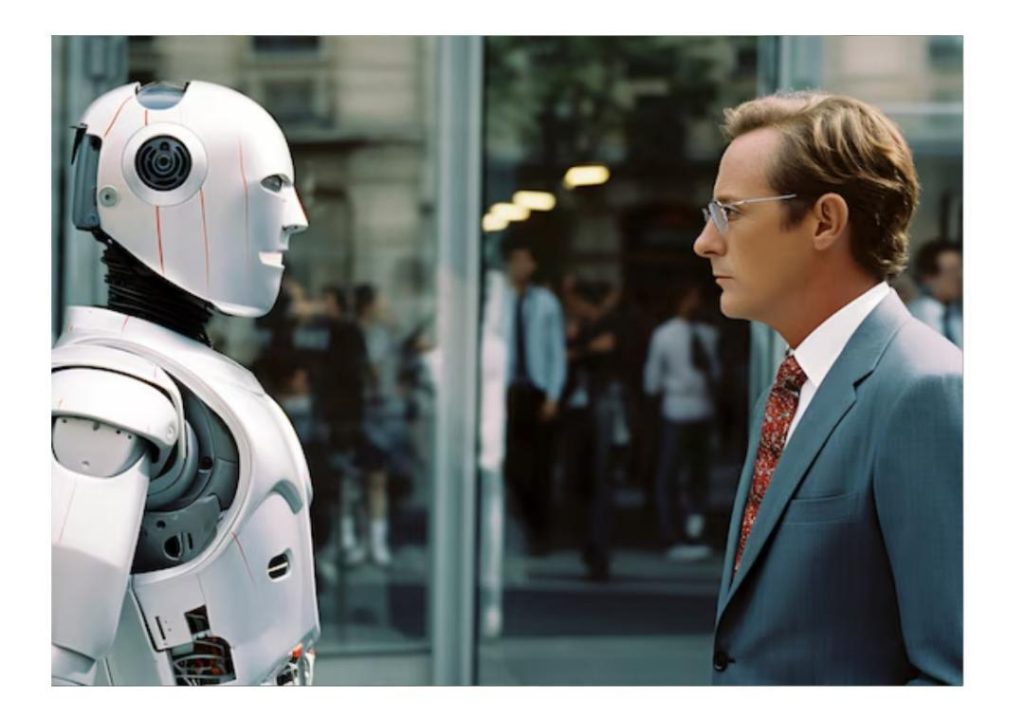
Is GenAI Smart Enough to Avoid Bad Advice?
The advent of Generative Adversarial Networks (GANs) and Large Language Models (LLMs) has revolutionized the field of artificial intelligence, enabling machines to generate human-like text, images, and even entire conversations. GenAI, as it is often referred to, has the potential to transform industries, streamline processes, and unlock new insights. However, as we increasingly rely on GenAI for guidance, it is essential to ask a crucial question: Is GenAI smart enough to avoid bad advice?
The speed and scale of GenAI can lead to surface-level answers or hallucinated facts. Without the right human guardrails, insights can be misleading, and decisions can be made based on flawed data. The risk of relying solely on GenAI is that we may be ignoring the nuances and complexities of human experience. Firms must build in checks to validate data, ensure bias control, and clarify sources before acting on AI output. Critical thinking remains essential to ensure AI recommendations aren’t taken at face value.
The Dangers of Surface-Level Answers
One of the primary concerns with GenAI is its ability to generate answers based on patterns and associations within the data it has been trained on. While this can be incredibly useful for generating ideas and hypotheses, it can also lead to surface-level answers that lack depth and context. For example, a GenAI system may provide a list of potential solutions to a complex problem without considering the potential unintended consequences or long-term implications.
Moreover, GenAI is only as good as the data it is trained on. If the data is biased, incomplete, or inaccurate, the insights generated by the AI will be similarly flawed. This is particularly concerning in fields such as healthcare, finance, and law, where decisions have significant consequences for individuals and society.
The Risk of Hallucinated Facts
Another concern with GenAI is the risk of hallucinated facts, which are false facts that are generated by the AI as if they were true. This can occur when the AI is attempting to fill in gaps in its knowledge or when it is simply making assumptions based on patterns it has learned. Hallucinated facts can have devastating consequences, particularly in fields such as science, where incorrect information can mislead researchers and lead to incorrect conclusions.
For example, a study published in the journal Nature found that a language model was able to generate false information about the mating habits of a particular species of fish. The study’s authors noted that the AI was able to generate these false facts because it had learned patterns and associations within the data it was trained on, rather than verifying the accuracy of the information.
The Importance of Human Guardrails
Given the risks associated with GenAI, it is essential that firms build in human guardrails to ensure that the insights generated by the AI are accurate, reliable, and actionable. This can be achieved through a combination of human oversight, data validation, and bias control.
Human oversight involves having human experts review and validate the insights generated by the AI. This can help to identify and correct errors, as well as provide context and nuance to the insights generated by the AI.
Data validation involves ensuring that the data used to train the AI is accurate, complete, and unbiased. This can be achieved through a combination of data cleaning, data validation, and data annotation.
Bias control involves ensuring that the AI is trained on diverse and representative data, and that it is not biased towards certain groups or individuals. This can be achieved through a combination of data augmentation, data balancing, and algorithmic design.
The Role of Critical Thinking
Finally, critical thinking remains essential when working with GenAI. It is not enough to simply rely on the insights generated by the AI; rather, we must also consider the underlying assumptions, biases, and limitations of the AI. We must also consider alternative perspectives and challenge our own assumptions.
Critical thinking is essential because GenAI is only as good as the data it is trained on, and because it is only as good as the assumptions and biases it has learned. By combining human oversight, data validation, bias control, and critical thinking, we can ensure that the insights generated by GenAI are accurate, reliable, and actionable.
Conclusion
In conclusion, while GenAI has the potential to revolutionize industries and unlock new insights, it is essential that firms build in checks to validate data, ensure bias control, and clarify sources before acting on AI output. Critical thinking remains essential to ensure AI recommendations aren’t taken at face value. By combining human oversight, data validation, bias control, and critical thinking, we can ensure that GenAI is used in a responsible and effective manner.
Source:
https://www.growthjockey.com/blogs/consulting-in-the-age-of-generative-ai