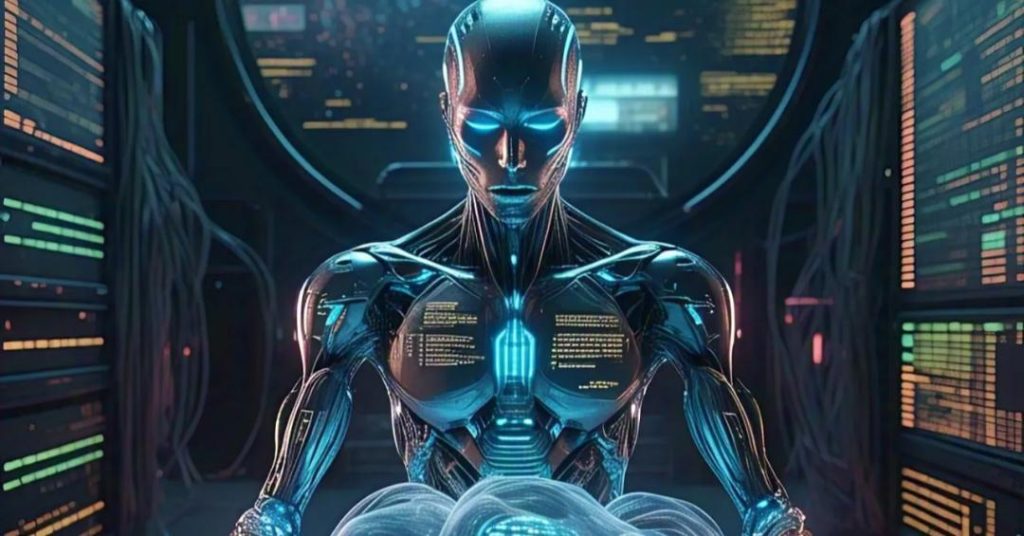
How Poor Data Management Can Put AI Projects at Risk?
The advent of Artificial Intelligence (AI) has brought about unprecedented opportunities for businesses to transform themselves and stay ahead of the competition. However, the success of AI projects largely depends on the quality and availability of data. A recent survey by Gartner revealed that a staggering 63% of organisations lack AI-ready data, putting their AI projects at risk of failure.
The survey, which polled 1,203 data management leaders in 2024, found that organisations that fail to understand the differences between AI-ready requirements and traditional data management practices are more likely to encounter issues with their AI projects. This highlights the importance of effective data management in AI adoption and implementation.
Poor metadata management and outdated practices are among the key factors contributing to the poor state of data quality. Metadata, which refers to data that provides information about other data, is crucial for AI systems to understand the context and meaning of the data. Without accurate and complete metadata, AI models may struggle to learn from the data, leading to inaccurate predictions and recommendations.
Outdated data management practices, such as relying on manual data processing and lack of data governance, can also hinder AI adoption. AI systems require large amounts of high-quality data to learn from, and manual processing can lead to errors, inconsistencies, and inaccuracies. Furthermore, lack of data governance can result in data silos, making it difficult to access and integrate data from different sources.
The consequences of poor data management can be severe. AI projects may fail to deliver expected results, leading to wasted resources, lost revenue, and damaged reputation. Moreover, poor data quality can also lead to biased AI models, which can perpetuate discrimination and unfairness.
So, what can organisations do to ensure that their data is AI-ready? Here are some best practices to follow:
- Understand AI-ready requirements: AI projects require high-quality, accurate, and complete data. Organisations must understand the specific requirements of their AI projects and ensure that their data meets those requirements.
- Develop a data governance strategy: A data governance strategy helps to ensure that data is accurate, complete, and consistent. It also helps to establish data standards, policies, and procedures for data management.
- Implement data quality checks: Data quality checks can help to identify and correct errors, inconsistencies, and inaccuracies in data. This is particularly important for AI projects, which require high-quality data to learn from.
- Use metadata management tools: Metadata management tools can help to capture, manage, and utilise metadata. This is critical for AI systems, which require accurate and complete metadata to understand the context and meaning of the data.
- Leverage automation and machine learning: Automation and machine learning can help to streamline data processing and improve data quality. These technologies can also help to identify patterns and anomalies in data, which can inform business decisions.
In conclusion, poor data management is a significant risk to AI projects. Organisations must understand the differences between AI-ready requirements and traditional data management practices to ensure that their data is high-quality, accurate, and complete. By following best practices such as developing a data governance strategy, implementing data quality checks, and leveraging automation and machine learning, organisations can overcome the challenges of poor data management and successfully implement AI projects.