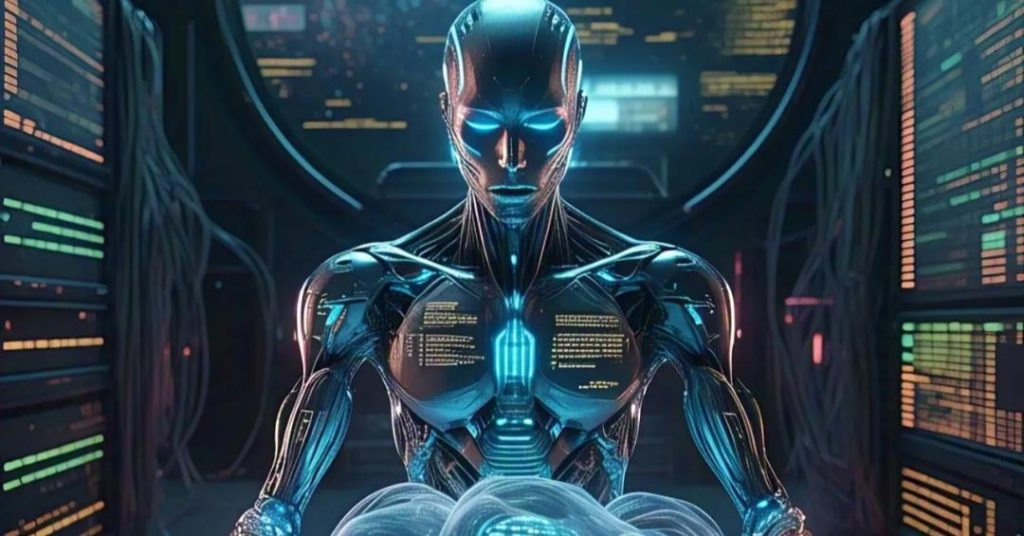
How Poor Data Management Can Put AI Projects at Risk?
Artificial Intelligence (AI) has revolutionized the way businesses operate, making it possible to automate processes, make data-driven decisions, and gain a competitive edge. However, the success of AI projects heavily relies on the quality and availability of data. Unfortunately, many organizations are failing to manage their data effectively, putting their AI projects at risk.
According to a recent Gartner survey, 63% of organizations lack AI-ready data, which is a significant concern for businesses investing in AI initiatives. The survey, conducted in 2024, involved 1,203 data management leaders and revealed that poor metadata management and outdated practices are major hurdles in the adoption of AI.
In this blog post, we will explore the importance of data management in AI projects, the consequences of poor data management, and the steps organizations can take to ensure the success of their AI initiatives.
The Importance of Data Management in AI Projects
AI projects rely heavily on data to learn, improve, and make predictions. The quality and quantity of data can significantly impact the performance of AI models. Poor data management can lead to inaccurate predictions, biased outcomes, and poor decision-making.
AI-ready data refers to data that is clean, curated, and optimized for AI applications. This type of data is essential for training AI models, which require large amounts of high-quality data to learn and improve.
The Consequences of Poor Data Management
Poor data management can have severe consequences on AI projects, including:
- Inaccurate Predictions: Poor data quality can lead to inaccurate predictions, which can result in costly mistakes and reputational damage.
- Biased Outcomes: Biased data can lead to biased outcomes, which can perpetuate discrimination and inequality.
- Poor Decision-Making: Poor data management can lead to poor decision-making, which can negatively impact business outcomes and strategy.
- Increased Costs: Poor data management can lead to increased costs, including the cost of data cleansing, data integration, and data migration.
- Delayed Projects: Poor data management can lead to delayed projects, which can impact business timelines and goals.
The Role of Metadata Management
Metadata management is the process of managing the data that describes other data. Metadata includes information such as data quality, data lineage, and data usage. Effective metadata management is critical in AI projects, as it provides insights into data quality, data accuracy, and data relevance.
Poor metadata management can lead to data inconsistencies, data inaccuracies, and data inconsistencies, which can negatively impact AI projects. Effective metadata management can help organizations to:
- Improve Data Quality: Metadata management can help organizations to identify and correct data inconsistencies and inaccuracies.
- Reduce Data Silos: Metadata management can help organizations to reduce data silos, which can improve data sharing and collaboration.
- Improve Data Governance: Metadata management can help organizations to improve data governance, which can improve data security and compliance.
The Importance of Outdated Practices
Outdated practices, such as data silos, data duplication, and data inconsistencies, can hinder AI adoption. These practices can lead to poor data quality, poor data accuracy, and poor data relevance, which can negatively impact AI projects.
Steps to Ensure the Success of AI Projects
To ensure the success of AI projects, organizations must prioritize data management. Here are some steps that organizations can take:
- Develop a Data Strategy: Organizations should develop a data strategy that outlines their data management goals, objectives, and policies.
- Improve Data Quality: Organizations should prioritize data quality, which includes data cleansing, data integration, and data migration.
- Implement Metadata Management: Organizations should implement metadata management, which includes data lineage, data quality, and data usage.
- Reduce Data Silos: Organizations should reduce data silos, which can improve data sharing and collaboration.
- Invest in Data Governance: Organizations should invest in data governance, which can improve data security and compliance.
Conclusion
Poor data management can put AI projects at risk, leading to inaccurate predictions, biased outcomes, and poor decision-making. Effective metadata management and the avoidance of outdated practices are critical in AI projects. Organizations that prioritize data management can ensure the success of their AI initiatives and gain a competitive edge in the market.
News Source: