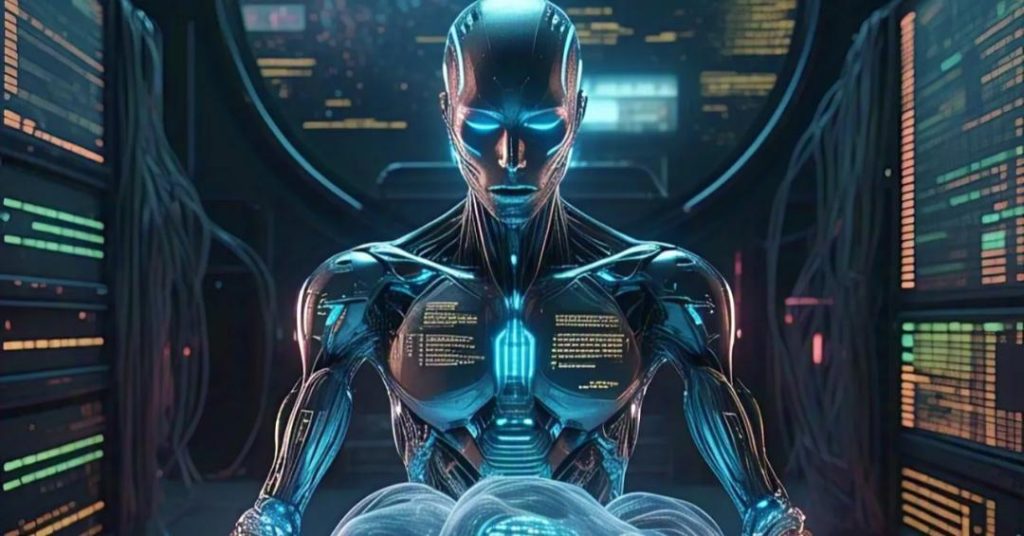
How Poor Data Management Can Put AI Projects at Risk?
The advent of Artificial Intelligence (AI) has revolutionized the way businesses operate, making it possible to automate tasks, gain insights, and make data-driven decisions. However, the success of AI projects heavily relies on the quality of data used to train and test these systems. A recent survey by Gartner revealed that a staggering 63% of organisations lack AI-ready data, putting their AI projects at risk of failure.
The survey, which polled 1,203 data management leaders in 2024, found that organisations failing to realize the differences between AI-ready requirements and traditional data management are endangering the success of their efforts. The findings highlight the critical importance of proper data management in AI projects, emphasizing the need for organisations to adopt new strategies and best practices to ensure data quality and availability.
The Challenges of AI-Ready Data
AI-ready data is not just about having large amounts of data, but rather about having high-quality data that is relevant, accurate, and suitable for AI applications. The challenges of obtaining AI-ready data are numerous, and include:
- Data Quality: AI systems are only as good as the data they are trained on. Poor data quality, including inaccuracies, inconsistencies, and missing values, can lead to biased or incorrect results.
- Data Volume: AI models require large amounts of data to learn and improve. Organisations may struggle to collect and process the required amounts of data, particularly in domains where data is scarce or difficult to obtain.
- Data Variety: AI models require diverse and varied data to learn and generalize. Organisations may struggle to collect and integrate data from different sources, formats, and domains.
- Data Velocity: AI models require real-time data to learn and respond to changing conditions. Organisations may struggle to handle the high velocity of data streaming in from various sources.
The Consequences of Poor Data Management
The consequences of poor data management in AI projects can be far-reaching and devastating. These include:
- Failure to Achieve AI Objectives: Poor data management can lead to AI models that fail to achieve their objectives, resulting in wasted resources and lost opportunities.
- Biased or Incorrect Results: Poor data quality can lead to biased or incorrect results, which can have serious consequences in domains such as healthcare, finance, and law enforcement.
- Loss of Trust: Poor data management can erode trust in AI systems and organisations, leading to a loss of confidence and credibility.
- Increased Costs: Poor data management can lead to increased costs, as organisations may need to re-collect, re-process, or re-train their AI models.
The Role of Metadata Management
Metadata management plays a critical role in AI projects, as it provides the necessary context and information about the data used to train and test AI models. Poor metadata management can lead to:
- Data Silos: Poor metadata management can lead to data silos, where data is isolated and not accessible to other parts of the organisation.
- Data Inconsistencies: Poor metadata management can lead to data inconsistencies, where data is inconsistent or incomplete.
- Data Quality Issues: Poor metadata management can lead to data quality issues, where data is inaccurate, outdated, or incomplete.
Best Practices for AI-Ready Data Management
To overcome the challenges of AI-ready data management, organisations should adopt the following best practices:
- Develop a Data Strategy: Develop a data strategy that aligns with AI objectives and requirements.
- Invest in Data Quality: Invest in data quality initiatives, including data cleansing, data integration, and data validation.
- Implement Metadata Management: Implement metadata management solutions to provide context and information about the data used to train and test AI models.
- Use Data Catalogs: Use data catalogs to provide a single source of truth for data metadata and to enable data discovery and exploration.
- Invest in Data Science: Invest in data science initiatives, including data visualization, data storytelling, and data analytics.
Conclusion
The success of AI projects relies heavily on the quality of data used to train and test these systems. Poor data management can put AI projects at risk of failure, leading to wasted resources, lost opportunities, and erosion of trust. By adopting best practices for AI-ready data management, organisations can ensure that their AI projects are successful and achieve their objectives.