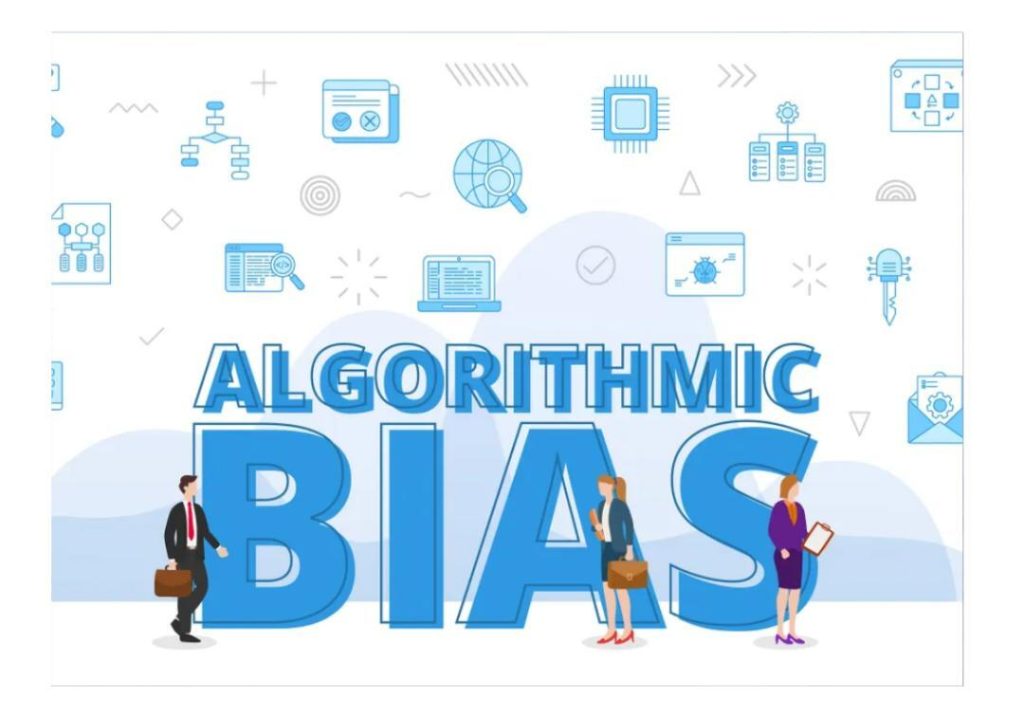
Is Your AI System Reinforcing Real-World Bias?
Artificial intelligence (AI) has revolutionized the way we live and work, from personalized recommendations to autonomous vehicles. However, the development and deployment of AI systems have also raised serious concerns about bias and fairness. As AI becomes increasingly integrated into critical decision-making processes, such as hiring, lending, and policing, it is essential to ensure that these systems do not perpetuate existing biases and inequalities.
Unfortunately, AI systems can be biased, not because they are inherently flawed, but because they are only as objective as the data and models used to train them. Without proper checks and balances, biased inputs can lead to unfair outputs, exacerbating existing social and economic inequalities.
In India, the government has taken a proactive approach to address algorithmic bias as a core challenge, rather than a side effect. The country’s AI regulation roadmap is focused on ensuring that AI systems are transparent, explainable, and fair. This is a significant step towards building trust in AI and ensuring that these systems do not perpetuate harmful biases.
What is Algorithmic Bias?
Algorithmic bias refers to the unfair or discriminatory outcomes that result from AI systems’ reliance on biased data or flawed decision-making processes. This can occur in various ways, including:
- Data bias: AI systems learn from the data they are trained on, which means that they can perpetuate biases present in that data. For example, if a dataset used to train an AI system is predominantly composed of male voices, the system may be less accurate in recognizing female voices.
- Model bias: AI systems can also be biased due to the design of the algorithm or model used to develop them. For instance, a machine learning model may be more likely to recognize white faces than black faces if it is trained on a dataset that is predominantly composed of white faces.
- Selection bias: AI systems may also be biased due to the selection of data or features used to train them. For example, if a dataset used to train an AI system is skewed towards a particular geographic region or demographic group, the system may be less accurate or less fair for other groups.
The Consequences of Algorithmic Bias
Algorithmic bias can have serious consequences, particularly in domains such as:
- Hiring: AI-powered hiring systems may disproportionately reject candidates from underrepresented groups, perpetuating existing inequalities in the job market.
- Lending: AI-powered lending systems may deny loans to individuals or businesses from marginalized communities, exacerbating financial inequalities.
- Policing: AI-powered policing systems may disproportionately target certain racial or ethnic groups, perpetuating systemic racism and discrimination.
India’s AI Regulation Roadmap
In response to the growing concerns about algorithmic bias, the Indian government has launched a comprehensive AI regulation roadmap. The roadmap is focused on ensuring that AI systems are transparent, explainable, and fair, and that they do not perpetuate harmful biases.
Some of the key measures proposed in the roadmap include:
- Explainability: AI systems must be designed to provide clear and transparent explanations for their decisions and outputs.
- Auditability: AI systems must be designed to allow for regular audits and testing to identify and mitigate biases.
- Data quality: AI systems must be trained on high-quality, diverse, and representative data to minimize the risk of bias.
- Human oversight: AI systems must be designed to allow for human oversight and intervention to ensure that they are functioning fairly and ethically.
Building Trust in AI
Building trust in AI is crucial to ensuring that these systems are used effectively and ethically. To build trust, it is essential to ensure that AI systems are transparent, explainable, and fair. This can be achieved through:
- Transparency: AI systems must be designed to provide clear and transparent explanations for their decisions and outputs.
- Explainability: AI systems must be designed to provide clear and transparent explanations for their decisions and outputs.
- Accountability: AI systems must be designed to be accountable to humans, and to allow for human intervention and oversight.
- Ethical considerations: AI systems must be designed with ethical considerations in mind, and must be tested and evaluated against ethical and moral principles.
Conclusion
Algorithmic bias is a significant challenge in the development and deployment of AI systems. India’s AI regulation roadmap is a step towards ensuring that these systems are transparent, explainable, and fair. By prioritizing explainability, auditability, data quality, and human oversight, we can ensure that AI systems do not perpetuate harmful biases and that they are used effectively and ethically.
References
https://www.growthjockey.com/blogs/ethical-use-of-ai-laws-in-india