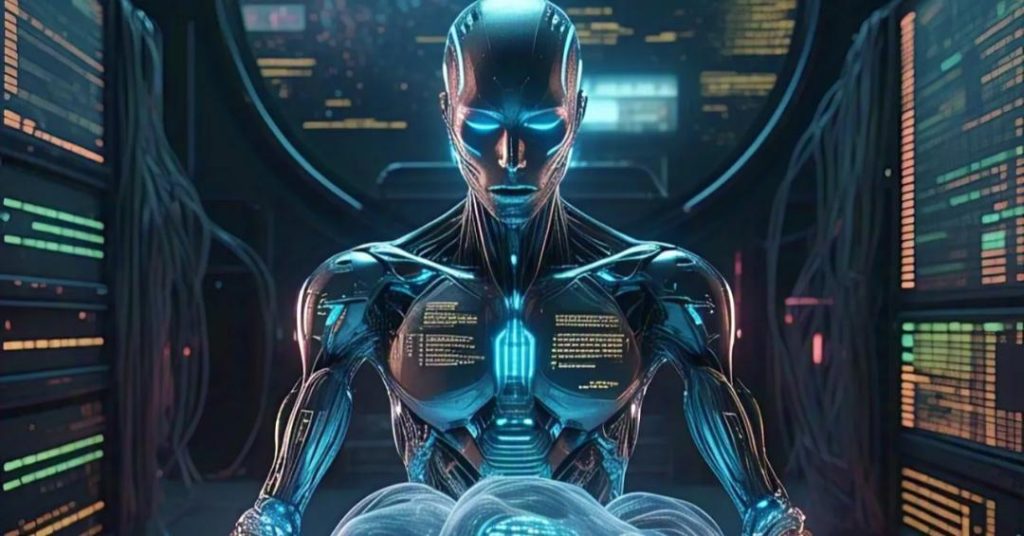
How Poor Data Management Can Put AI Projects at Risk?
Artificial Intelligence (AI) has become a crucial aspect of modern businesses, with organizations leveraging its potential to drive innovation, improve efficiency, and make informed decisions. However, the success of AI projects depends on various factors, including the quality of the data used to train and test these systems. Unfortunately, a recent survey by Gartner reveals that a staggering 63% of organizations lack AI-ready data, putting their AI projects at risk of failure.
The survey, which polled 1,203 data management leaders in 2024, found that poor data management practices and inadequate metadata management are significant barriers to AI adoption. Organizations failing to understand the differences between AI-ready data requirements and traditional data management are likely to face challenges in their AI initiatives.
In this blog post, we will explore the importance of data management in AI projects and the risks associated with poor data management practices.
The Role of Data in AI Projects
Data is the lifeblood of any AI project. It is used to train and test AI models, which are then deployed to perform specific tasks such as image recognition, natural language processing, and predictive analytics. The quality and quantity of data used in AI projects have a direct impact on their accuracy, efficiency, and overall performance.
AI models require high-quality data to learn and improve over time. This data should be relevant, accurate, and diverse, covering a wide range of scenarios and conditions. Poor-quality data, on the other hand, can lead to biased or inaccurate AI models, which can have serious consequences in applications such as healthcare, finance, and transportation.
Challenges in AI-Ready Data Management
While AI projects require high-quality data, many organizations struggle to manage their data effectively. The Gartner survey found that 63% of organizations lack AI-ready data, which is characterized by its ability to be used for machine learning and deep learning algorithms. This lack of AI-ready data is attributed to various factors, including:
- Inadequate metadata management: Metadata is critical in AI projects, as it provides context and meaning to the data. Poor metadata management can lead to data inconsistencies, errors, and inaccuracies, which can negatively impact AI model performance.
- Outdated data management practices: Many organizations are still using traditional data management practices, which are not designed to handle the complexities of AI projects. These practices may not provide the level of data quality, security, and scalability required for AI applications.
- Lack of data governance: Data governance is essential in AI projects, as it ensures that data is collected, stored, and used in a responsible and ethical manner. Without effective data governance, organizations may face data quality issues, security breaches, and compliance risks.
Consequences of Poor Data Management in AI Projects
The consequences of poor data management in AI projects can be severe, including:
- Inaccurate AI models: Poor-quality data can lead to biased or inaccurate AI models, which can have serious consequences in applications such as healthcare, finance, and transportation.
- Project delays and failures: Poor data management can lead to project delays and failures, as AI models may not perform as expected or may require significant rework to address data quality issues.
- Loss of trust and reputation: Organizations that fail to manage their data effectively may lose the trust and confidence of their customers, partners, and stakeholders, damaging their reputation and brand.
- Security risks: Poor data management can lead to security risks, as sensitive data may be exposed to unauthorized access or breaches.
Best Practices for AI-Ready Data Management
To avoid the risks associated with poor data management, organizations should adopt best practices for AI-ready data management, including:
- Develop a data governance framework: Establish a data governance framework that outlines data collection, storage, and use policies and procedures.
- Implement metadata management: Implement metadata management practices that provide context and meaning to the data.
- Use data quality tools: Use data quality tools to ensure that data is accurate, complete, and consistent.
- Invest in data analytics: Invest in data analytics tools and techniques to analyze and improve data quality, security, and scalability.
- Develop a data strategy: Develop a data strategy that aligns with the organization’s AI goals and objectives.
Conclusion
Poor data management can put AI projects at risk of failure, leading to inaccurate AI models, project delays, and security risks. To avoid these risks, organizations should adopt best practices for AI-ready data management, including developing a data governance framework, implementing metadata management, using data quality tools, investing in data analytics, and developing a data strategy.
By prioritizing data management, organizations can ensure the success of their AI projects and reap the benefits of AI adoption, including increased efficiency, improved decision-making, and enhanced customer experiences.
Source: