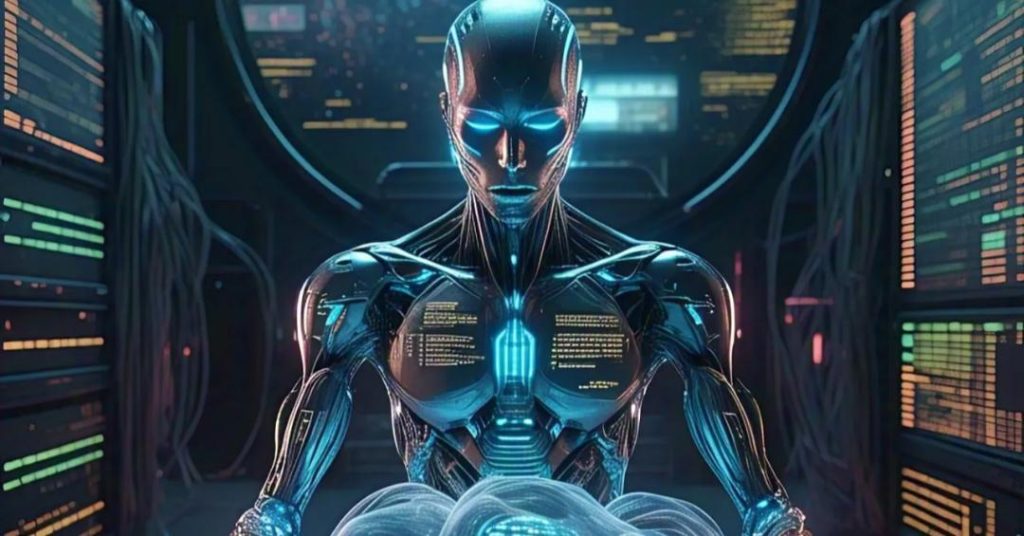
How Poor Data Management can Put AI Projects at Risk?
The integration of Artificial Intelligence (AI) in various industries has become a hot topic in recent years. As AI technology continues to advance, businesses are increasingly relying on it to gain a competitive edge, improve efficiency, and make informed decisions. However, a recent survey by Gartner revealed a shocking truth – 63% of organisations lack AI-ready data, putting their AI projects at risk of failure.
The survey, which polled 1,203 data management leaders in 2024, found that organisations that fail to understand the differences between AI-ready data requirements and traditional data management practices are more likely to struggle with the success of their AI projects.
So, what exactly is AI-ready data, and why is it so crucial for AI projects? AI-ready data refers to data that is clean, accurate, complete, and relevant, and is specifically designed to support AI and machine learning algorithms. This type of data is essential for training AI models, as it enables them to learn from patterns and make accurate predictions.
The problem lies in the fact that many organisations are still using outdated data management practices, which are not designed to handle the unique requirements of AI-ready data. This can lead to a range of issues, including data inconsistencies, inaccuracies, and incomplete data, which can severely impact the performance of AI models.
Metadata management is another critical aspect of AI data management that is often overlooked. Metadata refers to the data that provides context and information about other data, such as data quality, data lineage, and data usage. Without proper metadata management, it can be difficult to track the origin and quality of data, which can lead to issues such as data corruption and poor data quality.
In addition to metadata management, organisations must also ensure that their data is properly curated and integrated. AI models require a large amount of data to train and validate, and this data must be integrated from multiple sources and formats. Without proper data curation and integration, AI models may not have access to the data they need to learn and improve.
So, what can organisations do to avoid the pitfalls of poor data management and ensure the success of their AI projects? Here are a few strategies that can help:
- Understand AI-ready data requirements: Organisations must understand the specific requirements of AI-ready data and design their data management practices accordingly.
- Implement metadata management: Implementing a metadata management strategy can help organisations track the origin and quality of data, ensuring that AI models have access to high-quality data.
- Curate and integrate data: Organisations must curate and integrate data from multiple sources and formats to ensure that AI models have access to the data they need to learn and improve.
- Use data governance: Establishing a data governance strategy can help organisations ensure that data is consistent, accurate, and complete, and that AI models are trained and validated using high-quality data.
- Monitor and evaluate AI projects: Organisations must regularly monitor and evaluate the performance of their AI projects to identify areas for improvement and ensure that they are meeting their goals.
In conclusion, poor data management can put AI projects at risk by leading to issues such as data inconsistencies, inaccuracies, and incomplete data. To avoid these pitfalls, organisations must understand AI-ready data requirements, implement metadata management, curate and integrate data, use data governance, and regularly monitor and evaluate AI projects. By following these strategies, organisations can ensure the success of their AI projects and reap the benefits of AI technology.