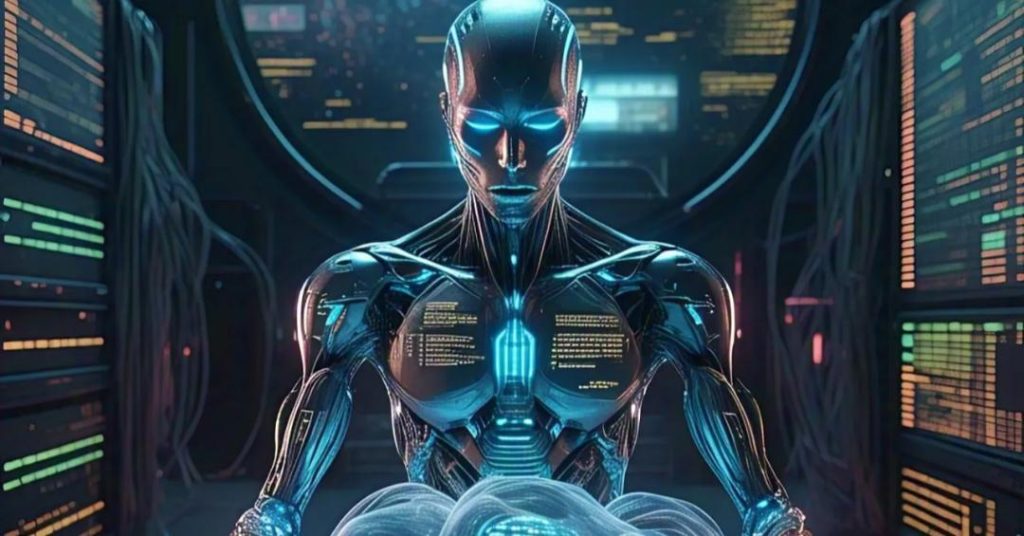
How Poor Data Management Can Put AI Projects at Risk?
The rapid advancement of Artificial Intelligence (AI) has led to an increased demand for data-driven insights. However, a recent survey by Gartner has revealed that 63% of organizations lack AI-ready data, putting their AI projects at risk. The survey of 1,203 data management leaders in 2024 found that organizations failing to realize the differences between AI-ready requirements and traditional data management are endangering the success of their efforts.
The importance of AI-ready data cannot be overstated. AI algorithms require high-quality, accurate, and relevant data to learn from and make informed decisions. Poor data management can lead to inaccurate predictions, biased decision-making, and reduced model performance. In this blog post, we will explore the consequences of poor data management on AI projects and provide insights on how organizations can overcome these challenges.
Consequences of Poor Data Management
Poor data management can have a significant impact on AI projects. Some of the consequences include:
- Inaccurate Predictions: AI algorithms require high-quality data to make accurate predictions. Poor data management can lead to inaccurate predictions, which can result in costly mistakes and reduced model performance.
- Biased Decision-Making: AI algorithms can learn biases present in the data, leading to biased decision-making. This can have serious consequences, particularly in fields such as finance, healthcare, and education.
- Reduced Model Performance: Poor data management can lead to reduced model performance, making it more challenging to achieve the desired outcomes.
- Increased Costs: Poor data management can lead to increased costs, as organizations may need to spend more time and resources collecting and cleaning data.
Causes of Poor Data Management
Poor data management is often caused by outdated practices and a lack of understanding of AI-ready requirements. Some of the common causes include:
- Lack of Data Governance: Poor data governance can lead to data quality issues, inconsistent data formats, and a lack of transparency.
- Inadequate Data Quality: Inadequate data quality can lead to inaccurate predictions, biased decision-making, and reduced model performance.
- Outdated Data Management Practices: Outdated data management practices can lead to inefficiencies, errors, and increased costs.
- Lack of AI-Ready Data: Many organizations lack AI-ready data, making it challenging to achieve the desired outcomes.
Solutions to Overcome Poor Data Management
To overcome poor data management, organizations can take the following steps:
- Establish Data Governance: Establishing data governance is essential to ensure data quality, consistency, and transparency.
- Improve Data Quality: Improving data quality is critical to achieving accurate predictions and reducing biased decision-making.
- Adopt AI-Ready Data Management Practices: Adopting AI-ready data management practices can help organizations overcome the challenges of poor data management.
- Invest in Data Science: Investing in data science can help organizations develop the skills and expertise needed to manage AI-ready data.
Best Practices for AI-Ready Data Management
To ensure AI-ready data management, organizations can follow the best practices listed below:
- Define AI-Ready Requirements: Define AI-ready requirements to ensure data meets the necessary standards.
- Use Data Lineage: Use data lineage to track the origin, movement, and storage of data.
- Implement Data Quality Checks: Implement data quality checks to ensure data accuracy and consistency.
- Use Machine Learning: Use machine learning to automate data processing and reduce errors.
- Invest in Data Visualization: Invest in data visualization to provide insights into data quality and performance.
Conclusion
Poor data management can put AI projects at risk, leading to inaccurate predictions, biased decision-making, and reduced model performance. To overcome these challenges, organizations must establish data governance, improve data quality, adopt AI-ready data management practices, and invest in data science. By following the best practices outlined above, organizations can ensure AI-ready data management and achieve the desired outcomes.
News Source:
https://www.dqindia.com/opinion/the-ai-data-dilemma-why-poor-data-management-puts-ai-projects-at-risk-8888254