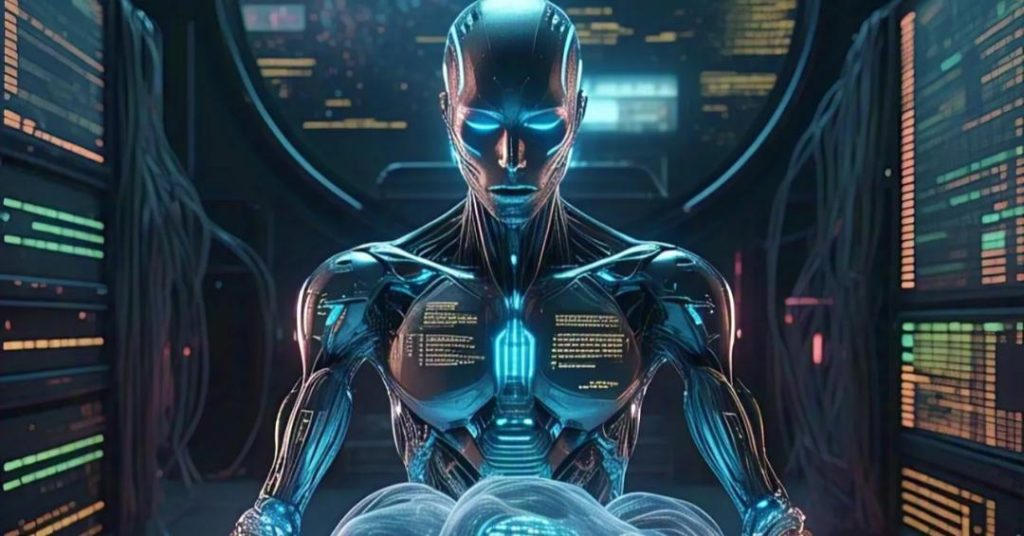
How Poor Data Management Can Put AI Projects at Risk
The rapid adoption of Artificial Intelligence (AI) has led to a surge in AI-related projects across various industries. However, a recent survey by Gartner revealed that 63% of organizations lack AI-ready data, putting the success of these projects at risk. The survey, which polled 1,203 data management leaders in 2024, highlights the importance of proper data management in ensuring the success of AI initiatives.
Poor metadata management and outdated practices are significant hindrances to AI adoption. Organizations failing to recognize the distinct requirements of AI-ready data and traditional data management are more likely to encounter challenges in their AI projects. In this blog post, we will delve into the consequences of poor data management on AI projects and explore strategies for overcoming these challenges.
The Consequences of Poor Data Management
Poor data management can have far-reaching consequences for AI projects. Some of the most common issues include:
- Data Quality Issues: Poor data management can lead to data inconsistencies, inaccuracies, and incompleteness. This can result in AI models producing incorrect or unreliable results, which can be detrimental to the success of the project.
- Inefficient Data Processing: Outdated practices and inadequate infrastructure can lead to slow data processing, which can hinder the development and training of AI models.
- Lack of Data Standardization: Poor data management can result in a lack of standardization, making it difficult to integrate data from different sources and create a unified view of the data.
- Inadequate Data Governance: Poor data governance can lead to data security breaches, unauthorized access, and uncontrolled data usage, which can put the organization at risk.
- Insufficient Data Analytics: Poor data management can limit the ability to perform advanced data analytics, which is essential for AI model development and training.
The Impact of Poor Data Management on AI Projects
The consequences of poor data management on AI projects can be severe. Some of the most common effects include:
- Project Delays: Poor data management can lead to project delays, as teams struggle to gather and process high-quality data.
- Increased Costs: Poor data management can result in increased costs, as teams need to spend more time and resources on data cleaning, processing, and governance.
- Reduced Accuracy: Poor data management can lead to reduced accuracy in AI model predictions, which can be detrimental to the success of the project.
- Limited Insights: Poor data management can limit the insights gained from AI models, making it difficult to make informed business decisions.
- Lost Opportunities: Poor data management can lead to lost opportunities, as organizations fail to leverage AI technology to drive business growth and innovation.
Strategies for Overcoming Poor Data Management
To overcome the challenges of poor data management, organizations can adopt the following strategies:
- Implement Data Quality Initiatives: Implement data quality initiatives, such as data cleaning, processing, and governance, to ensure high-quality data.
- Invest in Data Analytics: Invest in data analytics tools and techniques to gain insights from data and improve data-driven decision-making.
- Develop a Data Governance Strategy: Develop a data governance strategy to ensure data security, access control, and standardization.
- Invest in AI-Ready Data Infrastructure: Invest in AI-ready data infrastructure, such as cloud-based data storage and processing, to support the development and training of AI models.
- Foster a Data-Driven Culture: Foster a data-driven culture within the organization, encouraging the use of data to drive business decisions and innovation.
Conclusion
Poor data management can have severe consequences for AI projects, including project delays, increased costs, reduced accuracy, limited insights, and lost opportunities. To overcome these challenges, organizations must implement data quality initiatives, invest in data analytics, develop a data governance strategy, invest in AI-ready data infrastructure, and foster a data-driven culture. By adopting these strategies, organizations can ensure the success of their AI projects and unlock the full potential of AI technology.
News Source: