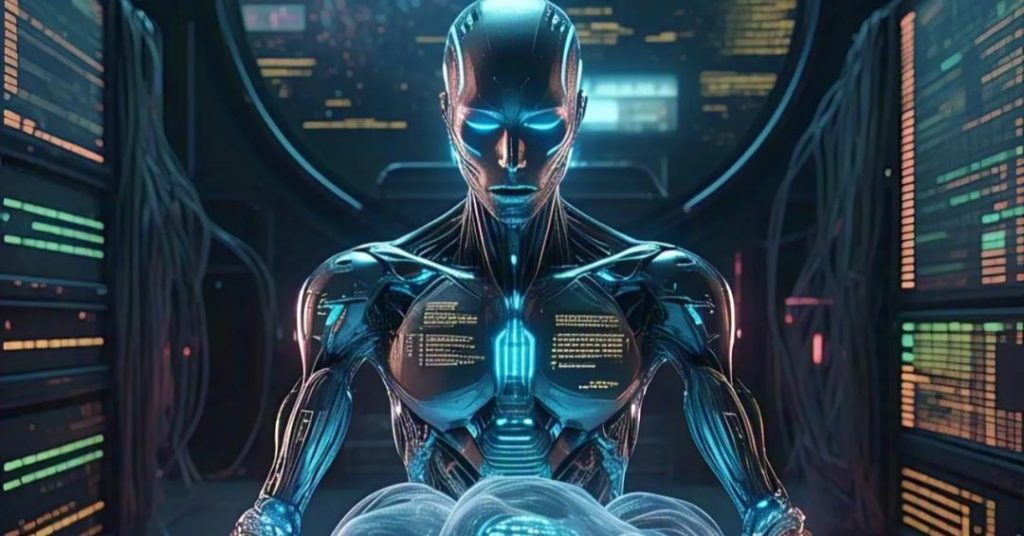
How Poor Data Management Can Put AI Projects at Risk?
Artificial Intelligence (AI) has revolutionized the way businesses operate, making it possible to automate tasks, gain insights, and make data-driven decisions. However, for AI projects to succeed, it is crucial to have high-quality data that is accurately processed and analyzed. Unfortunately, a recent survey by Gartner reveals that many organizations lack AI-ready data, putting their AI projects at risk.
According to the survey, 63% of organizations lack AI-ready data, which is a significant concern given the critical role that data plays in AI projects. AI-ready data refers to data that is accurate, complete, and relevant, and that can be easily accessed and analyzed. Without such data, AI algorithms cannot function effectively, leading to poor performance and inaccurate results.
The survey, which polled 1,203 data management leaders in 2024, found that organizations that fail to recognize the differences between AI-ready requirements and traditional data management practices are more likely to encounter difficulties in their AI projects. This highlights the need for organizations to adopt new data management practices that are specifically designed for AI initiatives.
So, what are the consequences of poor data management on AI projects? Here are some of the risks that organizations face:
- Inaccurate Results: Poor data quality can lead to inaccurate results, which can have serious consequences for businesses. For instance, if an AI algorithm is trained on biased or incomplete data, it may produce biased results, leading to wrong decisions being made.
- Poor Performance: AI algorithms require high-quality data to perform well. If the data is of poor quality, the algorithm may not be able to learn effectively, leading to poor performance and inaccurate results.
- Increased Costs: Poor data management can lead to increased costs, as organizations may need to spend more time and resources to clean and preprocess the data.
- Delayed Projects: Poor data management can delay AI projects, as organizations may need to spend more time and resources to address data quality issues.
- Lack of Trust: Poor data management can erode trust in AI systems, as users may not have confidence in the accuracy of the results.
So, what are some of the common pitfalls that organizations face when it comes to data management for AI projects? Here are some of the most common issues:
- Lack of Standardization: One of the most common pitfalls is the lack of standardization in data management practices. Different teams and departments may have different data management practices, leading to inconsistencies and inaccuracies.
- Inadequate Data Governance: Another common issue is inadequate data governance, which refers to the lack of policies and procedures to manage and control data. This can lead to data being stored in different formats and locations, making it difficult to access and analyze.
- Outdated Practices: Many organizations are still using outdated data management practices, such as manual data entry and spreadsheets, which can lead to inaccuracies and inefficiencies.
- Lack of Metadata Management: Metadata management refers to the process of managing and organizing data about data, such as data quality, data lineage, and data provenance. Without effective metadata management, it can be difficult to understand the quality and accuracy of the data.
So, how can organizations overcome these challenges and ensure that their AI projects are successful? Here are some best practices to follow:
- Implement Standardized Data Management Practices: Organizations should implement standardized data management practices across different teams and departments to ensure consistency and accuracy.
- Establish Effective Data Governance: Organizations should establish effective data governance practices, including policies and procedures to manage and control data.
- Use Modern Data Management Tools: Organizations should use modern data management tools, such as data lakes, data warehouses, and data integration platforms, to manage and analyze data.
- Focus on Metadata Management: Organizations should focus on metadata management, including data quality, data lineage, and data provenance, to ensure that the data is accurate and trustworthy.
- Invest in Data Quality: Organizations should invest in data quality initiatives, including data cleansing, data normalization, and data validation, to ensure that the data is accurate and complete.
In conclusion, poor data management can put AI projects at risk, leading to inaccurate results, poor performance, increased costs, delayed projects, and lack of trust. To overcome these challenges, organizations should implement standardized data management practices, establish effective data governance, use modern data management tools, focus on metadata management, and invest in data quality initiatives. By following these best practices, organizations can ensure that their AI projects are successful and that they can reap the benefits of AI adoption.