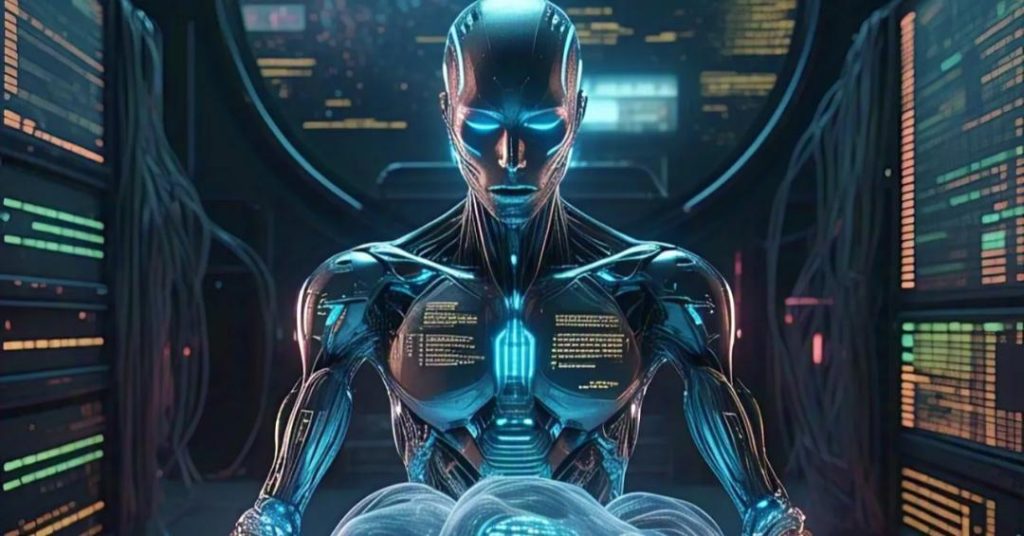
How Poor Data Management Can Put AI Projects at Risk?
The resurgence of Artificial Intelligence (AI) has led to a surge in its adoption across various industries. However, despite the hype surrounding AI, many organizations are struggling to make the most of this technology. A recent survey by Gartner revealed that 63% of organizations lack AI-ready data, putting their AI projects at risk of failure.
The survey, which involved 1,203 data management leaders in 2024, found that organizations failing to realize the differences between AI-ready requirements and traditional data management practices are endangering the success of their AI efforts. This is a wake-up call for organizations that are investing heavily in AI but are not paying attention to the quality and management of their data.
So, what are the consequences of poor data management for AI projects? In this blog post, we will explore the risks and challenges associated with poor data management and provide insights on how to overcome these hurdles.
The Challenges of AI-Ready Data
AI-ready data is more than just having a large amount of data. It requires data that is high-quality, relevant, and easily accessible. AI algorithms are designed to work with large datasets, but they are only as good as the data they are trained on. Poor-quality data can lead to inaccurate insights, biased results, and ultimately, the failure of AI projects.
The challenges of AI-ready data are numerous. Here are a few:
- Data Quality: AI algorithms are designed to work with high-quality data. However, many organizations struggle to ensure the quality of their data. Poor data quality can lead to inaccurate insights, biased results, and ultimately, the failure of AI projects.
- Data Completeness: AI algorithms require complete and consistent data to produce accurate results. However, many organizations struggle to ensure that their data is complete and consistent.
- Data Timeliness: AI algorithms require timely data to produce accurate results. However, many organizations struggle to ensure that their data is up-to-date and relevant.
- Data Integration: AI algorithms require data from multiple sources to produce accurate results. However, many organizations struggle to integrate data from multiple sources.
The Consequences of Poor Data Management
Poor data management can have severe consequences for AI projects. Here are a few:
- Inaccurate Insights: Poor data quality can lead to inaccurate insights, which can be costly and time-consuming to correct.
- Biased Results: Poor data quality can lead to biased results, which can have serious consequences, such as unfair decision-making and discriminatory outcomes.
- Delayed Projects: Poor data management can lead to delayed projects, which can be costly and time-consuming to recover from.
- Failure to Meet Business Objectives: Poor data management can lead to failure to meet business objectives, which can have serious consequences, such as loss of revenue and reputation.
The Importance of Metadata Management
Metadata management is critical for AI projects. Metadata is the data that describes other data, such as the source, format, and quality of the data. Metadata management involves creating, managing, and maintaining metadata to ensure that it is accurate, complete, and consistent.
The importance of metadata management for AI projects cannot be overstated. Here are a few reasons why:
- Data Discovery: Metadata management enables data discovery, which is critical for AI projects. Data discovery involves identifying and cataloging data assets, which enables organizations to identify and utilize the data they need.
- Data Integration: Metadata management enables data integration, which is critical for AI projects. Data integration involves combining data from multiple sources, which enables organizations to produce accurate and comprehensive insights.
- Data Quality: Metadata management enables data quality, which is critical for AI projects. Metadata management involves creating, managing, and maintaining metadata to ensure that it is accurate, complete, and consistent.
Best Practices for AI-Ready Data
So, how can organizations ensure that their data is AI-ready? Here are a few best practices:
- Data Governance: Establish a data governance program to ensure that data is managed consistently across the organization.
- Data Quality: Ensure that data is high-quality, relevant, and easily accessible.
- Data Completeness: Ensure that data is complete and consistent.
- Data Timeliness: Ensure that data is up-to-date and relevant.
- Data Integration: Ensure that data is integrated from multiple sources.
- Metadata Management: Ensure that metadata is accurate, complete, and consistent.
- Data Cataloging: Create a data catalog to identify and catalog data assets.
- Data Visualization: Use data visualization tools to enable data discovery and exploration.
Conclusion
Poor data management can put AI projects at risk of failure. AI-ready data is more than just having a large amount of data. It requires data that is high-quality, relevant, and easily accessible. Organizations must ensure that their data is AI-ready by establishing a data governance program, ensuring data quality, completeness, and timeliness, and integrating data from multiple sources. By following these best practices, organizations can ensure that their data is AI-ready and that their AI projects are successful.
News Source