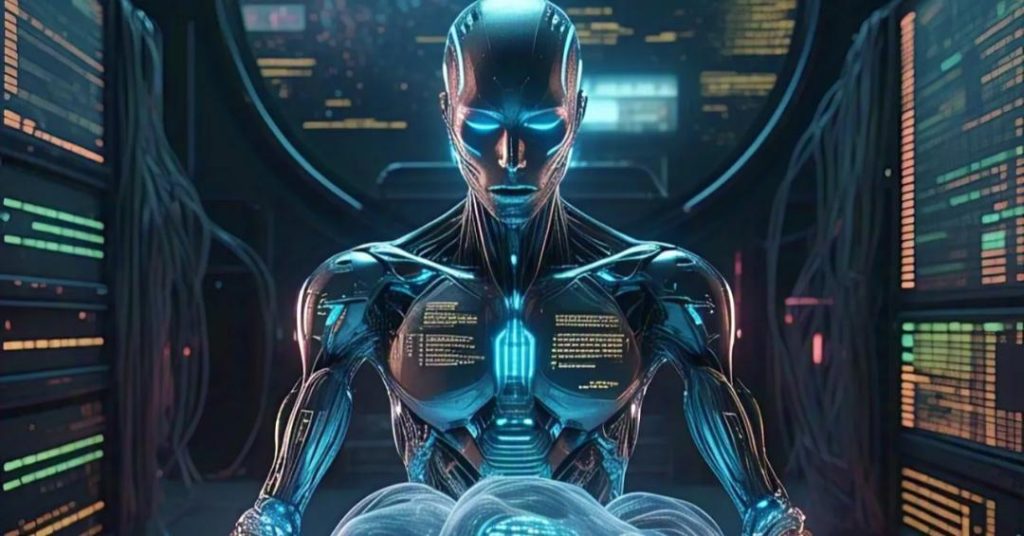
How Poor Data Management Can Put AI Projects at Risk
The adoption of Artificial Intelligence (AI) has been gaining momentum in recent years, with many organizations investing heavily in AI projects to drive innovation, improve efficiency, and gain a competitive edge. However, a recent survey by Gartner has revealed a startling statistic: 63% of organizations lack AI-ready data, putting their AI projects at risk of failure.
The survey, which polled 1,203 data management leaders in 2024, found that organizations are struggling to manage their data effectively, which is hindering the adoption of AI. The survey also revealed that many organizations are failing to realize the differences between AI-ready requirements and traditional data management practices.
So, what exactly is AI-ready data, and why is it so crucial for AI projects? AI-ready data refers to high-quality, organized, and easily accessible data that can be used to train and deploy AI models. This type of data is typically characterized by its completeness, consistency, and relevance to the AI application.
In contrast, traditional data management practices focus on storing and retrieving data in a way that is efficient for human consumption. These practices do not take into account the unique requirements of AI, such as the need for large amounts of data, high-quality data, and fast data processing.
The consequences of poor data management can be severe. Without AI-ready data, AI projects are likely to fail or struggle to deliver the expected results. This can lead to wasted resources, missed opportunities, and a loss of confidence in AI.
So, what are the common pitfalls of poor data management that can put AI projects at risk? Here are a few examples:
-
Poor Metadata Management: Metadata refers to the data that describes other data, such as the date it was created, who created it, and what it is used for. Poor metadata management can lead to data being mislabeled, misplaced, or lost, making it difficult to find and use the data effectively.
-
Outdated Data Storage and Retrieval: Many organizations still use outdated data storage and retrieval systems, which can lead to slow data processing, data loss, and data corruption. These systems are not designed to handle the large amounts of data required for AI applications.
-
Data Inconsistency and Incompleteness: AI algorithms require high-quality data that is consistent and complete. Poor data management can lead to data inconsistencies and incompleteness, which can compromise the accuracy and reliability of AI models.
-
Lack of Data Governance: Data governance refers to the policies, procedures, and standards that govern the management of data. Without effective data governance, data can become disorganized, inconsistent, and difficult to use.
-
Inadequate Data Quality: AI algorithms require high-quality data that is accurate, complete, and relevant. Poor data quality can lead to AI models that are biased, inaccurate, or ineffective.
To avoid these pitfalls and ensure the success of AI projects, organizations need to prioritize data management. Here are a few strategies that can help:
-
Invest in Data Management Tools: Organizations should invest in data management tools that can help them manage their data effectively. These tools can include data integration platforms, data governance software, and data quality tools.
-
Implement Data Governance: Organizations should establish effective data governance policies, procedures, and standards to ensure that data is managed consistently and reliably.
-
Focus on Data Quality: Organizations should prioritize data quality by ensuring that data is accurate, complete, and relevant. This can be achieved by implementing data quality tools and processes.
-
Develop AI-Ready Data: Organizations should develop AI-ready data by ensuring that data is organized, consistent, and easily accessible. This can be achieved by implementing data integration platforms and data governance software.
-
Train Data Management Teams: Organizations should train their data management teams to understand the unique requirements of AI and how to manage data effectively for AI applications.
In conclusion, poor data management can put AI projects at risk by hindering the adoption of AI and compromising the accuracy and reliability of AI models. To avoid these pitfalls, organizations need to prioritize data management by investing in data management tools, implementing data governance, focusing on data quality, developing AI-ready data, and training data management teams. By doing so, organizations can ensure the success of their AI projects and reap the benefits of AI adoption.