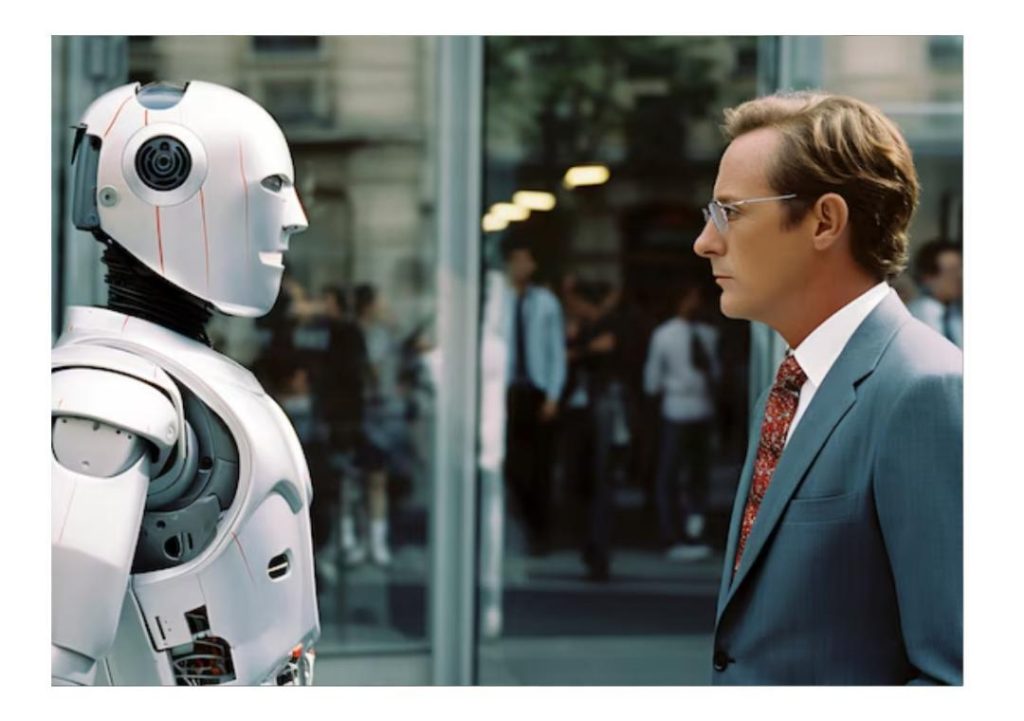
Is GenAI Smart Enough to Avoid Bad Advice?
The advent of Generative AI (GenAI) has revolutionized the way we approach problem-solving and decision-making. With its lightning-fast processing capabilities and ability to generate vast amounts of data, GenAI has become an indispensable tool for businesses and organizations. However, as we increasingly rely on GenAI to provide insights and recommendations, it’s essential to ask: is GenAI smart enough to avoid bad advice?
The answer is a resounding “maybe.” While GenAI has made tremendous progress in recent years, it’s not without its limitations. The speed and scale at which GenAI processes information can sometimes lead to surface-level answers or even hallucinated facts. Without the right human guardrails, insights can be misleading, and firms must take proactive steps to ensure that GenAI recommendations are accurate and reliable.
In this blog post, we’ll explore the challenges of relying solely on GenAI for advice, the potential risks of unchecked AI output, and the importance of building in checks to validate data, control bias, and clarify sources.
The Limits of GenAI
GenAI’s strength lies in its ability to process vast amounts of data quickly and efficiently. This allows it to identify patterns, make connections, and generate insights that would be impossible for humans to achieve on their own. However, this very strength also creates challenges.
One of the primary limitations of GenAI is its lack of contextual understanding. While it can process vast amounts of data, it often lacks the nuance and context that humans take for granted. This can lead to surface-level answers or oversimplifications that may not accurately reflect the complexity of a situation.
For example, consider a firm seeking to optimize its supply chain. GenAI might quickly identify patterns and generate recommendations for streamlining logistics and reducing costs. However, without a deep understanding of the firm’s specific business needs and market context, the AI’s recommendations may not be tailored to the company’s unique situation.
Another limitation of GenAI is its susceptibility to bias. The data used to train AI models can be biased, reflecting the biases of the humans who created and curated the data. This can lead to inaccurate or misleading insights, particularly when it comes to sensitive topics such as social justice, ethics, or human rights.
The Risks of Unchecked AI Output
The risks of unchecked AI output are numerous and potentially far-reaching. When firms rely solely on GenAI for advice, they may miss critical aspects of a situation or fail to consider alternative perspectives. This can lead to poor decision-making, costly mistakes, or even catastrophic consequences.
For example, consider a financial institution using GenAI to make lending decisions. Without proper human oversight, the AI may prioritize speed and efficiency over careful consideration of the borrower’s creditworthiness or financial situation. This could result in reckless lending, increased defaults, and even financial instability.
Similarly, in the healthcare sector, unchecked AI output could lead to misdiagnosis or mistreatment of patients. Without human medical expertise and judgment, AI may misinterpret medical data or fail to consider the patient’s individual circumstances, leading to catastrophic consequences.
Building in Checks and Balances
To mitigate the risks associated with GenAI, firms must build in checks and balances to validate data, control bias, and clarify sources. This requires a proactive approach to AI development and deployment, including:
- Data validation: Firms must ensure that the data used to train AI models is accurate, complete, and representative of the target audience.
- Bias control: Firms must take steps to minimize bias in AI models, including using diverse and representative training data, regular auditing, and human oversight.
- Source clarification: Firms must clarify the sources of AI-generated insights and recommendations, ensuring that users understand the limitations and potential biases of the AI output.
By building in these checks and balances, firms can ensure that GenAI recommendations are accurate, reliable, and actionable. This requires a combination of human expertise, domain knowledge, and careful consideration of the potential risks and limitations of AI.
Conclusion
GenAI has the potential to revolutionize the way we approach problem-solving and decision-making. However, its speed and scale also create challenges that require careful consideration. Firms must build in checks and balances to validate data, control bias, and clarify sources, ensuring that GenAI recommendations are accurate, reliable, and actionable.
Critical thinking remains essential to ensure that AI recommendations are not taken at face value. By combining human expertise with AI-generated insights, firms can unlock the full potential of GenAI while minimizing the risks associated with its use.
Source: https://www.growthjockey.com/blogs/consulting-in-the-age-of-generative-ai