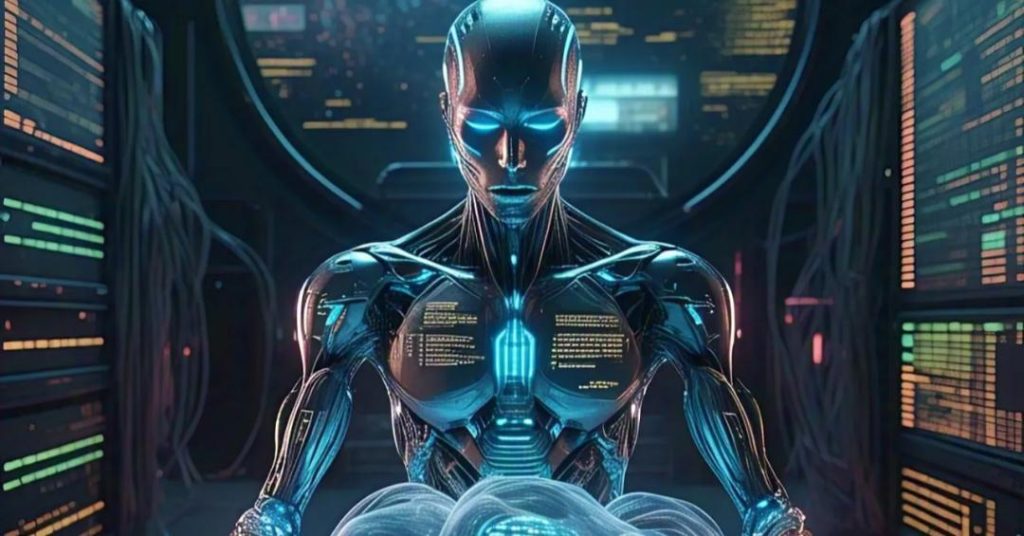
How poor data management can put AI projects at risk?
Artificial intelligence (AI) has been touted as a game-changer for businesses, with the potential to drive innovation, increase efficiency, and improve decision-making. However, a recent survey by Gartner has revealed that poor data management practices are putting AI projects at risk, with a staggering 63% of organizations lacking AI-ready data. This lack of preparedness is not only hindering AI adoption but also threatening the success of AI initiatives.
The survey, which polled 1,203 data management leaders in 2024, found that organizations are struggling to understand the differences between AI-ready data requirements and traditional data management practices. As a result, many AI projects are being delayed, scaled back, or even abandoned due to inadequate data management.
So, what is going wrong? And more importantly, how can organizations overcome these challenges to ensure the success of their AI projects?
The AI data dilemma
The Gartner survey highlights the growing concern that many organizations are facing when it comes to preparing their data for AI. The survey found that 63% of organizations lack AI-ready data, which includes data that is high-quality, relevant, and readily available for use in AI models. This lack of preparedness is a major obstacle to AI adoption, as AI models require high-quality data to learn from and make accurate predictions.
The survey also found that 44% of organizations are struggling to integrate their data silos, which are making it difficult to access and share data across the organization. This lack of integration is not only hindering AI adoption but also making it difficult for organizations to gain insights from their data.
Metadata management: The unsung hero
Metadata management is often overlooked, but it is a critical component of AI-ready data. Metadata refers to the data that describes other data, such as the format, structure, and relationships between different data sets. Without accurate and up-to-date metadata, it is difficult to understand the context and meaning of the data, which is critical for AI model training.
The Gartner survey found that 57% of organizations are struggling to manage their metadata, which is making it difficult to prepare their data for AI. This lack of metadata management is not only hindering AI adoption but also making it difficult for organizations to ensure data quality and integrity.
Outdated practices: The biggest obstacle
The survey also found that outdated data management practices are a major obstacle to AI adoption. Many organizations are still using traditional data management practices, such as data warehousing and data lakes, which are not designed to handle the complexity and volume of AI-ready data.
The survey found that 51% of organizations are using traditional data management practices, which are making it difficult to prepare their data for AI. This lack of adaptability is not only hindering AI adoption but also making it difficult for organizations to stay competitive in today’s fast-paced digital landscape.
The consequences of poor data management
The consequences of poor data management can be severe, including:
- Delayed or abandoned AI projects: Without high-quality, relevant, and readily available data, AI projects are likely to be delayed or abandoned, which can result in lost revenue and opportunities.
- Low-quality AI models: Poor data management can result in low-quality AI models, which can lead to inaccurate predictions and poor decision-making.
- Data breaches: Poor data management can result in data breaches, which can compromise sensitive information and damage an organization’s reputation.
- Compliance issues: Poor data management can result in compliance issues, which can result in fines and penalties.
Best practices for AI-ready data management
To overcome the challenges of poor data management, organizations should follow best practices for AI-ready data management, including:
- Data integration: Integrate data from different sources and systems to ensure a single, unified view of the data.
- Data quality: Ensure data quality by cleaning, processing, and validating data to ensure it is accurate and reliable.
- Metadata management: Manage metadata to ensure accurate and up-to-date descriptions of the data.
- Data governance: Establish a data governance framework to ensure data is used responsibly and in accordance with organizational policies and regulations.
- AI-ready data: Ensure data is AI-ready by using data preparation and machine learning algorithms to prepare data for AI model training.
Conclusion
Poor data management is a major obstacle to AI adoption, and organizations must take steps to overcome these challenges to ensure the success of their AI projects. By following best practices for AI-ready data management, organizations can ensure high-quality, relevant, and readily available data for AI model training, which can result in more accurate predictions and better decision-making.
The Gartner survey highlights the growing concern that many organizations are facing when it comes to preparing their data for AI. By understanding the challenges and best practices for AI-ready data management, organizations can overcome these challenges and ensure the success of their AI projects.
News Source:
https://www.dqindia.com/opinion/the-ai-data-dilemma-why-poor-data-management-puts-ai-projects-at-risk-8888254