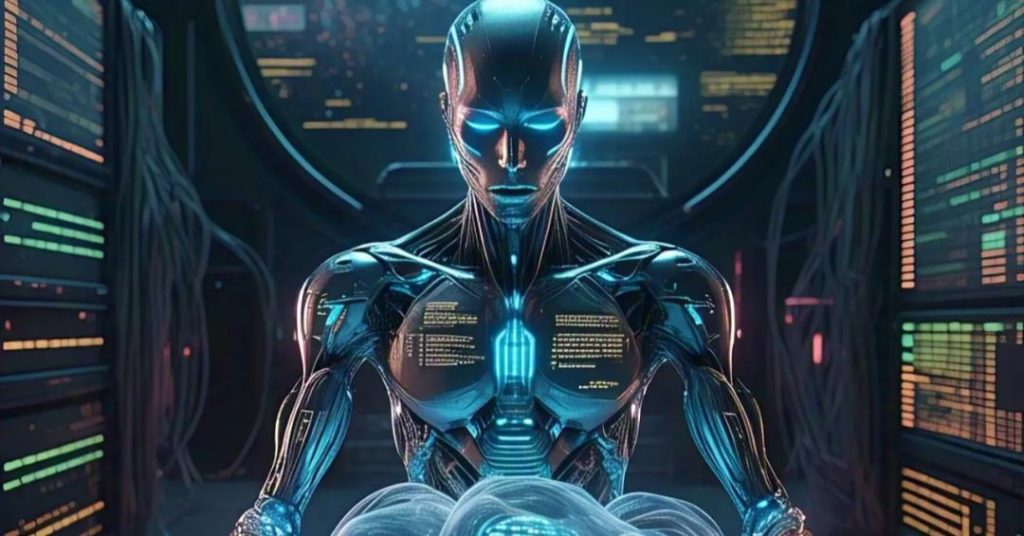
How Poor Data Management Can Put AI Projects at Risk?
The adoption of Artificial Intelligence (AI) has become a key strategy for businesses to stay ahead in today’s competitive landscape. However, a recent survey by Gartner has revealed a shocking truth – 63% of organizations lack AI-ready data, putting their AI projects at risk of failure. This staggering statistic highlights the crucial role that data management plays in the success of AI initiatives.
The survey, which polled 1,203 data management leaders in 2024, found that a significant number of organizations are failing to realize the differences between AI-ready requirements and traditional data management practices. This lack of understanding is resulting in poor data management, which is, in turn, hindering the adoption of AI.
So, what exactly is AI-ready data, and why is it so critical to the success of AI projects? AI-ready data refers to high-quality, structured, and standardized data that is specifically designed to support the development and deployment of AI models. This type of data is essential because AI algorithms require large amounts of data to learn and improve, and poor-quality data can lead to inaccurate predictions, biased results, and a lack of trust in the AI system.
On the other hand, traditional data management practices focus on storing and retrieving data efficiently, rather than preparing it for AI applications. This approach may have been sufficient for traditional business intelligence and analytics, but it is no longer sufficient in today’s AI-driven landscape.
So, what are the consequences of poor data management for AI projects? The risks are numerous:
- Inaccurate predictions: Poor-quality data can lead to inaccurate predictions, which can result in bad business decisions and a lack of trust in the AI system.
- Biased results: AI models that are trained on biased or incomplete data can produce biased results, which can perpetuate inequality and discrimination.
- Delayed project timelines: Poor data management can lead to delays in project timelines, as data quality issues need to be addressed before AI models can be deployed.
- Higher costs: Poor data management can result in higher costs, as organizations may need to invest in additional data cleaning and processing tools and services.
- Lack of trust: Poor data management can erode trust in the AI system, as users may not have confidence in the accuracy and reliability of the results.
So, what can organizations do to ensure that their data management practices are AI-ready? Here are some best practices to follow:
- Develop a data strategy: Organizations should develop a data strategy that takes into account the specific requirements of AI projects.
- Use metadata management: Metadata management is critical for AI-ready data, as it allows organizations to track and manage the quality and accuracy of their data.
- Implement data quality checks: Organizations should implement data quality checks to ensure that their data is accurate, complete, and consistent.
- Use data governance: Data governance is essential for ensuring that data is managed consistently and in accordance with organizational policies and procedures.
- Invest in data storage and processing: Organizations should invest in data storage and processing technologies that are designed to support the demands of AI applications.
In conclusion, poor data management is a significant risk to the success of AI projects. Organizations that fail to develop AI-ready data management practices will struggle to realize the benefits of AI and may even see their projects fail. By developing a data strategy, using metadata management, implementing data quality checks, using data governance, and investing in data storage and processing, organizations can ensure that their data management practices are AI-ready and support the successful adoption of AI.
News Source:
https://www.dqindia.com/opinion/the-ai-data-dilemma-why-poor-data-management-puts-ai-projects-at-risk-8888254