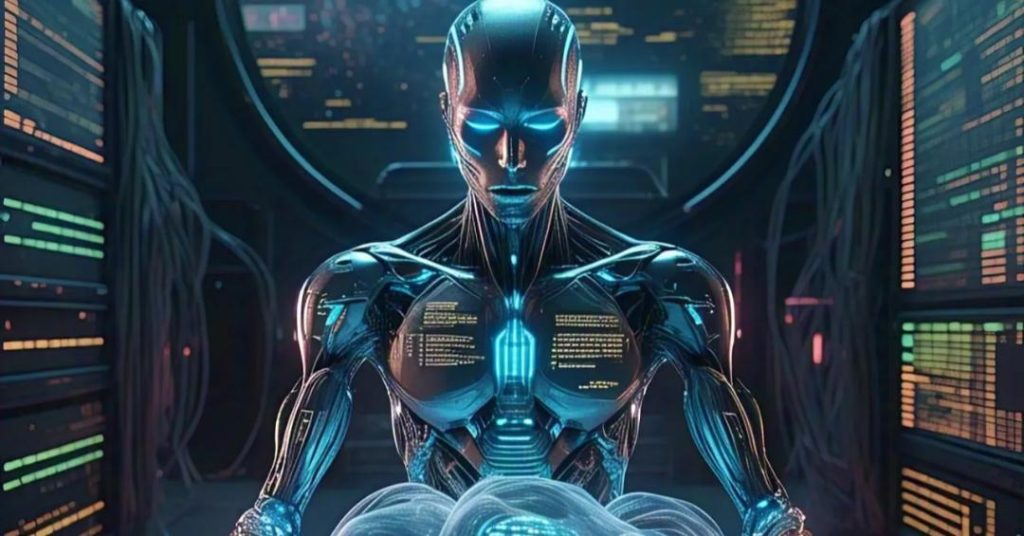
How Poor Data Management Can Put AI Projects at Risk?
Artificial Intelligence (AI) has revolutionized the way businesses operate, and its adoption is expected to continue growing in the coming years. However, the success of AI projects depends heavily on the quality and availability of data. A recent survey by Gartner revealed that a staggering 63% of organizations lack AI-ready data, putting their AI projects at risk of failure. In this blog post, we’ll explore how poor data management can hinder AI adoption and what measures organizations can take to overcome these challenges.
A Gartner survey of 1,203 data management leaders in 2024 found that many organizations are failing to realize the differences between AI-ready data requirements and traditional data management practices. This lack of understanding is leading to a significant risk of AI project failure. The survey highlights the importance of having a clear understanding of the AI-ready data requirements and ensuring that data management practices are aligned with these requirements.
Poor metadata management is another significant challenge that organizations face when it comes to AI adoption. Metadata is critical for AI algorithms to understand the context, relationships, and meaning of the data. However, many organizations lack a robust metadata management strategy, making it difficult to extract insights from their data. Without accurate and complete metadata, AI models may produce inaccurate or biased results, leading to poor decision-making.
Outdated data management practices are also hindering AI adoption. Many organizations are still relying on traditional data management approaches, such as data warehousing and ETL (Extract, Transform, Load) processes. These approaches are not designed to handle the complexity and volume of data required for AI applications. Moreover, they are often manual and time-consuming, making it difficult to scale and maintain large data sets.
The lack of AI-ready data is not just a technical challenge; it also has significant business implications. Organizations that fail to adopt AI-ready data practices may struggle to stay competitive in their industries. AI is transforming the way businesses operate, and organizations that fail to adopt AI-ready data practices may be left behind.
So, what can organizations do to overcome these challenges and ensure the success of their AI projects?
Firstly, organizations need to prioritize data quality and ensure that their data is accurate, complete, and relevant. This requires a robust data governance strategy that ensures data is properly managed and maintained throughout its lifecycle.
Secondly, organizations need to invest in metadata management. This includes developing a metadata strategy, creating metadata repositories, and ensuring that metadata is accurate and complete. Metadata management is critical for AI algorithms to understand the context and relationships of the data.
Thirdly, organizations need to adopt modern data management practices that are designed for AI applications. This includes adopting cloud-based data platforms, using machine learning-based data processing, and leveraging open-source data technologies.
Fourthly, organizations need to invest in data engineering and analytics skills. Data engineering and analytics are critical for building and maintaining AI-ready data pipelines. Without these skills, organizations may struggle to extract insights from their data.
Finally, organizations need to prioritize data storytelling and ensure that their data is presented in a way that is easy to understand and interpret. AI models produce complex insights, and organizations need to be able to communicate these insights effectively to stakeholders.
In conclusion, poor data management is a significant risk to AI project success. Organizations that fail to prioritize data quality, metadata management, and modern data management practices may struggle to adopt AI and stay competitive in their industries. By prioritizing these areas, organizations can ensure the success of their AI projects and unlock the full potential of AI.