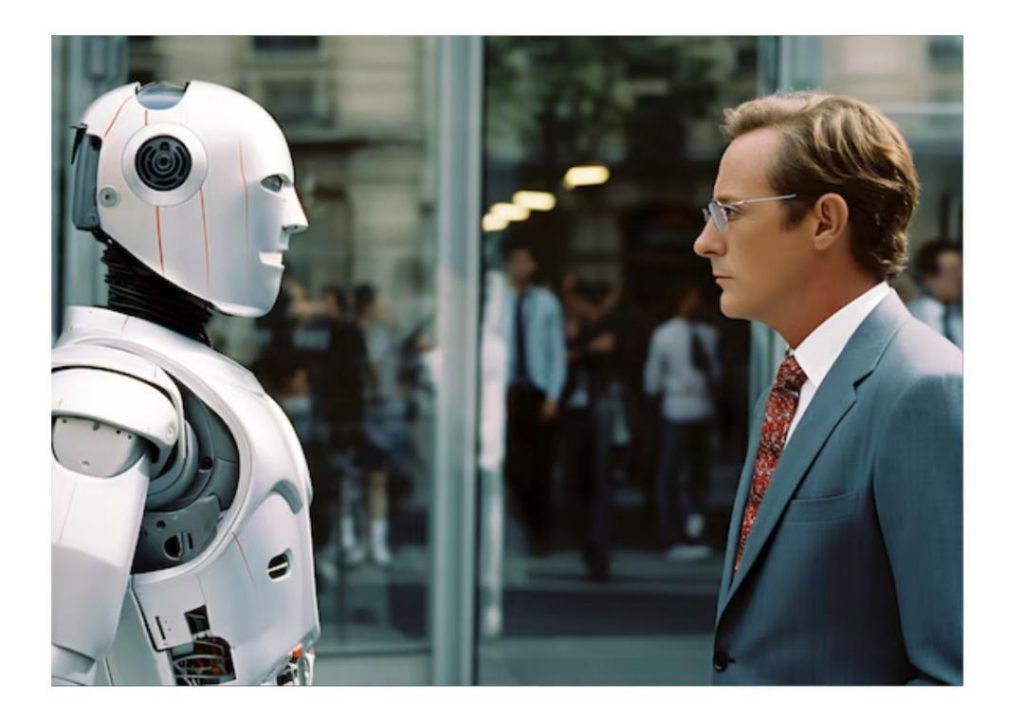
Is GenAI Smart Enough to Avoid Bad Advice?
The advent of Generative AI (GenAI) has revolutionized the way we approach problem-solving, decision-making, and knowledge discovery. With its unparalleled speed and processing power, GenAI can generate answers, insights, and recommendations at an unprecedented scale. However, as we increasingly rely on AI-driven outputs, it’s crucial to examine whether GenAI is smart enough to avoid bad advice and provide accurate, reliable, and actionable insights.
The speed of GenAI can lead to surface-level answers or hallucinated facts. Without the right human guardrails, insights can be misleading. Firms must build in checks validating data, ensuring bias control, and clarifying sources before acting on AI output. Critical thinking remains essential to ensure AI recommendations aren’t taken at face value.
GenAI’s impressive capabilities are rooted in its ability to process vast amounts of data and identify patterns, connections, and relationships that would be impossible for humans to discern. This power has led to breakthroughs in fields like medicine, finance, and marketing. However, this same power also increases the risk of errors, biases, and misinformation.
One of the primary concerns is the potential for GenAI to perpetuate existing biases and amplify discriminatory patterns. AI systems are only as good as the data they were trained on, and if this data is flawed or biased, the output will inevitably reflect these biases. This can lead to inaccurate predictions, unfair decisions, and perpetuation of systemic inequalities.
Another challenge is the risk of superficial answers. GenAI’s speed and processing power can lead to quick, surface-level solutions that may not address the underlying complexities of a problem. This can result in short-term fixes that ultimately exacerbate the issue or create new problems.
A third concern is the potential for hallucinated facts. GenAI’s ability to generate text, images, and audio can lead to the creation of fake or misleading information. This can be particularly problematic in fields like journalism, academia, and politics, where accuracy and credibility are paramount.
To mitigate these risks, firms must implement robust checks and balances to validate GenAI output. This includes:
- Data validation: Ensure that the data used to train the AI system is accurate, comprehensive, and representative of the issue at hand.
- Bias control: Implement algorithms and techniques to detect and mitigate biases in the data and output.
- Source clarification: Verify the credibility and reliability of sources cited in AI-driven recommendations.
- Human oversight: Integrate human experts and critical thinkers to review and validate AI output.
- Transparency: Provide clear explanations of AI-driven recommendations, including the data used, algorithms employed, and potential biases.
In addition to these technical measures, it’s essential to develop a culture of critical thinking and skepticism in organizations. This includes:
- Questioning AI output: Encourage employees to question and critically evaluate AI-driven recommendations, rather than accepting them at face value.
- Seeking diverse perspectives: Foster a culture of diverse perspectives and opinions, to mitigate the risk of groupthink and biased thinking.
- Continuous learning: Encourage ongoing learning and professional development, to stay up-to-date with the latest advancements in AI and its applications.
In conclusion, while GenAI has the potential to revolutionize the way we approach problem-solving and decision-making, it’s crucial to acknowledge its limitations and biases. By implementing robust checks and balances, fostering a culture of critical thinking, and ensuring transparency and accountability, firms can harness the power of GenAI without compromising accuracy, reliability, and trust.
Source: https://www.growthjockey.com/blogs/consulting-in-the-age-of-generative-ai