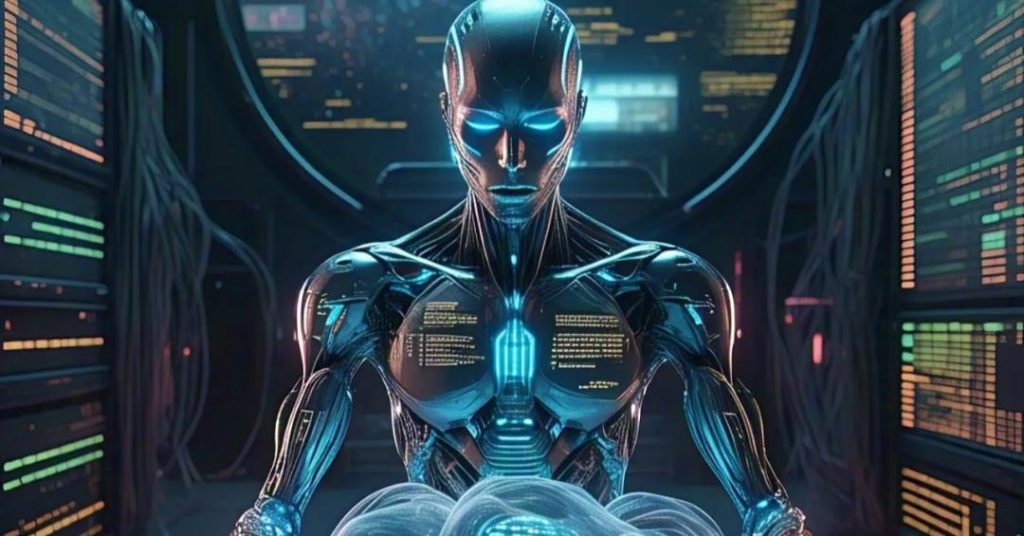
How poor data management can put AI projects at risk?
The adoption of Artificial Intelligence (AI) has become a crucial aspect of many business strategies, with its potential to revolutionize operations, improve efficiency, and drive growth. However, a recent survey by Gartner has revealed a stark reality: 63% of organisations lack AI-ready data, putting their AI projects at risk of failure. This alarming statistic highlights the critical importance of effective data management in ensuring the success of AI initiatives.
A survey of 1,203 data management leaders in 2024 found that organisations failing to recognize the differences between AI-ready requirements and traditional data management are endangering the success of their efforts. This suggests that many companies are not adequately preparing their data for AI adoption, which can have devastating consequences for their AI projects.
So, what is AI-ready data, and why is it so essential for AI projects? AI-ready data refers to high-quality, relevant, and well-structured data that is specifically designed to meet the unique requirements of AI algorithms. This type of data is essential for AI models to learn from, make accurate predictions, and drive meaningful insights.
However, many organisations are struggling to provide AI-ready data due to poor metadata management and outdated practices. Metadata management refers to the process of collecting, organizing, and maintaining data about data, including its structure, content, and relationships. Without effective metadata management, organisations are unable to understand the complexity and nuances of their data, leading to poor data quality and reduced effectiveness of AI models.
The consequences of poor data management for AI projects are severe. Without AI-ready data, AI models are unable to learn from and make accurate predictions about the data. This can lead to reduced accuracy, decreased confidence in AI outputs, and ultimately, the failure of AI projects.
Furthermore, poor data management can also lead to data silos, where data is fragmented and inaccessible across the organisation. This can result in duplicated efforts, wasted resources, and reduced collaboration between teams. In the context of AI projects, data silos can hinder the development of integrated AI models, making it challenging to gain a comprehensive understanding of complex business processes.
So, what can organisations do to avoid the pitfalls of poor data management and ensure the success of their AI projects?
- Understand AI-ready data requirements: Organisations must recognize the unique requirements of AI-ready data and develop strategies to meet these requirements. This involves understanding the complexity of AI algorithms, data formats, and data quality expectations.
- Implement effective metadata management: Implementing effective metadata management practices is essential for organisations to understand the complexity and nuances of their data. This involves collecting, organizing, and maintaining data about data, including its structure, content, and relationships.
- Integrate data from various sources: AI projects often require integrating data from various sources, including structured and unstructured data. Organisations must develop strategies to integrate this data, ensuring that it is consistent, accurate, and accessible.
- Develop data governance frameworks: Developing data governance frameworks is essential for organisations to ensure that data is managed consistently and efficiently. This involves establishing policies, procedures, and standards for data management, including data quality, security, and access.
- Monitor and evaluate data quality: Organisations must monitor and evaluate the quality of their data to ensure that it meets AI-ready requirements. This involves developing data quality metrics, tracking data quality over time, and taking corrective actions to improve data quality.
In conclusion, poor data management can put AI projects at risk, leading to reduced accuracy, decreased confidence in AI outputs, and ultimately, the failure of AI projects. Organisations must recognize the importance of AI-ready data and develop strategies to meet these requirements. By implementing effective metadata management, integrating data from various sources, developing data governance frameworks, and monitoring and evaluating data quality, organisations can ensure the success of their AI projects and unlock the full potential of AI.