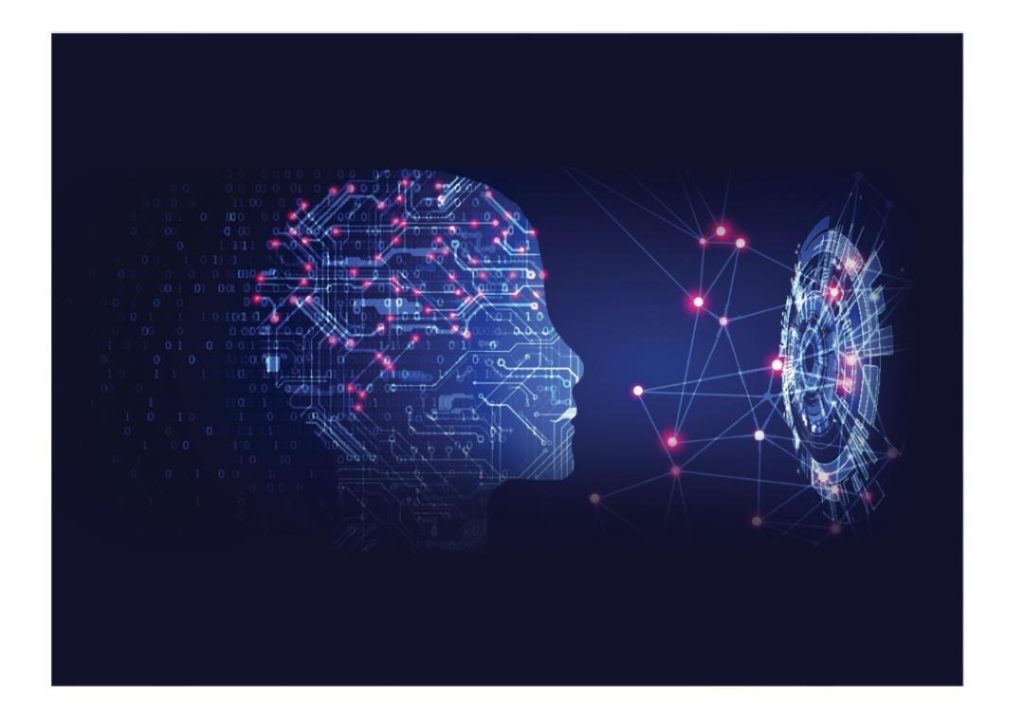
Should AI Decisions be Explainable to the Average User?
The increasing reliance on artificial intelligence (AI) to make decisions is revolutionizing various industries, from credit scoring to medical recommendations. While AI has the potential to improve efficiency and accuracy, it also raises concerns about accountability and transparency. As AI influences more decisions that affect our daily lives, it becomes essential to ensure that users can understand how these outputs were generated. In this blog post, we’ll explore the importance of explainability in AI decision-making and why it’s crucial for responsible AI adoption.
What is Explainability in AI?
Explainability refers to the ability of AI systems to provide clear and transparent information about their decision-making processes. This includes the logic, data, and algorithms used to generate outputs. Explainability is not just about providing a detailed explanation of how AI works, but also about making the information accessible to non-technical users.
The Importance of Explainability
Explainability is essential for several reasons:
- Accountability: Without explainability, AI decisions can seem arbitrary and unaccountable. When users can’t understand how AI arrived at a particular decision, they may question its validity and trustworthiness.
- Transparency: Transparency is the foundation of responsible AI adoption. By providing clear explanations, AI systems demonstrate their integrity and commitment to fairness, justice, and equality.
- Trust: Users need to trust AI systems to make decisions that affect their lives. Explainability helps build trust by providing a clear understanding of how AI works and what factors influence its decisions.
- Fairness: Explainability ensures that AI decisions are fair and unbiased. By providing transparent explanations, AI systems can demonstrate that they are not perpetuating existing biases or discriminating against certain groups.
- Learning and Improvement: Explainability enables users to understand the limitations and biases of AI systems, allowing for continuous learning and improvement.
Challenges in Achieving Explainability
Achieving explainability in AI decision-making is not without its challenges:
- Complexity: AI systems are often complex and involve multiple algorithms, data sources, and parameters. Providing clear explanations can be challenging due to the complexity of these systems.
- Data Quality: AI systems rely on high-quality data to make accurate decisions. However, poor data quality can lead to biased or incorrect decisions, making it difficult to provide clear explanations.
- Algorithmic Transparency: Some AI algorithms, such as deep neural networks, can be difficult to interpret and explain. This lack of transparency can make it challenging to provide clear explanations for AI decisions.
Best Practices for Achieving Explainability
To achieve explainability in AI decision-making, follow these best practices:
- Use interpretable algorithms: Choose AI algorithms that are designed to provide clear explanations, such as decision trees or linear regression models.
- Provide clear documentation: Document AI systems thoroughly, including information about data sources, algorithms, and decision-making processes.
- Use visualizations: Visualize AI decision-making processes to help users understand how AI arrived at a particular decision.
- Conduct regular testing: Regularly test AI systems to ensure they are fair, unbiased, and transparent.
- Involve users: Involve users in the development and testing of AI systems to ensure they understand how AI works and can provide feedback.
The Indian Perspective
In India, the government has recognized the importance of explainability in AI decision-making. The Indian Ministry of Electronics and Information Technology (MeitY) has launched the “National Artificial Intelligence Portal” to promote the development and use of AI in India. The portal provides guidelines and resources for organizations to develop and deploy AI systems responsibly.
The “Ethical Use of AI Laws in India” article on Growth Jockey highlights the importance of explainability in AI decision-making in India. The article emphasizes the need for clear regulations and guidelines to ensure that AI systems are developed and deployed ethically. It also highlights the importance of transparency and accountability in AI decision-making.
Conclusion
Explainability is crucial for ensuring accountability, transparency, trust, fairness, and learning in AI decision-making. As AI influences more decisions in our daily lives, it’s essential to ensure that users can understand how these outputs were generated. By following best practices and involving users in the development and testing of AI systems, we can achieve explainability and promote responsible AI adoption. Transparency is not optional; it’s the foundation of responsible AI adoption.
News Source:
https://www.growthjockey.com/blogs/ethical-use-of-ai-laws-in-india