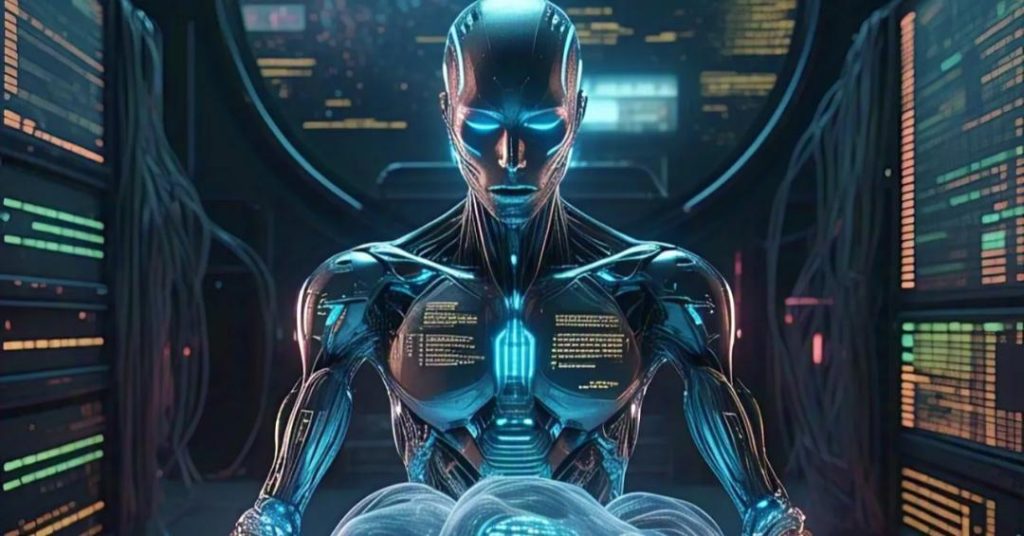
How Poor Data Management can Put AI Projects at Risk?
The advent of Artificial Intelligence (AI) has revolutionized the way businesses operate, making it possible to automate tasks, gain insights, and make data-driven decisions. However, the success of AI projects heavily relies on the quality and availability of data. A recent survey by Gartner reveals that 63% of organizations lack AI-ready data, putting their AI projects at risk of failure. In this blog post, we’ll delve into the importance of data management in AI projects and why poor data management can lead to project failures.
The AI Data Dilemma
The Gartner survey, which polled 1,203 data management leaders in 2024, found that organizations are struggling to manage their data effectively, particularly when it comes to AI-ready data. The survey highlights the need for organizations to understand the differences between AI-ready requirements and traditional data management practices. Unfortunately, many organizations are failing to make this distinction, which is putting their AI projects at risk.
AI-ready data is critical because it enables organizations to train and deploy AI models accurately. However, poor data management practices can lead to data inconsistencies, inaccuracies, and inefficiencies, which can hinder the success of AI projects. The survey found that organizations that fail to recognize the importance of AI-ready data are more likely to experience project delays, cost overruns, and ultimately, project failures.
The Role of Metadata Management
Metadata management plays a crucial role in AI projects. Metadata refers to the data that describes the structure, content, and context of other data. In the context of AI projects, metadata management is essential for ensuring data quality, consistency, and reliability. Poor metadata management can lead to data inconsistencies, making it challenging for AI models to learn and make accurate predictions.
Metadata management involves the creation, maintenance, and use of metadata to support the lifecycle of data. This includes data discovery, data profiling, data quality, and data governance. Effective metadata management ensures that data is accurately described, easily accessible, and efficiently utilized. In AI projects, metadata management is critical for ensuring that data is properly labeled, categorized, and prepared for training AI models.
Outdated Data Management Practices
The Gartner survey also found that many organizations are still using outdated data management practices, which are hindering their ability to adopt AI. These practices include:
- Data silos: Many organizations still maintain data silos, where data is stored in isolated systems, making it difficult to access and integrate data from different sources.
- Lack of data standardization: Organizations often lack standardized data formats, making it challenging to integrate data from different sources and systems.
3.Inadequate data governance: Many organizations lack effective data governance practices, which can lead to data inconsistencies, inaccuracies, and inefficiencies.
4.Insufficient data quality: Poor data quality can lead to inaccurate predictions, poor decision-making, and ultimately, project failures.
Best Practices for Data Management in AI Projects
To avoid the pitfalls of poor data management, organizations should adopt the following best practices:
1.Implement a data governance framework: Develop a data governance framework that outlines roles, responsibilities, and policies for data management.
2.Use metadata management tools: Utilize metadata management tools to create, maintain, and use metadata to support the lifecycle of data.
3.Standardize data formats: Standardize data formats to ensure consistency and accuracy across different systems and sources.
4.Integrate data from different sources: Integrate data from different sources to ensure a single source of truth and improve data accuracy.
5.Monitor data quality: Monitor data quality regularly to identify and address data inconsistencies and inaccuracies.
Conclusion
In conclusion, poor data management can put AI projects at risk of failure. The Gartner survey highlights the importance of AI-ready data and the need for organizations to understand the differences between AI-ready requirements and traditional data management practices. By adopting best practices for data management, such as implementing a data governance framework, using metadata management tools, standardizing data formats, integrating data from different sources, and monitoring data quality, organizations can ensure the success of their AI projects.
References: