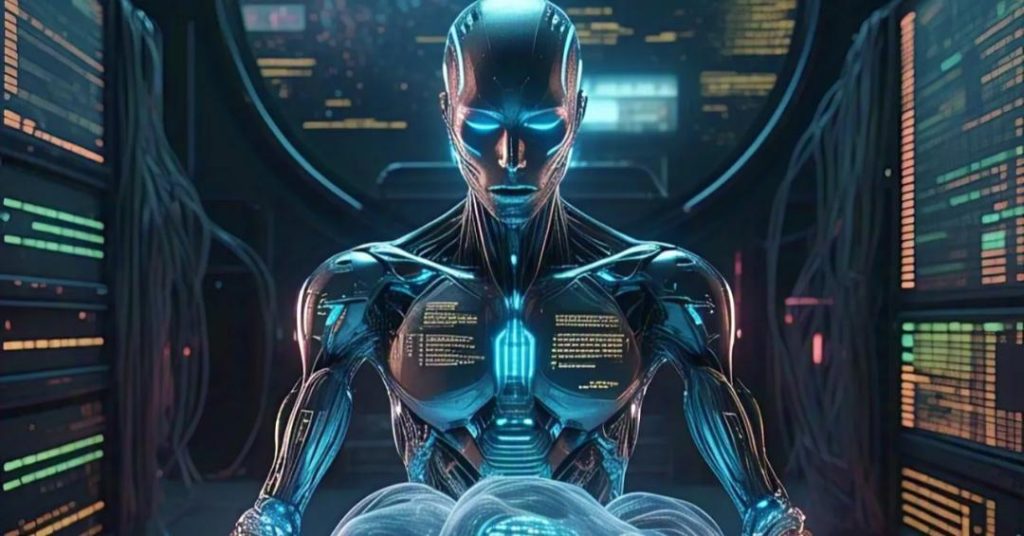
How Poor Data Management Can Put AI Projects at Risk
The adoption of Artificial Intelligence (AI) has been gaining momentum in recent years, with many organizations investing heavily in AI projects to drive innovation and stay ahead of the competition. However, despite the potential benefits, many AI projects are at risk of failing due to poor data management. According to a recent Gartner survey, 63% of organizations lack AI-ready data, which is a significant concern for those looking to unlock the full potential of AI.
The survey, which polled 1,203 data management leaders in 2024, found that poor metadata management and outdated practices are major hurdles to AI adoption. Moreover, many organizations fail to realize the differences between AI-ready requirements and traditional data management, which puts their AI projects at risk of failure.
In this blog post, we will explore the consequences of poor data management on AI projects and provide insights on how organizations can overcome these challenges to ensure the success of their AI initiatives.
The AI Data Dilemma
AI projects require high-quality, AI-ready data to deliver accurate results. However, many organizations struggle to provide this type of data due to poor data management practices. AI-ready data is characterized by its ability to provide insights and patterns that can be used to train machine learning models. This type of data is typically high-quality, relevant, and well-structured.
On the other hand, traditional data management practices are geared towards storing and retrieving large amounts of data, rather than providing insights and patterns. This means that many organizations are struggling to adapt their data management strategies to meet the needs of AI projects.
Consequences of Poor Data Management
Poor data management can have serious consequences for AI projects, including:
- Inaccurate Results: AI models are only as good as the data they are trained on. Poor data management can lead to inaccurate results, which can be costly and time-consuming to correct.
- Delayed Projects: Poor data management can cause delays in AI project timelines, which can have a significant impact on the overall success of the project.
- Increased Costs: Poor data management can lead to increased costs, as organizations may need to re-collect or re-prepare data to meet the needs of their AI projects.
- Loss of Trust: Poor data management can erode trust in AI projects, as stakeholders may lose confidence in the accuracy and reliability of the results.
The Role of Metadata Management
Metadata management plays a critical role in ensuring the success of AI projects. Metadata is the data that describes other data, such as the format, structure, and content of the data. In the context of AI projects, metadata management is essential for providing insights and patterns that can be used to train machine learning models.
Poor metadata management can lead to a range of challenges, including:
- Data Silos: Poor metadata management can lead to data silos, where data is stored in isolation and is not accessible to other parts of the organization.
- Data Inconsistencies: Poor metadata management can lead to data inconsistencies, which can make it difficult to integrate data from different sources.
- Data Quality Issues: Poor metadata management can lead to data quality issues, which can impact the accuracy and reliability of AI models.
Best Practices for AI-Ready Data Management
To overcome the challenges of poor data management and ensure the success of AI projects, organizations should adopt the following best practices:
- Implement a Data Governance Framework: Implement a data governance framework that outlines the roles and responsibilities of different stakeholders in managing data.
- Use AI-Ready Data Formats: Use AI-ready data formats, such as JSON or CSV, to make it easier to integrate data from different sources.
- Provide High-Quality Data: Provide high-quality data that is relevant, well-structured, and free from errors.
- Use Metadata Management Tools: Use metadata management tools to provide insights and patterns that can be used to train machine learning models.
- Monitor Data Quality: Monitor data quality regularly to ensure that data meets the needs of AI projects.
Conclusion
Poor data management can put AI projects at risk of failure. To overcome this challenge, organizations must adopt best practices for AI-ready data management, including implementing a data governance framework, using AI-ready data formats, providing high-quality data, using metadata management tools, and monitoring data quality. By adopting these best practices, organizations can ensure the success of their AI projects and unlock the full potential of AI.