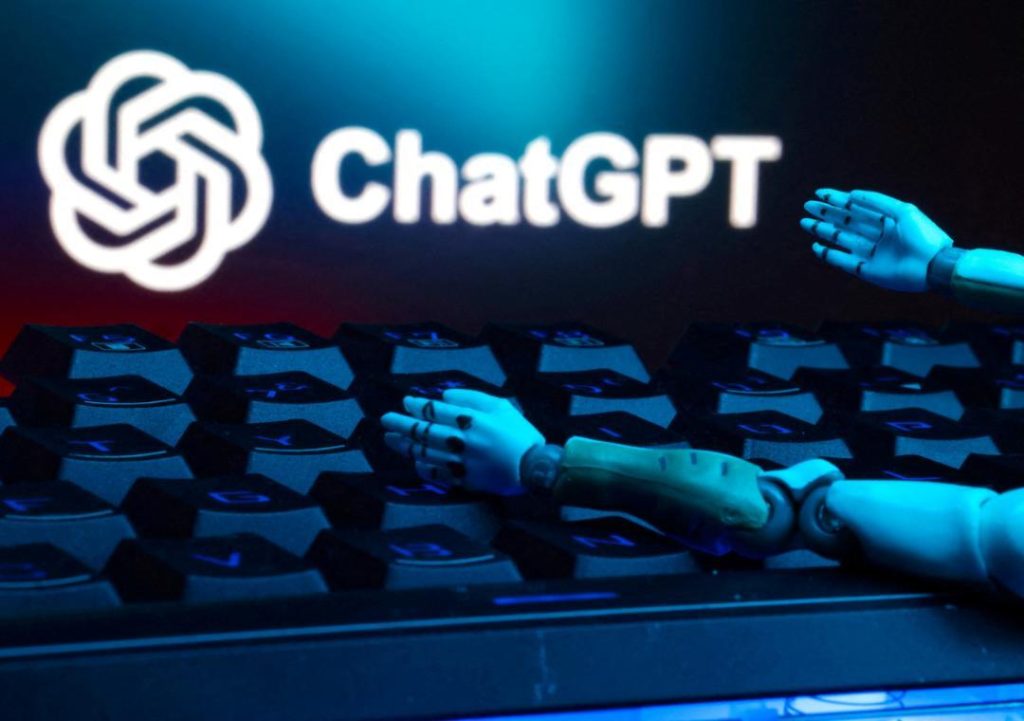
OpenAI’s o3 and o4-mini models hallucinate more than older models
Artificial intelligence (AI) has made tremendous progress in recent years, and one of the most significant advancements has been the development of language models. These models are capable of generating human-like text, and are being used in a wide range of applications, from chatbots to language translation. However, a recent report from OpenAI, a leading AI research organization, has highlighted a concerning issue with two of its latest models, o3 and o4-mini. According to the report, these models are more prone to hallucinating, or making up, answers than their older counterparts.
What is hallucination in AI models?
Before we dive into the details of OpenAI’s report, it’s essential to understand what hallucination means in the context of AI models. In simple terms, hallucination refers to the phenomenon where a language model generates text that is not based on any real-world information. This can include making up facts, names, or events, or even creating fictional stories. While this might seem harmless, hallucination can have serious consequences, particularly in applications where accuracy and reliability are crucial, such as language translation or generating medical reports.
OpenAI’s report highlights alarming trend
According to the report, OpenAI’s o3 and o4-mini models hallucinated 33% and 48% of the time, respectively. To put this into perspective, OpenAI’s older o3-mini model scored 14.8% in hallucination rating, while its o1 model scored 16%. These figures are alarming, and suggest that the newer models are more prone to generating inaccurate or fictional information.
The report highlights that the o3 and o4-mini models are more likely to hallucinate in certain situations, such as when they are asked to generate text based on incomplete information or when they are faced with ambiguous or unclear prompts. This is particularly concerning, as these situations are common in many real-world applications, such as customer service chatbots or language translation software.
What’s causing the hallucinations?
OpenAI researchers are still trying to understand the root cause of the hallucinations in their newer models. However, they have identified a few potential factors that may be contributing to this phenomenon. One possible explanation is the increased size and complexity of the newer models, which may be making it easier for them to generate fictional information. Another possibility is that the newer models are simply more creative or imaginative than their older counterparts, which can sometimes lead to hallucinations.
Implications for AI development
The findings of OpenAI’s report have significant implications for the development of AI models. First and foremost, it highlights the need for researchers to focus on developing more robust and accurate models that can avoid hallucinations. This may involve using different algorithms or architectures, or incorporating additional training data to help the models learn more accurate information.
Secondly, the report suggests that AI models may need to be designed with specific safeguards to prevent hallucinations. For example, models could be trained to identify and flag instances where they are generating fictional information, or to require additional confirmation before generating text.
Conclusion
OpenAI’s report on the hallucination rates of its o3 and o4-mini models is a sobering reminder of the challenges and limitations of AI development. While these models are incredibly powerful and have the potential to revolutionize many industries, we must also acknowledge the potential risks and pitfalls associated with their use.
As researchers and developers, it is essential that we prioritize the development of more accurate and reliable AI models, and that we take steps to prevent hallucinations and other forms of inaccurate information. By doing so, we can ensure that AI is used to benefit humanity, rather than to harm or mislead it.
Source: