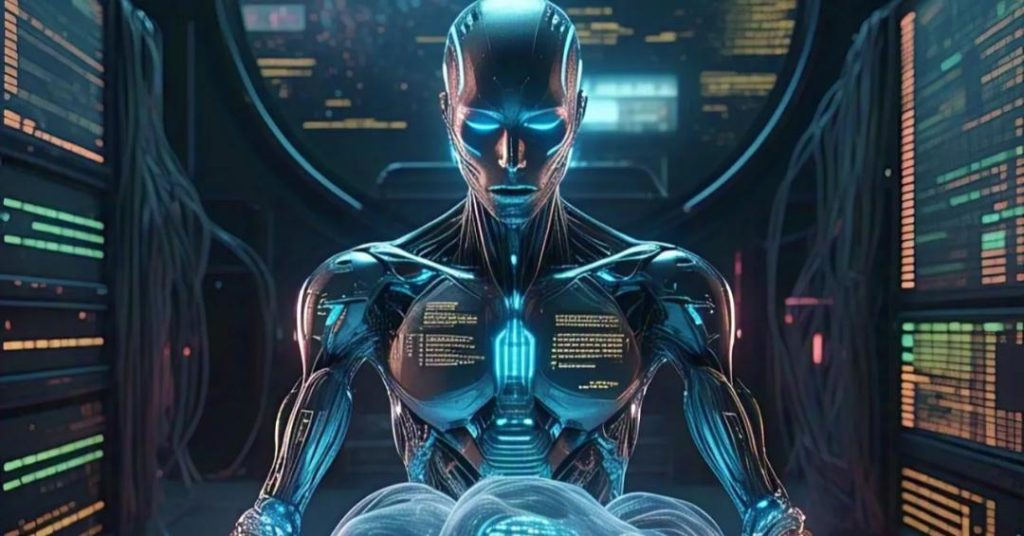
How Poor Data Management Can Put AI Projects at Risk
The advent of Artificial Intelligence (AI) has revolutionized the way businesses operate, making it possible to automate tasks, gain insights, and drive innovation. However, the success of AI projects largely depends on the quality of data used to train and test these models. Unfortunately, a recent survey by Gartner has revealed that 63% of organisations lack AI-ready data, putting their AI projects at risk of failure.
The survey, which polled 1,203 data management leaders in 2024, found that organisations failing to realise the differences between AI-ready requirements and traditional data management practices are endangering the success of their efforts. This is a stark reminder of the importance of effective data management in ensuring the success of AI projects.
So, what exactly is AI-ready data, and why is it so crucial to the success of AI projects? AI-ready data refers to the quality, quantity, and relevance of data that is required to train and test AI models. This data must be clean, accurate, and well-structured to enable AI algorithms to learn and make predictions or decisions accurately.
Poor data management practices can lead to a range of issues that hinder AI adoption, including:
- Data Quality Issues: Poor data quality can result in inaccurate or incomplete data, which can negatively impact the performance of AI models. This can lead to errors, biases, and inconsistencies, ultimately affecting the decision-making process.
- Inconsistent Data Formats: Inconsistent data formats can make it challenging to integrate data from different sources, leading to data silos and making it difficult to extract insights.
- Lack of Metadata Management: Metadata management is the process of collecting, managing, and maintaining data about data. Poor metadata management can lead to a lack of visibility into data quality, making it difficult to identify and address issues.
- Outdated Data Practices: Outdated data practices can hinder AI adoption by making it difficult to integrate new data sources and formats, and by failing to take advantage of new technologies and tools.
The consequences of poor data management can be severe, including:
- Failed AI Projects: Poor data management can lead to failed AI projects, resulting in wasted time, resources, and budgets.
- Limited Insights: Poor data quality and inconsistent data formats can limit the insights that can be gained from AI models, making it difficult to make informed decisions.
- Biased AI Models: Poor data management can lead to biased AI models, which can perpetuate existing biases and perpetuate inequality.
- Regulatory Non-Compliance: Poor data management can result in non-compliance with regulatory requirements, leading to fines and reputational damage.
So, what can organisations do to ensure the success of their AI projects? Here are some best practices for effective data management:
- Develop a Data Strategy: Develop a data strategy that outlines the organisation’s data management goals and objectives.
- Improve Data Quality: Implement data quality checks and validation rules to ensure that data is accurate, complete, and consistent.
- Implement Metadata Management: Implement metadata management tools and processes to collect, manage, and maintain data about data.
- Integrate New Data Sources: Integrate new data sources and formats to ensure that data is integrated and consistent.
- Invest in AI-Ready Data Tools: Invest in AI-ready data tools and technologies to ensure that data is ready for AI processing.
- Monitor and Govern Data: Monitor and govern data to ensure that it is used responsibly and in compliance with regulatory requirements.
In conclusion, poor data management can put AI projects at risk of failure. To ensure the success of AI projects, organisations must develop a data strategy, improve data quality, implement metadata management, integrate new data sources, invest in AI-ready data tools, and monitor and govern data. By following these best practices, organisations can ensure that their data is AI-ready and ready to support the success of their AI projects.