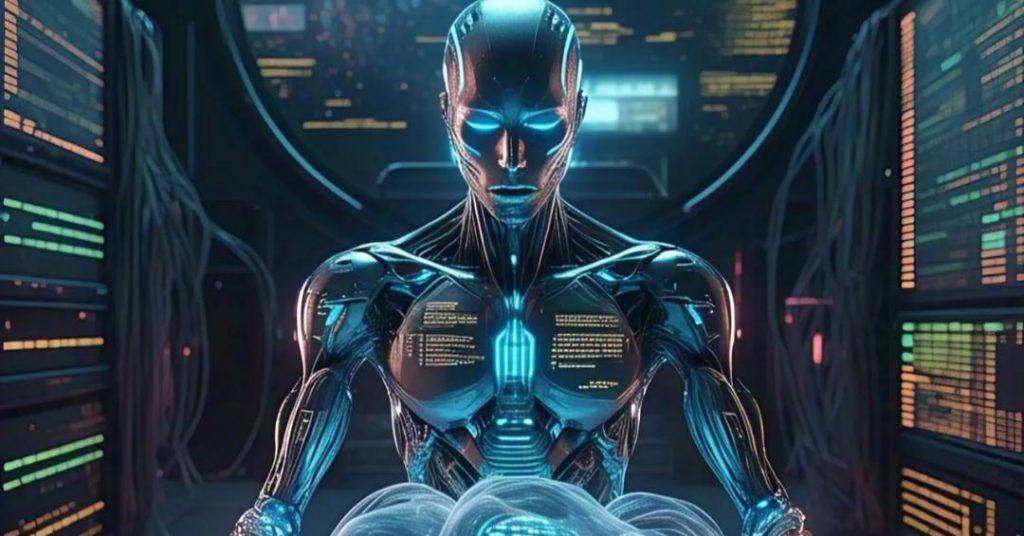
How Poor Data Management can Put AI Projects at Risk?
Artificial Intelligence (AI) has become a buzzword in the industry, with many organisations investing heavily in AI projects to gain a competitive edge. However, a recent survey by Gartner revealed a shocking statistic: 63% of organisations lack AI-ready data, putting their AI projects at risk of failure. This raises a crucial question: what’s going wrong with data management, and how can organisations overcome these challenges to ensure the success of their AI initiatives?
The survey, which polled 1,203 data management leaders in 2024, found that a significant number of organisations are failing to meet the unique requirements of AI-ready data. Traditional data management practices and poor metadata management are major culprits, hindering the adoption of AI and putting projects at risk.
So, what exactly is AI-ready data? AI algorithms require high-quality, relevant, and structured data to learn from and make accurate predictions. This data must be accessible, consistent, and scalable to support the complex calculations and processing involved in AI. Unfortunately, many organisations struggle to provide this level of data quality, leading to suboptimal AI performance and, ultimately, project failure.
One of the primary reasons for poor data management is the lack of understanding between AI-ready requirements and traditional data management practices. AI projects require a fundamentally different approach to data management, driven by the need for high-quality, real-time data and the ability to handle large volumes of data. However, many organisations are still stuck in traditional data management silos, where data is managed in a linear, batch-based manner.
Another key issue is metadata management. Metadata is the data that describes other data, such as the source, format, and quality of the data. In traditional data management, metadata is often overlooked or managed in a manual, ad-hoc manner. However, AI projects require accurate and up-to-date metadata to ensure data quality and consistency. Poor metadata management can lead to data inconsistencies, errors, and inaccuracies, which can have severe consequences for AI performance.
The consequences of poor data management can be severe. AI projects that fail to deliver expected results can lead to wasted resources, lost revenue, and damaged reputations. Moreover, the lack of AI-ready data can also hinder the ability to make informed business decisions, leading to stagnation and decreased competitiveness.
So, what can organisations do to overcome these challenges and ensure the success of their AI projects? Here are a few strategies:
- Understand AI-ready requirements: Organisations should take the time to understand the unique requirements of AI-ready data and the need for high-quality, real-time data.
- Implement metadata management: Implementing a metadata management strategy can help ensure data quality, consistency, and accuracy.
- Adopt modern data management practices: Organisations should adopt modern data management practices, such as data warehousing and cloud-based data storage, to support the complex calculations and processing involved in AI.
- Invest in data quality: Investing in data quality initiatives, such as data cleansing and data validation, can help ensure that data is accurate, complete, and consistent.
- Monitor and measure data quality: Organisations should monitor and measure data quality to ensure that it meets AI-ready requirements.
In conclusion, poor data management can put AI projects at risk of failure. Organisations must understand the unique requirements of AI-ready data and take steps to improve data quality, metadata management, and modern data management practices. By adopting these strategies, organisations can ensure the success of their AI projects and unlock the full potential of AI.