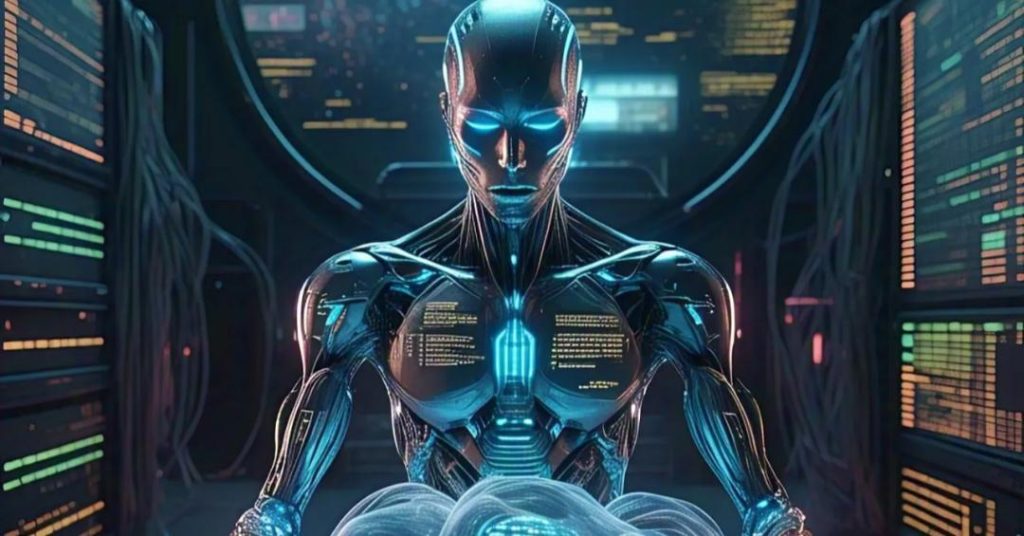
How Poor Data Management Can Put AI Projects at Risk?
Artificial Intelligence (AI) has revolutionized various industries by improving efficiency, accuracy, and decision-making capabilities. However, AI projects are only as good as the data they are built upon. A recent survey by Gartner revealed that 63% of organizations lack AI-ready data, which puts their AI projects at risk of failure. This raises a critical question: what is causing this data management challenge, and how can organizations overcome it?
The AI Data Dilemma
The Gartner survey, which polled 1,203 data management leaders in 2024, found that organizations failing to recognize the differences between AI-ready requirements and traditional data management are endangering the success of their AI efforts. This is a significant concern, as AI projects rely heavily on high-quality, relevant, and accessible data to deliver accurate results.
The survey highlights the importance of metadata management in AI projects. Metadata refers to the data that describes other data, such as its origin, format, and relationships. In traditional data management, metadata is often overlooked or underutilized. However, in AI projects, metadata is crucial for understanding the context, relevance, and quality of the data.
Challenges in AI Data Management
Poor metadata management is just one of the challenges that organizations face in AI data management. Other challenges include:
- Data Quality: AI algorithms require high-quality data to deliver accurate results. However, poor data quality, including inaccuracies, inconsistencies, and missing values, can lead to incorrect predictions and decisions.
- Data Scalability: AI projects often involve large datasets that require significant computing resources. However, traditional data management systems may not be able to handle the scale and complexity of AI data.
- Data Integration: AI projects often require integrating data from multiple sources, including internal and external data sources. However, integrating data from different sources can be challenging, especially when dealing with incompatible formats and data structures.
- Data Governance: AI projects require strong data governance to ensure that data is secure, compliant, and accessible. However, traditional data governance practices may not be adequate for AI projects, which require more flexible and adaptive governance approaches.
Consequences of Poor Data Management
The consequences of poor data management in AI projects can be severe. Some of the consequences include:
- Project Failure: Poor data management can lead to project delays, budget overruns, and ultimately, project failure.
- Inaccurate Results: Poor data quality can lead to inaccurate predictions and decisions, which can have serious consequences, such as financial losses or reputational damage.
- Security Risks: Poor data governance can lead to security breaches, which can expose sensitive information and compromise organizational integrity.
- Lack of Trust: Poor data management can erode trust in AI projects, which can lead to reduced adoption and reduced benefits.
Best Practices for AI Data Management
To overcome the challenges and consequences of poor data management, organizations can adopt the following best practices:
- Develop a Data Strategy: Organizations should develop a data strategy that aligns with their AI goals and objectives.
- Invest in Metadata Management: Organizations should invest in metadata management to ensure that data is accurately described and contextualized.
- Implement Data Quality Controls: Organizations should implement data quality controls to ensure that data is accurate, complete, and consistent.
- Develop Data Integration Strategies: Organizations should develop data integration strategies to integrate data from multiple sources and formats.
- Establish Data Governance: Organizations should establish data governance to ensure that data is secure, compliant, and accessible.
Conclusion
Poor data management is a significant risk to AI projects, and organizations must take proactive steps to overcome this challenge. By developing a data strategy, investing in metadata management, implementing data quality controls, developing data integration strategies, and establishing data governance, organizations can ensure the success of their AI projects and unlock the full potential of AI.
Reference: