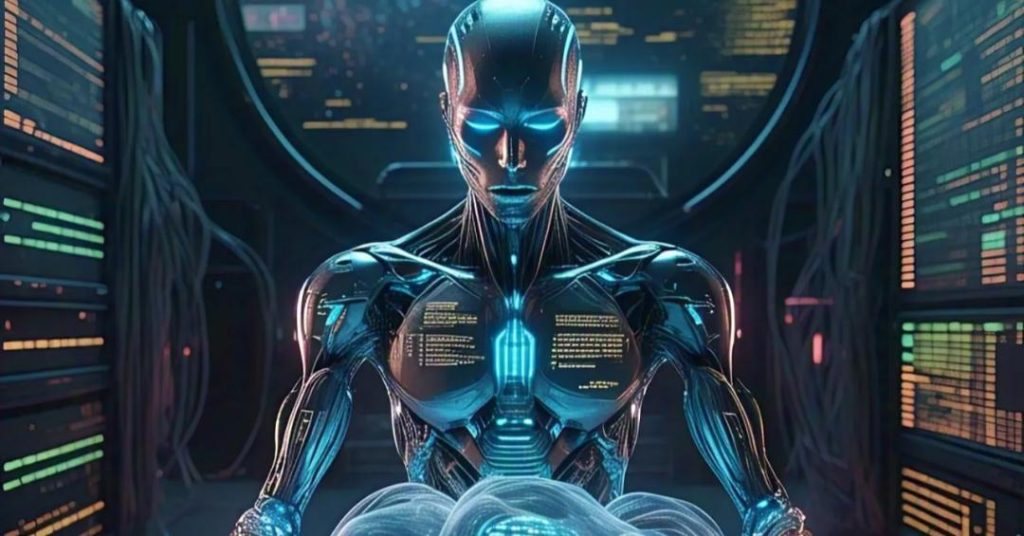
How Poor Data Management Can Put AI Projects at Risk?
Artificial Intelligence (AI) has been hailed as the next big thing in the technological world, with companies and organizations globally investing heavily in AI projects to gain a competitive edge. However, despite the hype, many AI projects are at risk of failing due to poor data management practices. A recent survey by Gartner revealed that a staggering 63% of organizations lack AI-ready data, putting the success of their AI projects in jeopardy.
The survey, which polled 1,203 data management leaders in 2024, found that organizations that fail to differentiate between AI-ready data requirements and traditional data management practices are significantly more likely to encounter issues with their AI projects. This highlights the importance of proper data management in AI adoption and the need for organizations to reassess their data management strategies to ensure they are adequately prepared for AI initiatives.
So, what are the consequences of poor data management on AI projects? Let’s dive deeper into the topic to understand the risks and challenges associated with it.
Why Data Management Matters in AI Projects
Data is the backbone of any AI project. Without high-quality, relevant, and accurately labeled data, AI models are unable to learn and improve. Poor data management can lead to a range of issues, including:
- Data Quality: Low-quality data can lead to inaccurate AI models, which can result in poor decision-making and negatively impact business outcomes.
- Data Inconsistency: Inconsistent data can lead to inconsistencies in AI model performance, making it difficult to train and deploy models.
- Data Incomplete: Incomplete data can result in AI models that are unable to make accurate predictions or decisions.
- Data Silos: Data silos can prevent organizations from accessing and integrating data across different departments and systems, hindering the development of AI models.
Consequences of Poor Data Management on AI Projects
The consequences of poor data management on AI projects can be severe, including:
- Delayed Project timelines: Poor data management can lead to delays in project timelines, resulting in increased costs and reduced ROI.
- Higher Costs: Poor data management can lead to increased costs due to the need for data cleaning, preprocessing, and reprocessing.
- Reduced Accuracy: Poor data management can result in reduced accuracy of AI models, leading to poor decision-making and negative business outcomes.
- Loss of Customer Trust: Poor data management can lead to data breaches or leaks, resulting in loss of customer trust and reputation damage.
Best Practices for Data Management in AI Projects
To avoid the pitfalls of poor data management, organizations can follow the following best practices:
- Data Governance: Establish a data governance framework that outlines data management policies, procedures, and roles.
- Data Quality: Implement data quality checks and monitoring to ensure data accuracy, completeness, and consistency.
- Data Integration: Integrate data from different sources and systems to ensure seamless data flow and accessibility.
- Metadata Management: Implement metadata management to track and manage data lineage, quality, and usage.
- Data Visualization: Use data visualization tools to track and monitor data quality, accuracy, and completeness.
Conclusion
Poor data management is a significant risk to AI projects, and organizations that fail to address these issues can expect delayed project timelines, increased costs, reduced accuracy, and loss of customer trust. By implementing best practices for data management, organizations can ensure they are adequately prepared for AI initiatives and can unlock the full potential of AI to drive business growth and innovation.