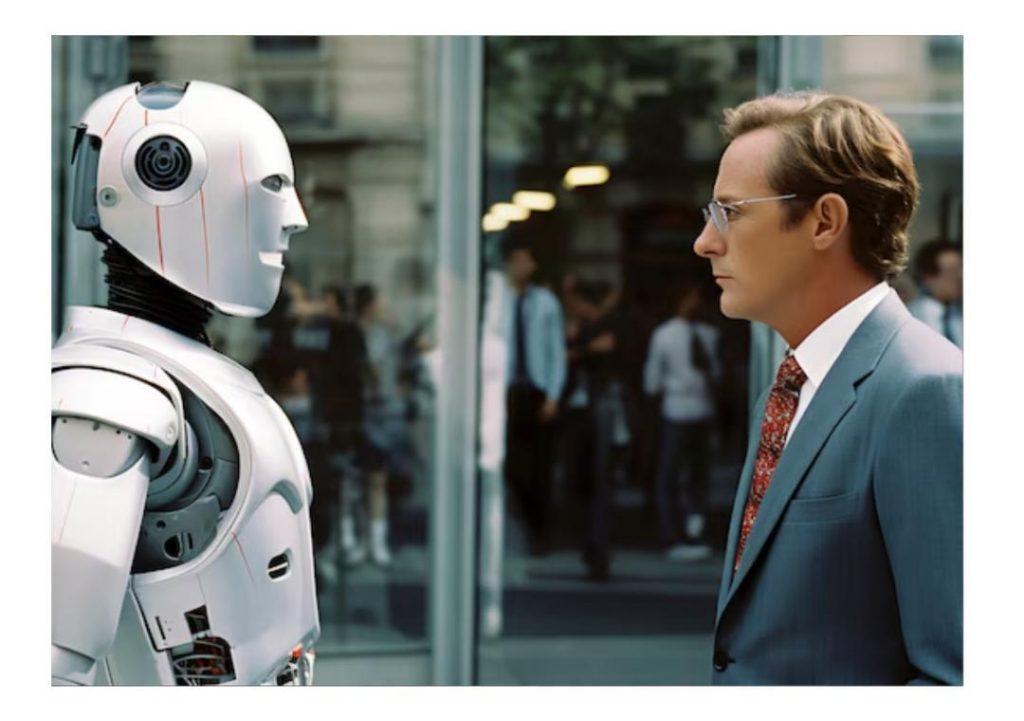
Is GenAI Smart Enough to Avoid Bad Advice?
The advent of Generative Adversarial Networks (GANs) and Large Language Models (LLMs) has revolutionized the way we interact with artificial intelligence. These models, collectively referred to as GenAI, have shown remarkable capabilities in generating human-like text, images, and even videos. However, as with any powerful technology, there are concerns about the potential pitfalls of relying solely on GenAI for decision-making. One of the most critical issues is the risk of bad advice, which can arise from the model’s inability to distinguish between accurate and inaccurate information.
The speed and scale of GenAI can lead to surface-level answers or hallucinated facts. Without the right human guardrails, insights can be misleading, and even worse, lead to catastrophic decisions. In this blog post, we’ll explore the challenges of GenAI-generated advice, the importance of human oversight, and the necessary measures firms must take to ensure the integrity of their AI-driven decision-making processes.
The Dangers of Surface-Level Analysis
GenAI models are designed to process vast amounts of data quickly and efficiently. While this speed can be beneficial in many situations, it also means that the models may not have the time or resources to delve deeper into complex issues. This can result in surface-level answers that may not be entirely accurate or relevant.
For instance, when a user asks a GenAI model to summarize a lengthy article, the model may focus on the most prominent keywords rather than the underlying arguments or nuances. This can lead to a simplified or even misleading understanding of the topic, which can have significant consequences in fields like healthcare, finance, or law.
The Hallucination Problem
Another concern is the phenomenon of “hallucinated facts,” where GenAI models generate factual statements that are entirely false. This can occur when the model is trained on biased or incomplete data, or when it uses shortcuts to generate responses rather than relying on actual evidence.
For example, a GenAI model trained on social media data may generate a statement claiming that a particular political candidate has a high approval rating, even if there is no evidence to support this claim. This can be particularly damaging in political or social contexts where accurate information is crucial for informed decision-making.
The Need for Human Oversight
While GenAI models can process vast amounts of data quickly and efficiently, they are not capable of critical thinking or nuanced analysis. Therefore, it is essential to have human experts review and validate the output of GenAI models to ensure that the insights are accurate, relevant, and actionable.
Firms must build in checks validating data, ensuring bias control, and clarifying sources before acting on AI output. This can involve techniques such as:
- Data validation: Verifying the accuracy and completeness of the data used to train the GenAI model.
- Bias detection: Identifying and mitigating biases in the data or model output.
- Source clarification: Determining the origin and credibility of the information generated by the GenAI model.
- Human review: Conducting regular human review and validation of the GenAI output to ensure it is accurate, relevant, and actionable.
The Importance of Critical Thinking
Critical thinking remains essential to ensure that AI recommendations are not taken at face value. Humans must be able to analyze the output of GenAI models, consider alternative perspectives, and make informed decisions that take into account the limitations and potential biases of the AI system.
In the age of GenAI, firms must prioritize critical thinking and human oversight to ensure that AI-driven decision-making processes are transparent, accountable, and effective. This can involve implementing robust governance frameworks, conducting regular training and education programs for employees, and fostering a culture of transparency and accountability.
Conclusion
While GenAI models have shown remarkable capabilities in generating human-like text and images, they are not infallible. The risk of bad advice, surface-level analysis, and hallucinated facts is real, and firms must take proactive measures to mitigate these risks. By building in checks validating data, ensuring bias control, and clarifying sources, and prioritizing critical thinking and human oversight, firms can ensure that AI-driven decision-making processes are robust, reliable, and effective.
Source:
https://www.growthjockey.com/blogs/consulting-in-the-age-of-generative-ai