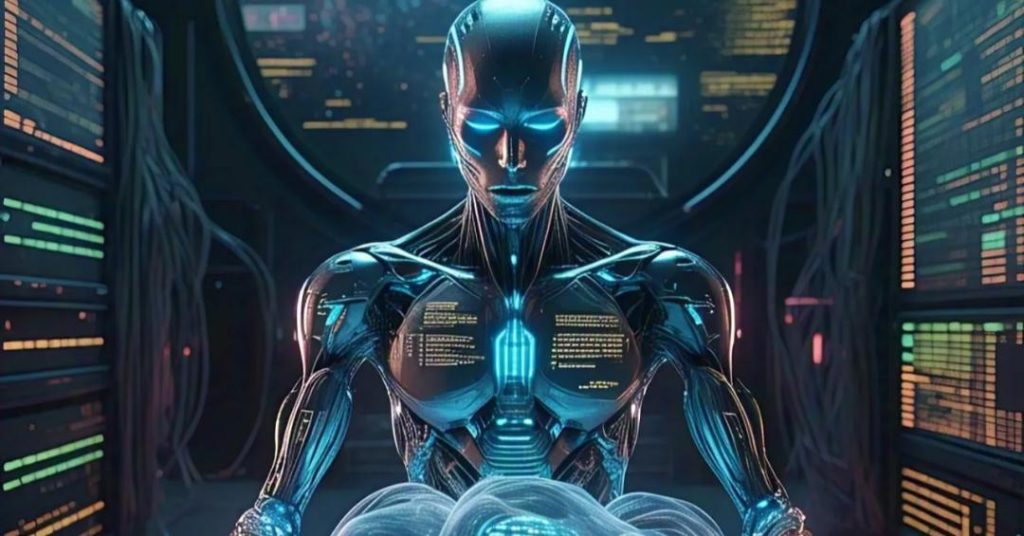
How Poor Data Management Can Put AI Projects at Risk?
The adoption of Artificial Intelligence (AI) has been gaining momentum across various industries, with many organizations investing heavily in AI projects to stay ahead of the competition. However, a recent survey by Gartner reveals that 63% of organizations lack AI-ready data, putting their AI projects at risk of failure. This staggering statistic highlights the crucial importance of effective data management in AI adoption.
The survey, which involved 1,203 data management leaders in 2024, found that organizations failing to realize the differences between AI-ready requirements and traditional data management practices are endangering the success of their AI efforts. This emphasizes the need for organizations to reassess their data management strategies and ensure that they are equipped to handle the unique demands of AI projects.
So, what is AI-ready data, and why is it essential for AI projects to succeed? AI-ready data refers to high-quality, well-organized, and easily accessible data that can be used to train AI models. This type of data is critical for AI projects as it enables them to learn from past experiences, make accurate predictions, and improve over time.
However, poor data management practices can hinder the creation of AI-ready data, leading to a range of issues, including:
- Data quality: Poor data quality can lead to AI models that are inaccurate, biased, or unreliable. This can result in incorrect decisions, wasted resources, and damaged reputations.
- Data scarcity: Inadequate data can hinder the training of AI models, making it difficult for them to learn and improve over time.
- Data silos: Data silos can prevent organizations from accessing the data they need to train AI models, leading to delays, inefficiencies, and increased costs.
- Lack of metadata management: Poor metadata management can make it difficult to understand the context and meaning of the data, leading to incorrect interpretations and poor decision-making.
To overcome these challenges, organizations must adopt effective data management practices that prioritize data quality, accessibility, and metadata management. This can be achieved by:
- Implementing data governance: Establishing a data governance framework can help organizations ensure that data is accurate, complete, and consistent across the organization.
- Improving data quality: Implementing data quality controls, such as data cleansing and normalization, can help organizations ensure that their data is accurate and reliable.
- Implementing metadata management: Implementing metadata management tools can help organizations understand the context and meaning of their data, making it easier to interpret and use.
- Breaking down data silos: Implementing data integration and data warehousing solutions can help organizations break down data silos and provide a single source of truth for their data.
- Investing in AI-specific data management tools: Investing in AI-specific data management tools, such as data labeling and annotation tools, can help organizations prepare their data for AI projects.
In conclusion, poor data management can put AI projects at risk, leading to inaccurate predictions, wasted resources, and damaged reputations. To overcome these challenges, organizations must adopt effective data management practices that prioritize data quality, accessibility, and metadata management. By implementing data governance, improving data quality, implementing metadata management, breaking down data silos, and investing in AI-specific data management tools, organizations can ensure that their AI projects are successful and deliver the expected returns.