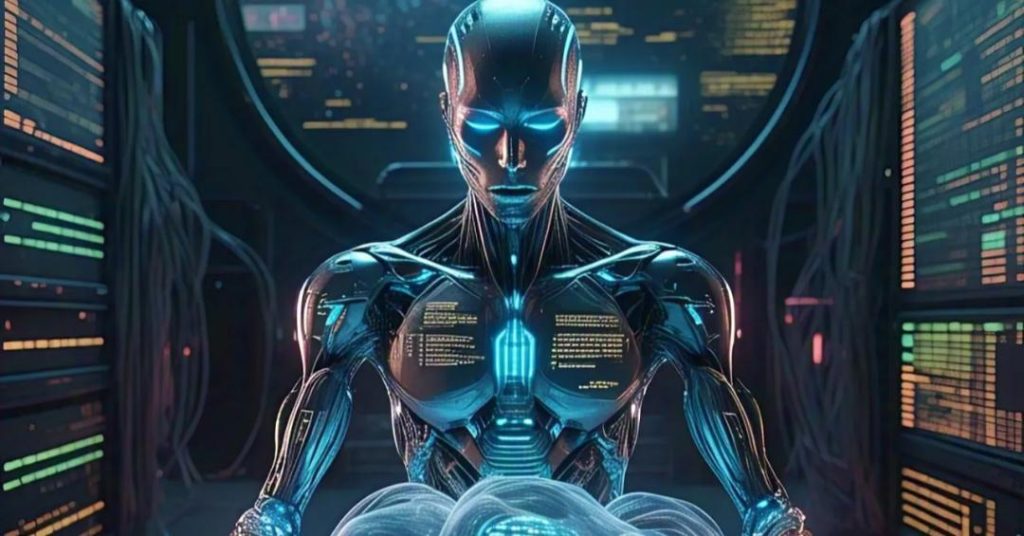
How Poor Data Management Can Put AI Projects at Risk?
The growing importance of Artificial Intelligence (AI) in today’s business landscape is undeniable. From automating mundane tasks to making informed decisions, AI has the potential to revolutionize the way organizations operate. However, a recent survey by Gartner has raised concerns about the potential risks that AI projects face due to poor data management. According to the survey, 63% of organizations lack AI-ready data, putting their AI projects at risk of failure.
In this blog post, we will delve into the world of AI and data management, exploring the reasons behind this alarming statistic and the consequences of poor data management on AI projects.
The AI-Data Dilemma
The Gartner survey, which polled 1,203 data management leaders in 2024, found that many organizations are failing to realize the differences between AI-ready data and traditional data management. This lack of understanding is leading to the creation of AI projects that are not designed to handle the complexities of AI-ready data.
AI-ready data is characterized by its ability to be used in machine learning models, which require large amounts of high-quality data to train and test algorithms. This type of data is typically characterized by its complexity, velocity, and variety. In contrast, traditional data management practices focus on storing and retrieving data in a structured format, which is not suitable for AI applications.
Consequences of Poor Data Management
The consequences of poor data management on AI projects are far-reaching and can lead to a range of negative outcomes. Some of the most common consequences include:
- Delayed Project Timelines: Poor data management can lead to delays in AI project timelines, as teams struggle to collect and process the required data.
- Higher Costs: The lack of AI-ready data can result in higher costs, as organizations may need to invest in new data management systems or hire additional personnel to collect and process data.
- Inaccurate Results: Poor data quality can lead to inaccurate results, which can have serious consequences in industries such as healthcare and finance.
- Failed Projects: In extreme cases, poor data management can lead to the failure of AI projects, resulting in significant financial and reputational losses.
Causes of Poor Data Management
So, what are the causes of poor data management that are putting AI projects at risk? Some of the most common causes include:
- Lack of Understanding: Many organizations lack a deep understanding of the complexities of AI-ready data and the requirements for collecting and processing it.
- Outdated Practices: Traditional data management practices are often not designed to handle the complexities of AI-ready data, leading to a lack of preparedness for AI projects.
- Metadata Management: Poor metadata management is a common issue in many organizations, making it difficult to track and manage data effectively.
- Data Quality: Poor data quality is a major issue in many organizations, resulting in inaccurate and incomplete data that is not suitable for AI applications.
Best Practices for AI-Ready Data Management
So, what can organizations do to ensure that they are managing their data effectively for AI projects? Some of the best practices include:
- Develop a Data Strategy: Organizations should develop a data strategy that outlines the requirements for collecting, processing, and storing AI-ready data.
- Use AI-Ready Data Platforms: Organizations should use AI-ready data platforms that are designed to handle the complexities of AI-ready data.
- Invest in Metadata Management: Organizations should invest in metadata management tools and techniques to track and manage data effectively.
- Focus on Data Quality: Organizations should prioritize data quality, ensuring that data is accurate, complete, and relevant for AI applications.
Conclusion
In conclusion, poor data management is a significant risk to AI projects, with 63% of organizations lacking AI-ready data. The consequences of poor data management can be severe, including delayed project timelines, higher costs, inaccurate results, and failed projects.
To avoid these risks, organizations must develop a deep understanding of the complexities of AI-ready data and implement best practices for data management. By investing in AI-ready data platforms, metadata management tools, and data quality initiatives, organizations can ensure that their AI projects are successful and deliver the expected benefits.