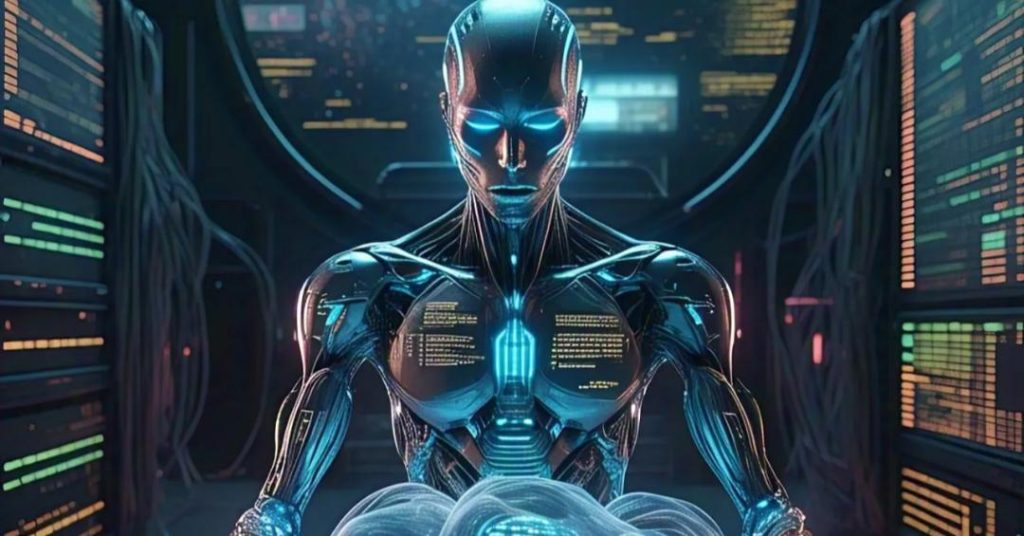
How Poor Data Management Can Put AI Projects at Risk
The adoption of Artificial Intelligence (AI) has become a top priority for many organizations, as it promises to revolutionize the way they operate and make data-driven decisions. However, a recent survey by Gartner revealed that a staggering 63% of organizations lack AI-ready data, putting their AI projects at risk of failure. This highlights the importance of effective data management in ensuring the success of AI initiatives.
The survey, which polled 1,203 data management leaders in 2024, found that organizations failing to recognize the differences between AI-ready requirements and traditional data management are endangering the success of their AI efforts. This is a concerning trend, as AI projects require high-quality, relevant, and easily accessible data to produce accurate results.
So, what is causing this data management conundrum, and how can organizations overcome it?
The Challenges of AI-Ready Data
AI-ready data is more than just having a large amount of data. It requires data that is accurate, relevant, and formatted in a way that can be easily consumed by AI algorithms. This can be a significant challenge for many organizations, as their existing data management practices may not be equipped to handle the complexities of AI-ready data.
One of the main challenges is the lack of metadata management. Metadata is the information that describes the structure and content of data, such as data types, formats, and relationships. In the context of AI, metadata is critical in helping algorithms understand the meaning and relevance of the data. Without proper metadata management, AI algorithms may struggle to interpret the data correctly, leading to inaccurate results.
Another challenge is the outdated practices and tools used in traditional data management. Many organizations are still relying on manual processes and legacy systems to manage their data, which can be time-consuming and prone to errors. AI projects require data to be readily available and easily accessible, which can be difficult to achieve with outdated practices and tools.
The Consequences of Poor Data Management
The consequences of poor data management can be severe for AI projects. Without high-quality, AI-ready data, organizations may struggle to achieve the desired outcomes from their AI initiatives. This can lead to:
- Inaccurate results: AI algorithms can only produce accurate results if they are given high-quality, relevant data. Poor data management can lead to inaccurate results, which can undermine the credibility of the AI project.
- Delays: Poor data management can slow down the development and deployment of AI projects, as data needs to be manually prepared and cleaned before it can be used.
- Increased costs: Poor data management can increase the costs of AI projects, as organizations may need to invest in additional resources and expertise to overcome the challenges of poor data management.
Best Practices for Effective Data Management
So, what can organizations do to overcome the challenges of poor data management and ensure the success of their AI projects? Here are some best practices:
- Implement a metadata management strategy: Organizations should implement a metadata management strategy that provides a single source of truth for data metadata. This can help ensure that data is consistent, accurate, and easily accessible.
- Use modern data management tools: Organizations should use modern data management tools that are designed to handle the complexities of AI-ready data. These tools can help automate data preparation, cleaning, and processing, making it easier to get high-quality data into AI algorithms.
- Focus on data quality: Organizations should prioritize data quality, ensuring that data is accurate, complete, and relevant. This can involve implementing data quality checks, data validation, and data cleansing processes.
- Develop a data governance strategy: Organizations should develop a data governance strategy that outlines the roles, responsibilities, and policies for data management. This can help ensure that data is managed consistently and in accordance with organizational policies and regulations.
Conclusion
The success of AI projects depends on having high-quality, AI-ready data. Poor data management can put AI projects at risk, leading to inaccurate results, delays, and increased costs. To overcome the challenges of poor data management, organizations should implement a metadata management strategy, use modern data management tools, focus on data quality, and develop a data governance strategy. By following these best practices, organizations can ensure that their AI projects are successful and deliver the desired outcomes.