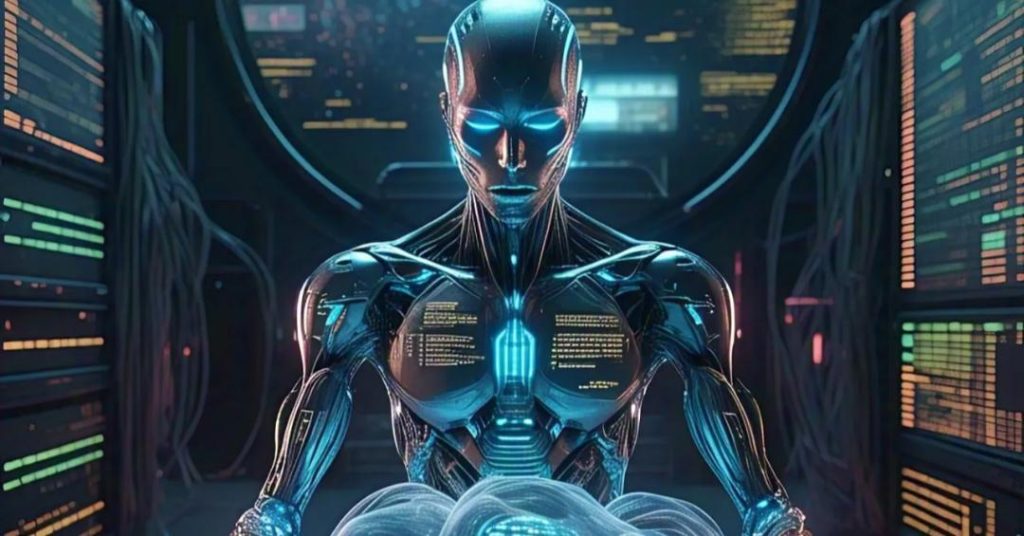
How Poor Data Management Can Put AI Projects at Risk?
Artificial Intelligence (AI) has revolutionized the way businesses operate, making it possible to automate tasks, gain insights, and make data-driven decisions. However, despite its numerous benefits, AI projects are often at risk of failure due to poor data management. A recent survey by Gartner revealed that 63% of organizations lack AI-ready data, making it challenging for them to realize the full potential of AI.
In this blog post, we will explore the consequences of poor data management on AI projects and discuss the importance of metadata management and modern data practices to ensure the success of AI initiatives.
The AI-Data Dilemma
The Gartner survey, which polled 1,203 data management leaders in 2024, found that many organizations are struggling to prepare their data for AI adoption. The survey revealed that 63% of organizations lack AI-ready data, which is characterized by its quality, relevance, and ability to be processed by AI algorithms. This lack of AI-ready data makes it difficult for organizations to derive insights, make predictions, and automate tasks, ultimately putting their AI projects at risk.
So, what are the consequences of poor data management on AI projects? Here are a few:
- Inaccurate Insights: Poor data quality can lead to inaccurate insights, which can result in wrong decisions and negative business outcomes.
- Lack of Trust: When data is not trusted, it is difficult to rely on AI-driven recommendations, leading to a lack of trust in the AI system.
- Slow Development: Poor data management can slow down the development of AI projects, as data scientists and engineers spend more time cleaning, processing, and preparing data.
- Higher Costs: Inaccurate data can lead to higher costs, as organizations may need to reprocess or recollect data, or even restart their AI projects.
Metadata Management: The Key to AI-Ready Data
Metadata management is the process of collecting, organizing, and maintaining data about data, including its structure, format, and relationships. Good metadata management is essential for AI-ready data, as it provides a clear understanding of the data’s meaning, context, and relationships.
Here are a few benefits of metadata management for AI-ready data:
- Improved Data Quality: Metadata management ensures that data is accurate, complete, and consistent, leading to improved data quality.
- Increased Efficiency: Metadata management automates data discovery, data profiling, and data cataloging, increasing efficiency and reducing data preparation time.
- Better Collaboration: Metadata management enables data scientists, engineers, and business stakeholders to collaborate more effectively, as they have a clear understanding of the data’s meaning and context.
Modern Data Practices for AI-Ready Data
In addition to metadata management, modern data practices are essential for AI-ready data. Here are a few:
- Data Lake Architecture: A data lake architecture is a centralized repository that stores all data in its raw format, allowing for better data discovery and analysis.
- Data Lineage: Data lineage is the process of tracking the origin, transformation, and movement of data, enabling organizations to understand the data’s history and quality.
- Data Governance: Data governance is the process of managing data across the organization, ensuring that data is consistent, accurate, and compliant with regulations.
Conclusion
Poor data management is a significant risk to AI projects, leading to inaccurate insights, lack of trust, slow development, and higher costs. Metadata management and modern data practices are essential for AI-ready data, enabling organizations to improve data quality, increase efficiency, and facilitate better collaboration.
In conclusion, organizations must prioritize data management and adopt modern data practices to ensure the success of their AI projects. By doing so, they can unlock the full potential of AI and drive business success.
Source: