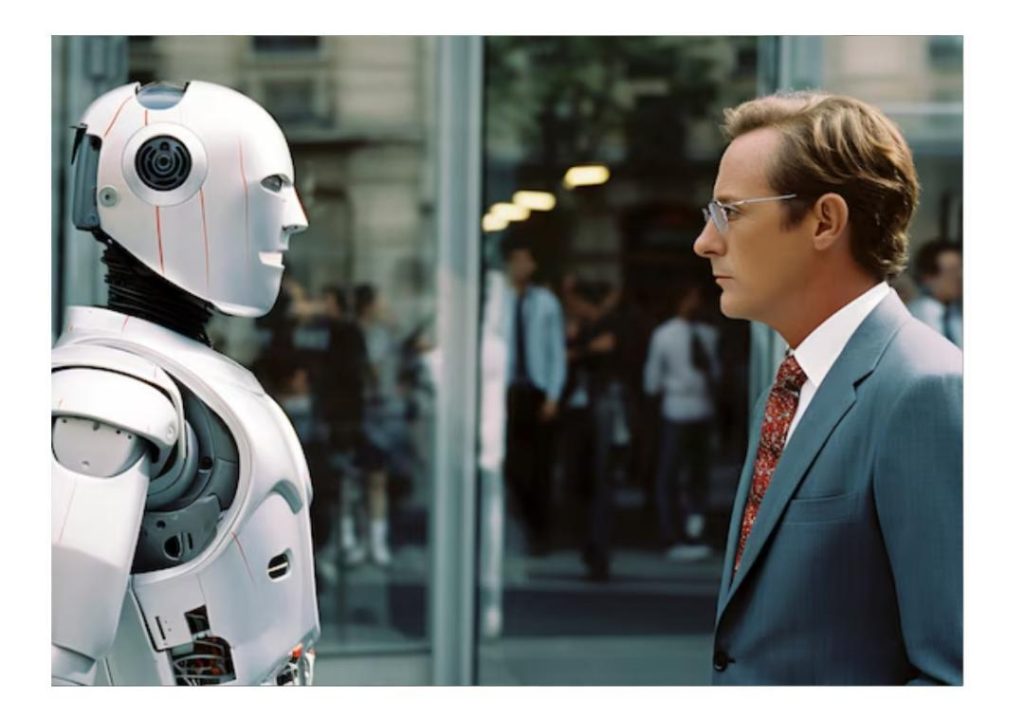
Is GenAI Smart Enough to Avoid Bad Advice?
The advent of Generative Artificial Intelligence (GenAI) has revolutionized the way we approach problem-solving and decision-making. With its unparalleled speed and ability to generate vast amounts of data, GenAI has the potential to unlock unprecedented insights and opportunities. However, as we increasingly rely on AI to inform our decisions, it’s essential to consider the potential pitfalls that come with its use. Specifically, can GenAI avoid providing bad advice, and what measures can we take to ensure the accuracy and reliability of its outputs?
The Risks of Surface-Level Answers
One of the primary concerns surrounding GenAI is its potential to provide surface-level answers or “hallucinated facts.” This phenomenon occurs when AI algorithms generate responses based on patterns and associations in the data, rather than a deep understanding of the underlying concepts. While this may seem impressive at first, it can lead to flawed or misleading information being presented as fact.
For instance, imagine an AI-powered chatbot that assists customers with product recommendations. Without adequate training data or human oversight, the chatbot may rely on superficial correlations between product features and customer preferences, rather than considering the nuances of individual needs and contexts. This could result in recommendations that are misaligned with customers’ actual requirements, leading to frustration and disappointment.
The Importance of Human Guardrails
To mitigate the risks associated with GenAI, it’s essential to build in checks and balances that validate the accuracy and reliability of its outputs. This involves implementing human guardrails that ensure AI-generated insights are grounded in fact, unbiased, and clearly sourced. Some strategies for achieving this include:
- Data validation: Verify the accuracy and completeness of the data used to train AI models. This may involve manual review, data cleaning, and validation against external sources.
- Bias control: Implement techniques to identify and mitigate biases in the data and AI algorithms. This may include using diverse training datasets, monitoring algorithm performance, and implementing fairness metrics.
- Source clarification: Ensure that AI outputs are clearly attributed to their sources, and that users understand the limitations and potential biases of the underlying data.
- Human oversight: Integrate human experts into the AI decision-making process to review and validate outputs, especially in critical or high-stakes applications.
Critical Thinking Remains Essential
While GenAI can process vast amounts of data and identify patterns, it is still a machine. It lacks the critical thinking and nuanced understanding that humans bring to decision-making. As such, it’s crucial to approach AI-generated insights with skepticism and rigor, rather than taking them at face value.
Best Practices for Working with GenAI
To ensure the accuracy and reliability of GenAI outputs, firms should adopt the following best practices:
- Define clear objectives: Establish specific goals and requirements for AI-generated insights, and ensure that the AI system is designed to meet those objectives.
- Monitor and evaluate performance: Continuously monitor AI performance, and evaluate its outputs against established standards and benchmarks.
- Integrate human expertise: Involve human experts in the AI decision-making process to provide context, nuance, and critical thinking.
- Foster a culture of skepticism: Encourage a culture of skepticism and rigor within your organization, and ensure that AI-generated insights are subject to regular review and validation.
Conclusion
GenAI has the potential to revolutionize the way we approach problem-solving and decision-making. However, its speed and ability to generate vast amounts of data also present significant risks, including the provision of surface-level answers or hallucinated facts. To mitigate these risks, it’s essential to build in checks and balances that validate the accuracy and reliability of AI-generated insights. By implementing human guardrails, fostering a culture of skepticism, and integrating human expertise into the AI decision-making process, firms can ensure that GenAI recommendations are not taken at face value, and that critical thinking remains essential to informed decision-making.
Source:
https://www.growthjockey.com/blogs/consulting-in-the-age-of-generative-ai