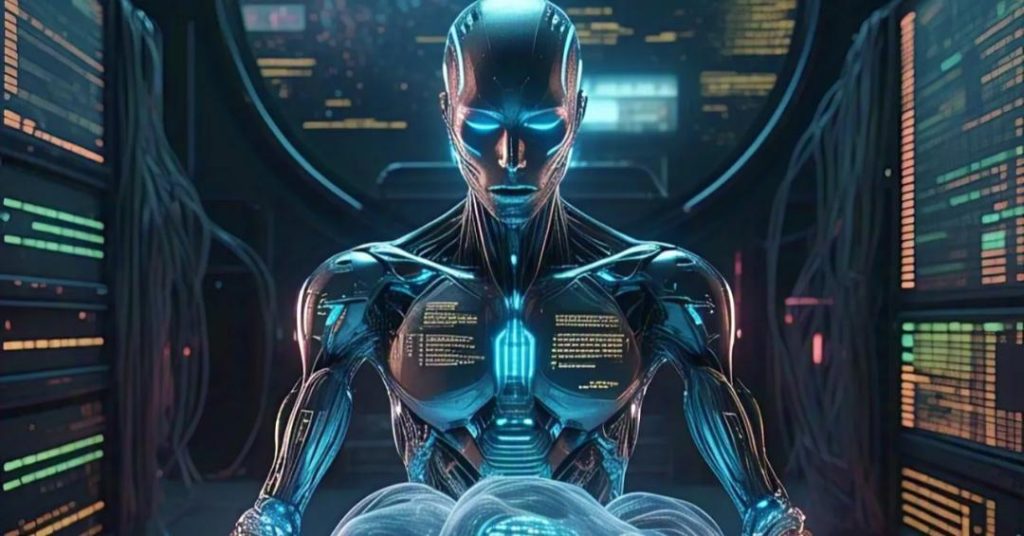
How Poor Data Management Can Put AI Projects at Risk?
The advent of Artificial Intelligence (AI) has revolutionized the way businesses operate, making it possible for organizations to make data-driven decisions, automate processes, and improve overall efficiency. However, the success of AI projects largely depends on the quality and availability of data. A recent survey by Gartner found that 63% of organizations lack AI-ready data, putting their AI projects at risk of failure.
The survey, which polled 1,203 data management leaders in 2024, revealed that organizations are struggling to manage their data effectively, leading to a significant gap between AI-ready requirements and traditional data management practices. This gap is a major obstacle to the successful implementation of AI projects, and it’s essential to understand the consequences of poor data management on AI adoption.
What is AI-Ready Data?
AI-ready data refers to high-quality, structured, and well-curated data that is specifically designed to support AI and machine learning algorithms. This type of data is essential for training AI models, as it enables them to learn from past experiences and make accurate predictions. AI-ready data is characterized by its richness, completeness, and consistency, making it possible for AI algorithms to produce accurate and reliable results.
The importance of AI-ready data cannot be overstated. AI models require large amounts of high-quality data to learn from, and poor data quality can lead to inaccurate predictions, biased results, and even model failure. In contrast, AI-ready data enables organizations to gain valuable insights from their data, make informed decisions, and drive business success.
Challenges of Poor Data Management
Poor data management is a major challenge that can hinder the success of AI projects. Some of the common challenges associated with poor data management include:
- Data Quality Issues: Poor data quality can lead to inaccurate predictions, biased results, and even model failure. AI models require high-quality data to produce accurate results, and poor data quality can undermine the entire AI project.
- Data Inconsistencies: Inconsistent data can lead to errors, inaccuracies, and biased results. AI models require consistent data to produce reliable results, and inconsistent data can undermine the entire AI project.
- Data Silos: Data silos refer to the fragmentation of data across different departments, systems, and applications. This can lead to data inconsistencies, inaccuracies, and even data loss.
- Outdated Practices: Outdated data management practices can hinder the success of AI projects. Traditional data management practices are not designed to support AI and machine learning algorithms, and they can lead to data inconsistencies, inaccuracies, and even model failure.
- Lack of Metadata Management: Metadata management refers to the process of managing data about data. Poor metadata management can lead to data inconsistencies, inaccuracies, and even data loss.
Consequences of Poor Data Management
The consequences of poor data management can be severe, and they can put AI projects at risk of failure. Some of the consequences of poor data management include:
- Failure to Achieve AI Goals: Poor data management can lead to the failure to achieve AI goals, such as improving customer service, reducing costs, and increasing efficiency.
- Inaccurate Predictions: Poor data management can lead to inaccurate predictions, biased results, and even model failure.
- Data Loss: Poor data management can lead to data loss, which can result in the loss of valuable business insights and opportunities.
- Regulatory Non-Compliance: Poor data management can lead to regulatory non-compliance, which can result in fines, penalties, and even reputational damage.
- Increased Costs: Poor data management can lead to increased costs, as organizations may need to invest in data quality improvement initiatives.
Best Practices for AI-Ready Data Management
To ensure the success of AI projects, it’s essential to adopt best practices for AI-ready data management. Some of the best practices include:
- Data Governance: Establish a data governance framework that ensures data quality, consistency, and compliance.
- Data Quality: Implement data quality initiatives to ensure high-quality data that is accurate, complete, and consistent.
- Metadata Management: Implement metadata management practices to ensure data about data is accurate, complete, and consistent.
- Data Integration: Implement data integration practices to ensure data is integrated across different systems, applications, and departments.
- Data Analytics: Implement data analytics practices to ensure data is analyzed and insights are gained to inform business decisions.
Conclusion
In conclusion, poor data management can put AI projects at risk of failure. AI-ready data is essential for the success of AI projects, and poor data management can lead to data inconsistencies, inaccuracies, and even model failure. To ensure the success of AI projects, it’s essential to adopt best practices for AI-ready data management, such as data governance, data quality, metadata management, data integration, and data analytics. By adopting these best practices, organizations can ensure the quality and availability of AI-ready data, and drive business success through AI adoption.