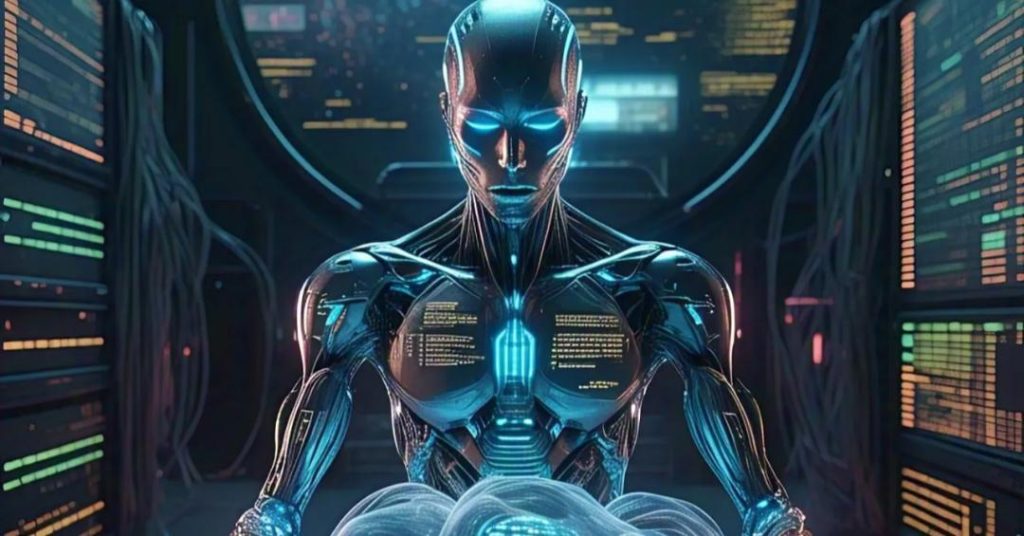
How Poor Data Management Can Put AI Projects at Risk?
Artificial intelligence (AI) has revolutionized the way businesses operate, with many organizations adopting AI solutions to enhance efficiency, improve decision-making, and drive innovation. However, despite the potential benefits of AI, many organizations are struggling to make the most of this technology due to poor data management practices.
A recent survey by Gartner found that 63% of organizations lack AI-ready data, putting their AI projects at risk of failure. The survey, which polled 1,203 data management leaders in 2024, revealed that many organizations are failing to realize the differences between AI-ready requirements and traditional data management practices.
In this blog post, we will explore the impact of poor data management on AI projects and provide insights on how organizations can overcome these challenges to achieve success with AI.
The Challenges of Poor Data Management
Poor data management is a common challenge that many organizations face when adopting AI solutions. AI requires high-quality, well-structured, and easily accessible data to train and test models. However, many organizations have legacy data management systems and practices that are not designed to support AI.
Some of the common challenges of poor data management include:
- Data Quality Issues: Poor data quality can lead to incorrect or incomplete data, which can negatively impact the accuracy and reliability of AI models.
- Data Inconsistencies: Inconsistent data formats, naming conventions, and data structures can make it difficult to integrate data from multiple sources and create a unified view of the data.
- Data Volume and Velocity: The sheer volume and velocity of data can overwhelm traditional data management systems, making it difficult to manage and analyze data in real-time.
- Data Security and Governance: Poor data management practices can compromise data security and governance, putting sensitive data at risk of unauthorized access or breaches.
The Consequences of Poor Data Management
The consequences of poor data management can be severe, and can put AI projects at risk of failure. Some of the consequences include:
- Inaccurate Insights: Poor data management can lead to inaccurate insights, which can negatively impact business decisions and strategy.
- Delayed Projects: Poor data management can delay AI projects, causing budget overruns and missed deadlines.
- Increased Costs: Poor data management can increase costs, as organizations may need to invest in additional infrastructure, personnel, and consulting services to overcome data management challenges.
- Loss of Competitive Advantage: Poor data management can put organizations at a competitive disadvantage, as they may struggle to keep up with the demands of AI-driven innovation.
The Need for AI-Ready Data Management
To overcome the challenges of poor data management and achieve success with AI, organizations need to adopt AI-ready data management practices. Some of the key strategies include:
- Data Integration: Integrating data from multiple sources and formats to create a unified view of the data.
- Data Warehousing: Creating a centralized data warehouse to store and manage large volumes of data.
- Data Analytics: Using data analytics and machine learning algorithms to analyze and visualize data.
- Data Governance: Establishing data governance practices to ensure data security, quality, and consistency.
- Metadata Management: Managing metadata to ensure data consistency, accuracy, and completeness.
Conclusion
Poor data management is a significant challenge that can put AI projects at risk of failure. Organizations need to adopt AI-ready data management practices to overcome these challenges and achieve success with AI. By integrating data from multiple sources, creating a centralized data warehouse, using data analytics and machine learning algorithms, establishing data governance practices, and managing metadata, organizations can ensure high-quality, well-structured, and easily accessible data to support AI initiatives.
Source: