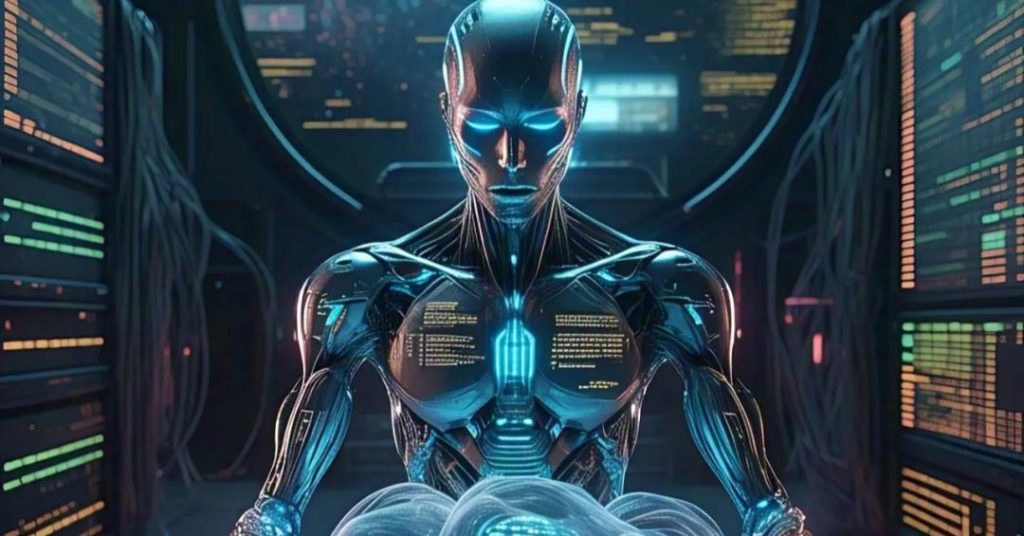
How Poor Data Management Can Put AI Projects at Risk
The rapid growth of Artificial Intelligence (AI) has led to a surge in the adoption of AI-powered solutions across various industries. However, a recent survey by Gartner has revealed a concerning trend that can threaten the success of these projects. According to the survey, 63% of organizations lack AI-ready data, putting their AI projects at risk of failure. In this blog post, we will explore the reasons behind this statistic and the importance of proper data management for AI projects.
The Gartner survey, which polled 1,203 data management leaders in 2024, found that organizations struggle to differentiate between AI-ready data requirements and traditional data management practices. This lack of understanding can lead to poor data management, which can hinder the adoption of AI solutions. The survey highlights the need for organizations to prioritize data management and to ensure that their data is AI-ready.
So, what are the consequences of poor data management on AI projects? Let’s take a closer look:
Metadata Management Issues
Metadata is a critical component of data management, as it provides context and meaning to the data. However, many organizations struggle with metadata management, which can lead to issues such as:
- Inconsistent data formats and structures
- Lack of data standardization
- Inadequate data documentation
- Inefficient data search and retrieval
These issues can hinder the ability of AI models to accurately process and analyze data, leading to poor performance and inaccurate results.
Outdated Data Practices
Outdated data practices can also put AI projects at risk. For example, many organizations still rely on manual data processing and analysis, which can be time-consuming and prone to errors. Additionally, the lack of automation and orchestration in data processing can lead to delays and inefficiencies.
Data Quality Issues
Data quality is another critical aspect of AI project success. Poor data quality can lead to inaccurate or incomplete data, which can negatively impact AI model performance. Common data quality issues include:
- Data inconsistencies
- Duplicate or redundant data
- Inaccurate or outdated data
- Lack of data validation and verification
Data Governance Challenges
Data governance is essential for ensuring the integrity and security of data. However, many organizations struggle with data governance, particularly in the context of AI projects. Data governance challenges can include:
- Lack of clear data ownership and responsibility
- Inadequate data security and compliance measures
- Inefficient data access and control mechanisms
- Inadequate data backup and recovery procedures
The Consequences of Poor Data Management
The consequences of poor data management on AI projects can be severe. Some of the potential consequences include:
- Poor AI model performance
- Inaccurate or incomplete results
- Delayed project timelines
- Increased costs and expenses
- Loss of business opportunities and revenue
Best Practices for AI-Ready Data Management
So, what can organizations do to ensure that their data is AI-ready? Here are some best practices to follow:
- Prioritize Metadata Management: Ensure that metadata is accurate, consistent, and well-documented. This will help AI models to accurately process and analyze data.
- Implement Modern Data Practices: Adopt modern data practices such as automation, orchestration, and cloud-based solutions to streamline data processing and analysis.
- Focus on Data Quality: Ensure that data is accurate, complete, and consistent. Implement data validation and verification procedures to ensure data quality.
- Establish Data Governance: Establish clear data ownership and responsibility, and implement data security and compliance measures to ensure data integrity and security.
- Monitor and Optimize: Continuously monitor and optimize data management processes to ensure that data is AI-ready and that AI models perform accurately and efficiently.
Conclusion
In conclusion, poor data management can put AI projects at risk. Organizations must prioritize data management and ensure that their data is AI-ready. By following best practices such as prioritizing metadata management, implementing modern data practices, focusing on data quality, establishing data governance, and monitoring and optimizing data management processes, organizations can ensure the success of their AI projects.
Source: