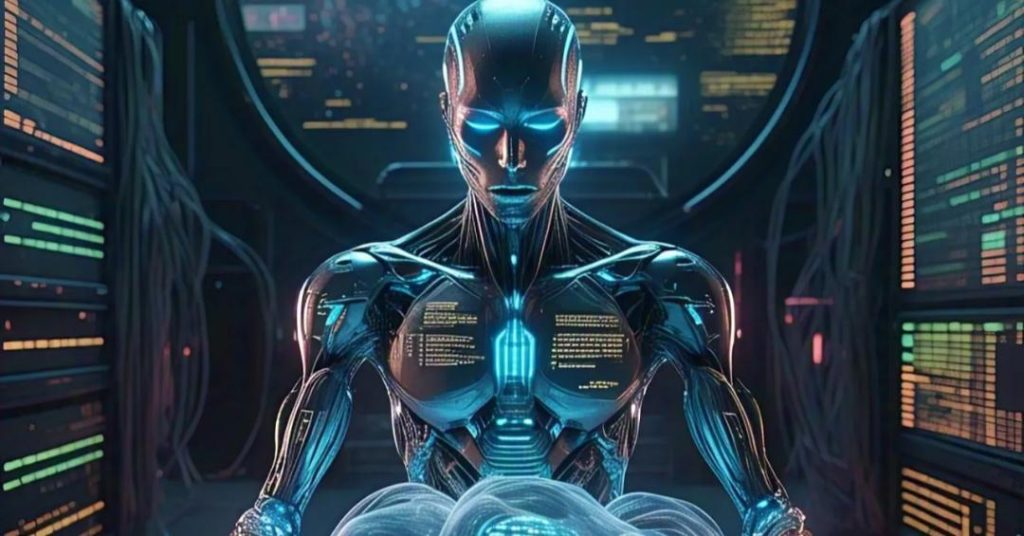
How Poor Data Management Can Put AI Projects at Risk?
Artificial intelligence (AI) has become a buzzword in today’s digital landscape, with many organizations eager to leverage its potential to drive innovation and growth. However, a recent survey by Gartner has sounded a warning bell, highlighting the critical role of data management in AI projects. The survey found that a staggering 63% of organizations lack AI-ready data, putting their AI projects at risk of failure. In this blog post, we will delve into the reasons behind this alarming statistic and explore the consequences of poor data management on AI adoption.
The AI Data Dilemma
The Gartner survey, which polled 1,203 data management leaders in 2024, revealed that organizations are struggling to manage their data effectively, particularly when it comes to AI-ready data. AI-ready data requires specific characteristics, such as high-quality, relevant, and timely data, which is not always the case in traditional data management practices. The survey found that many organizations are failing to realize the differences between AI-ready requirements and traditional data management, leading to a significant risk of AI project failure.
Consequences of Poor Data Management
Poor data management can have far-reaching consequences for AI projects, including:
- Inaccurate Insights: Poor quality data can lead to inaccurate insights, which can be detrimental to AI model performance and decision-making.
- Delayed or Abandoned Projects: Inadequate data management can cause AI projects to be delayed or abandoned altogether, resulting in missed opportunities and lost investments.
- Increased Costs: Poor data management can lead to increased costs, as organizations are forced to rework or re-source data, or even restart entire projects.
- Loss of Competitive Advantage: In today’s fast-paced digital landscape, organizations that fail to leverage AI effectively can lose their competitive edge, making it challenging to recover.
Metadata Management: A Critical Component
Metadata management is a critical component of AI-ready data, as it enables organizations to understand the context, quality, and relevance of their data. Poor metadata management can lead to:
- Data Silos: Data silos can occur when metadata is not properly managed, making it difficult to integrate data from different sources and silos.
- Inconsistent Data Quality: Inconsistent data quality can lead to inaccurate insights, poor decision-making, and decreased trust in AI models.
- Lack of Data Governance: Poor metadata management can result in a lack of data governance, making it challenging to manage data access, security, and compliance.
Outdated Practices Hinder AI Adoption
The survey also found that outdated practices are hindering AI adoption, including:
- Lack of Data Standardization: Lack of data standardization can lead to data inconsistencies, making it challenging to integrate data from different sources.
- Inadequate Data Quality: Inadequate data quality can lead to inaccurate insights, poor decision-making, and decreased trust in AI models.
- Insufficient Data Governance: Insufficient data governance can result in a lack of control over data access, security, and compliance.
Best Practices for AI-Ready Data Management
To avoid the pitfalls of poor data management and ensure the success of AI projects, organizations should follow best practices, including:
- Data Standardization: Standardize data formats, structures, and naming conventions to ensure consistency and interoperability.
- Data Quality: Ensure data quality by implementing data validation, data cleansing, and data profiling techniques.
- Metadata Management: Implement metadata management practices to ensure data governance, data security, and data compliance.
- Data Integration: Integrate data from different sources and silos to create a single, unified view of data.
- Continuous Monitoring: Continuously monitor data quality, data governance, and data compliance to ensure the integrity of AI-ready data.
Conclusion
In conclusion, poor data management is a significant risk factor for AI projects, and organizations must take proactive steps to ensure the quality, relevance, and timeliness of their data. By implementing best practices for AI-ready data management, organizations can avoid the pitfalls of poor data management and ensure the success of their AI projects. As the Gartner survey highlights, AI-ready data management is critical to realizing the full potential of AI and driving innovation and growth in today’s digital landscape.
News Source: