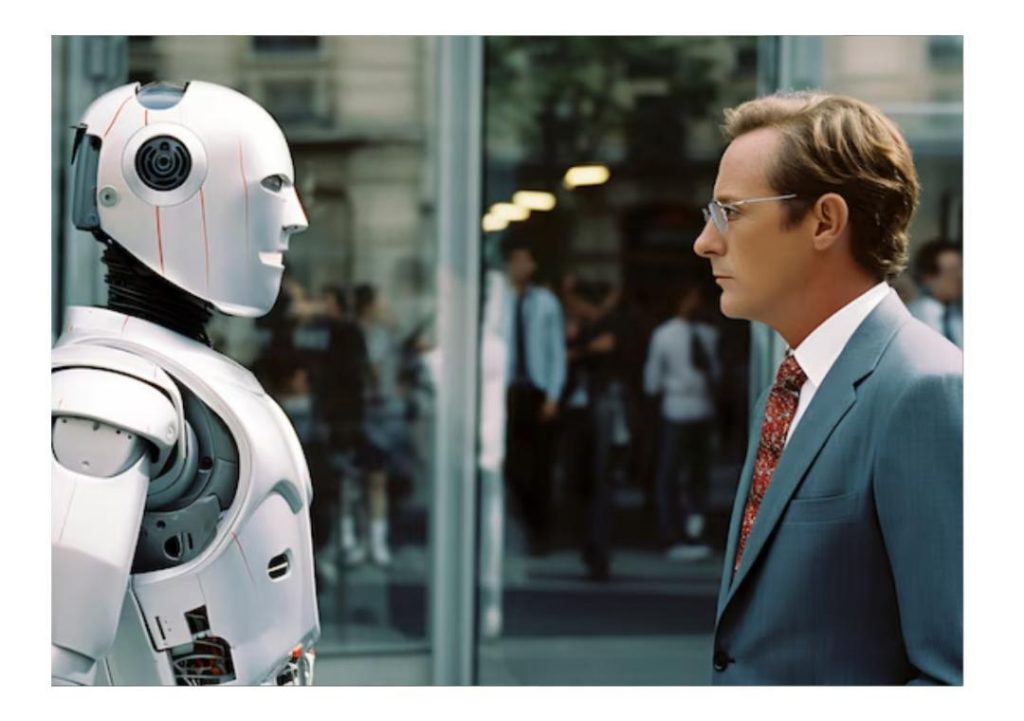
Is GenAI Smart Enough to Avoid Bad Advice?
The advent of Generative AI (GenAI) has revolutionized the way we approach decision-making, offering unprecedented speed and accuracy in generating insights. However, as we increasingly rely on AI to inform our decisions, it’s essential to ask: can GenAI truly avoid bad advice? The answer lies in the complexity of AI’s capabilities and our capacity to validate its outputs.
GenAI’s remarkable ability to process vast amounts of data and generate responses in real-time has led to its widespread adoption in various industries, from healthcare to finance. This technology can quickly analyze large datasets, identify patterns, and provide answers to complex questions. While this sounds like a dream come true, there’s a crucial caveat: GenAI’s outputs may not always be accurate or reliable.
The Speed of GenAI Can Lead to Surface-Level Answers
GenAI’s lightning-fast processing capabilities can sometimes result in superficial answers or “hallucinated facts.” These are facts that may appear plausible but are actually incorrect. Without proper human oversight, these incorrect facts can be disseminated and perpetuated, leading to misguided decisions.
For instance, consider a scenario where a financial institution uses GenAI to analyze market trends. The AI system quickly processes vast amounts of data and provides a prediction about the stock market’s future performance. The prediction may seem convincing, but without validation, it may be based on incomplete or outdated information. This could lead to costly investment decisions that ultimately harm the institution.
Bias Control: A Critical Component of GenAI Validation
Another significant concern is bias control. GenAI systems can perpetuate existing biases in the data they’re trained on, leading to inaccurate or discriminatory results. For example, if a GenAI system is trained on a dataset that contains biased language, it may learn to reproduce that bias in its output. This can have severe consequences, particularly in high-stakes applications like law enforcement or healthcare.
To mitigate these risks, firms must build in checks to validate data and ensure bias control. This can be achieved through:
- Data cleansing: Regularly reviewing and updating datasets to eliminate errors and biases.
- Diversity in training data: Ensuring that training data represents diverse perspectives and experiences.
- Bias detection algorithms: Implementing algorithms that detect and correct biases in the data.
Clarifying Sources: The Key to Accurate Insights
Another essential step in ensuring the accuracy of GenAI outputs is clarifying sources. This involves understanding where the data used to train the AI comes from and what assumptions are built into the model. By understanding the sources and assumptions, firms can better evaluate the reliability of the insights generated.
For instance, if a GenAI system is trained on social media data, firms should be aware that this data may be biased or incomplete. They should also understand the assumptions built into the model, such as the weightage given to different sources or the criteria used to select data.
Critical Thinking Remains Essential
As we increasingly rely on GenAI for insights, it’s essential to recognize that critical thinking remains a crucial component of decision-making. AI systems are only as good as the data they’re trained on and the assumptions built into the model. Without proper human oversight and validation, AI recommendations can be taken at face value, leading to misguided decisions.
Firms must adopt a hybrid approach that combines the speed and scale of GenAI with human expertise and critical thinking. This involves:
- Collaborative decision-making: Working closely with AI systems to ensure that insights are validated and verified by human experts.
- Explainability and transparency: Ensuring that AI systems provide clear explanations for their outputs and that firms understand the assumptions and biases built into the model.
- Continuous monitoring: Regularly monitoring AI outputs and updating models to reflect changes in data and assumptions.
Conclusion
GenAI has revolutionized the way we approach decision-making, offering unprecedented speed and accuracy in generating insights. However, as we increasingly rely on AI to inform our decisions, it’s essential to recognize the limitations and potential pitfalls of this technology.
Firms must build in checks to validate data, ensure bias control, and clarify sources before acting on AI output. Critical thinking remains essential to ensure that AI recommendations aren’t taken at face value. By adopting a hybrid approach that combines the strengths of human experts with the capabilities of GenAI, firms can harness the power of this technology while minimizing the risk of bad advice.
Source:
https://www.growthjockey.com/blogs/consulting-in-the-age-of-generative-ai