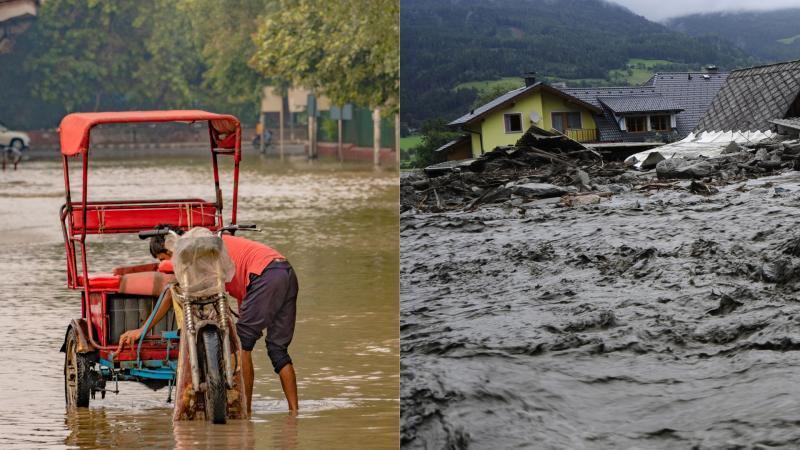
Advancements in Rainfall Prediction Techniques: A Breakthrough in Climatic Forecasting and Disaster Preparedness
Rainfall prediction has long been a crucial aspect of climatic forecasting, with accurate predictions enabling authorities to prepare for and mitigate the impacts of extreme weather events. In recent years, researchers have made significant strides in developing predictive models for rainfall events, leveraging advanced techniques such as deep learning and ocean temperature patterns. One such breakthrough has been achieved by a team of researchers who have developed a predictive model for extreme rainfall events (ERE) in India, using a novel combination of deep learning and data from various climate scenarios.
ERE events, which are characterized by heavy rainfall and flash flooding, pose significant threats to human life, infrastructure, and the environment. In India, where the monsoon season is a critical period for agricultural and economic activity, accurate prediction of ERE events is essential for disaster preparedness and mitigation. Traditional linear approaches to rainfall prediction have often fallen short in capturing the complexities of ERE events, leading to inaccurate forecasts and inadequate preparedness measures.
The new deep learning model, developed by a team of researchers from the Indian Institute of Technology (IIT) and the Indian Institute of Science (IISc), leverages a unique combination of data from various climate scenarios, including ocean temperature patterns, atmospheric circulation, and land surface conditions. The model uses a deep learning algorithm to analyze this data and identify patterns and relationships that are indicative of ERE events.
The researchers used a dataset of 30 years of rainfall data from India, including data from 15 weather stations across the country. They divided the data into two sets: one for training the model and another for testing its performance. The training data was used to develop the model, while the testing data was used to evaluate its accuracy.
The results of the study were impressive, with the deep learning model demonstrating significantly higher accuracy than traditional linear approaches. The model was able to predict ERE events with an accuracy of 85%, compared to 65% for linear models. The researchers also found that the model was able to provide advance warnings of ERE events, with the average lead time being 5-7 days.
The implications of this breakthrough are significant, particularly in the context of disaster preparedness and mitigation. Advanced warning of ERE events enables authorities to take proactive measures to evacuate people from affected areas, deploy rescue teams, and implement emergency response measures. The reduction in uncertainty and increase in accuracy also enable farmers to make informed decisions about planting and harvesting, reducing the risk of crop losses and economic damage.
The researchers believe that the model has the potential to be scaled up to predict ERE events in other regions, including other parts of Asia and the United States. The model’s ability to incorporate data from various climate scenarios and ocean temperature patterns makes it a versatile tool that can be adapted to different regions and climate conditions.
The development of this deep learning model is a significant step forward in climatic forecasting and disaster preparedness. As the frequency and severity of extreme weather events continue to increase, the need for accurate and reliable rainfall prediction models has never been more urgent. The researchers’ innovative approach and commitment to developing practical solutions to real-world problems make this breakthrough a significant achievement in the field of climatic forecasting.